ReTaSA: A Nonparametric Functional Estimation Approach for Addressing Continuous Target Shift
CoRR(2024)
摘要
The presence of distribution shifts poses a significant challenge for
deploying modern machine learning models in real-world applications. This work
focuses on the target shift problem in a regression setting (Zhang et al.,
2013; Nguyen et al., 2016). More specifically, the target variable y (also
known as the response variable), which is continuous, has different marginal
distributions in the training source and testing domain, while the conditional
distribution of features x given y remains the same. While most literature
focuses on classification tasks with finite target space, the regression
problem has an infinite dimensional target space, which makes many of the
existing methods inapplicable. In this work, we show that the continuous target
shift problem can be addressed by estimating the importance weight function
from an ill-posed integral equation. We propose a nonparametric regularized
approach named ReTaSA to solve the ill-posed integral equation and provide
theoretical justification for the estimated importance weight function. The
effectiveness of the proposed method has been demonstrated with extensive
numerical studies on synthetic and real-world datasets.
更多查看译文
关键词
label shift,target shift,distributional shift,domain adaptation,transfer learning,importance weight
AI 理解论文
溯源树
样例
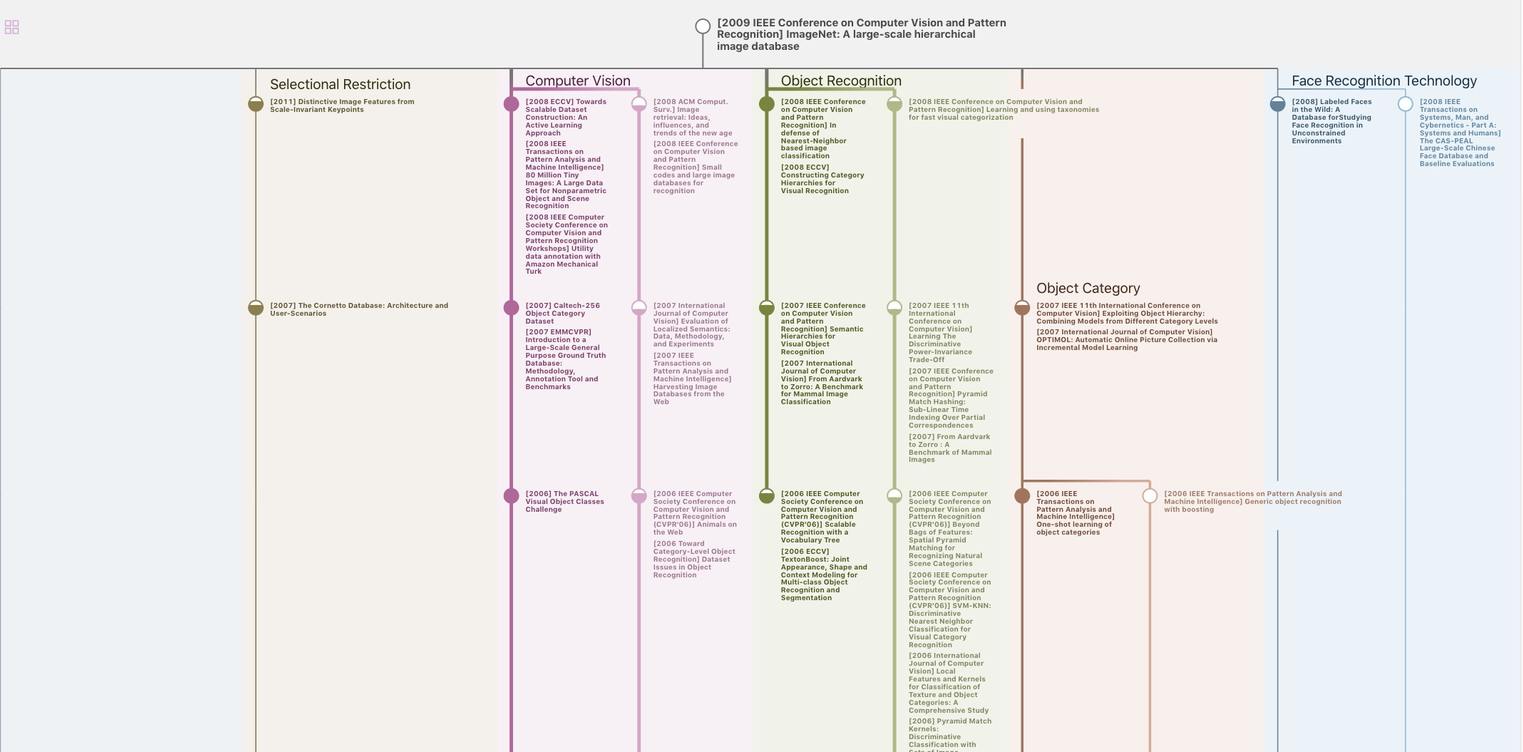
生成溯源树,研究论文发展脉络
Chat Paper
正在生成论文摘要