Biological Valuation Map of Flanders: A Sentinel-2 Imagery Analysis
CoRR(2024)
摘要
In recent years, machine learning has become crucial in remote sensing
analysis, particularly in the domain of Land-use/Land-cover (LULC). The synergy
of machine learning and satellite imagery analysis has demonstrated significant
productivity in this field, as evidenced by several studies. A notable
challenge within this area is the semantic segmentation mapping of land usage
over extensive territories, where the accessibility of accurate land-use data
and the reliability of ground truth land-use labels pose significant
difficulties. For example, providing a detailed and accurate pixel-wise labeled
dataset of the Flanders region, a first-level administrative division of
Belgium, can be particularly insightful. Yet there is a notable lack of
regulated, formalized datasets and workflows for such studies in many regions
globally. This paper introduces a comprehensive approach to addressing these
gaps. We present a densely labeled ground truth map of Flanders paired with
Sentinel-2 satellite imagery. Our methodology includes a formalized dataset
division and sampling method, utilizing the topographic map layout
'Kaartbladversnijdingen,' and a detailed semantic segmentation model training
pipeline. Preliminary benchmarking results are also provided to demonstrate the
efficacy of our approach.
更多查看译文
AI 理解论文
溯源树
样例
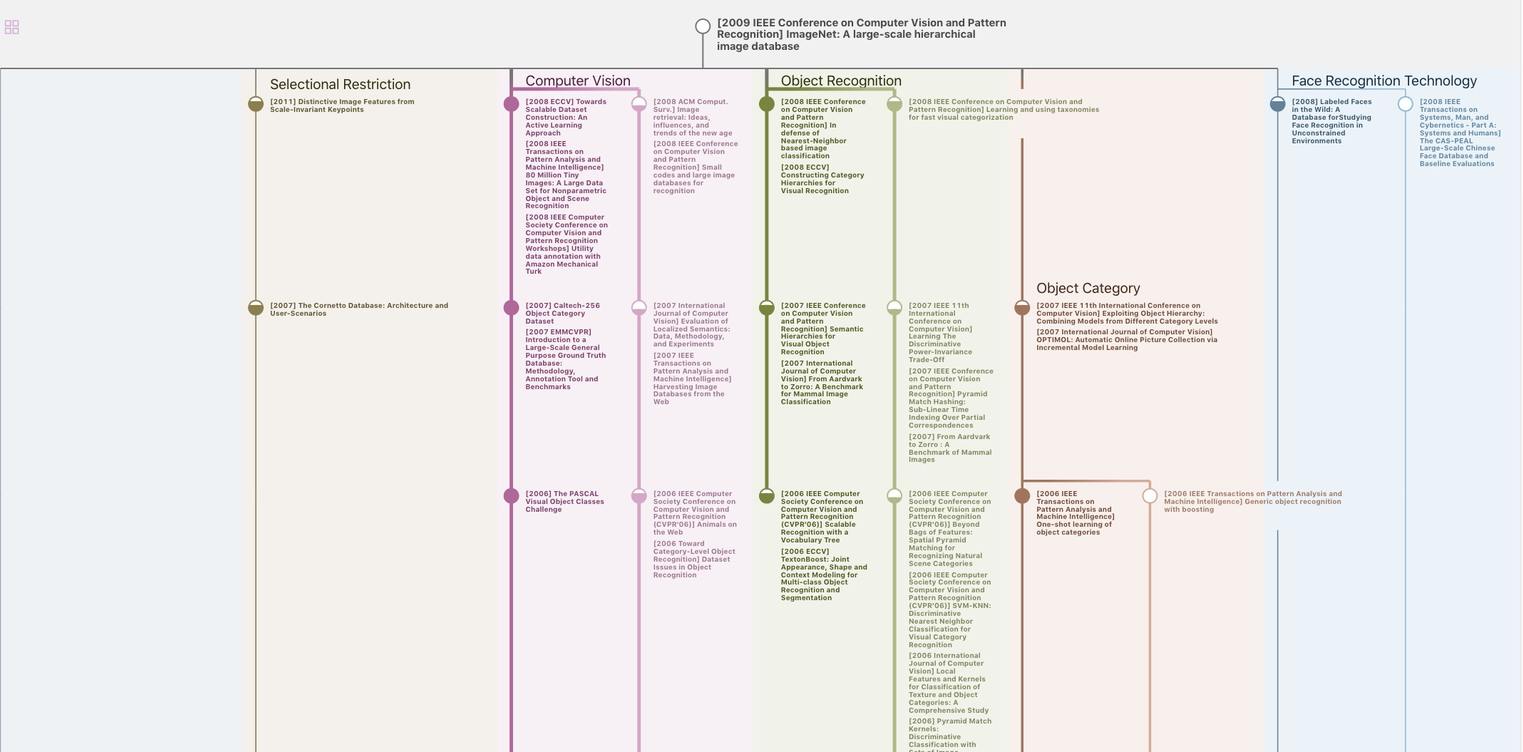
生成溯源树,研究论文发展脉络
Chat Paper
正在生成论文摘要