Efficient Online Crowdsourcing with Complex Annotations
AAAI 2024(2024)
摘要
Crowdsourcing platforms use various truth discovery algorithms to aggregate annotations from multiple labelers. In an online setting, however, the main challenge is to decide whether to ask for more annotations for each item to efficiently trade off cost (i.e., the number of annotations) for quality of the aggregated annotations. In this paper, we propose a novel approach for general complex annotation (such as bounding boxes and taxonomy paths), that works in an online crowdsourcing setting. We prove that the expected average similarity of a labeler is linear in their accuracy conditional on the reported label. This enables us to infer reported label accuracy in a broad range of scenarios. We conduct extensive evaluations on real-world crowdsourcing data from Meta and show the effectiveness of our proposed online algorithms in improving the cost-quality trade-off.
更多查看译文
关键词
HAI: Crowd Sourcing and Human Computation
AI 理解论文
溯源树
样例
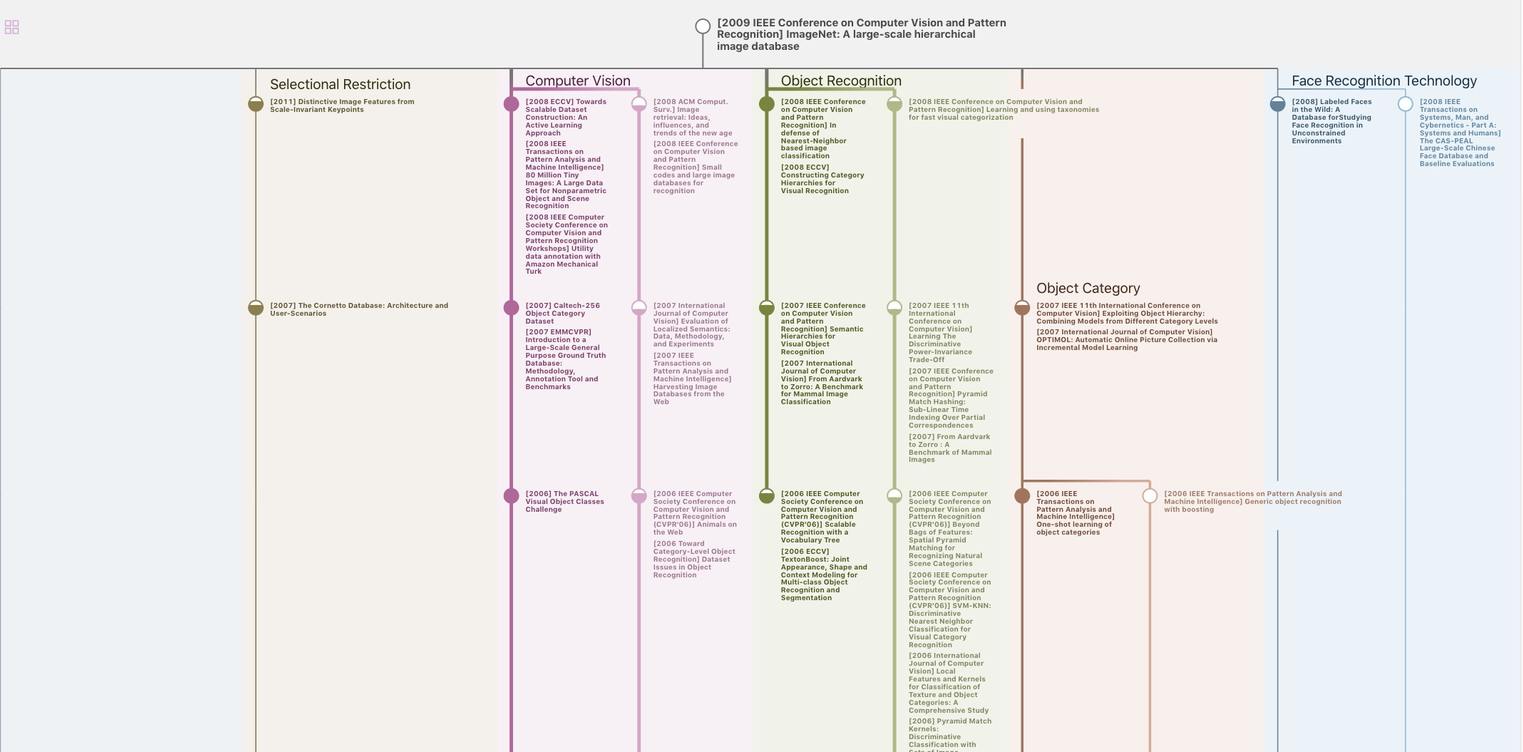
生成溯源树,研究论文发展脉络
Chat Paper
正在生成论文摘要