Predicting the DNA binding specificity of mutated transcription factors using family-level biophysically interpretable machine learning.
bioRxiv : the preprint server for biology(2024)
摘要
Sequence-specific interactions of transcription factors (TFs) with genomic DNA underlie many cellular processes. High-throughput in vitro binding assays coupled with computational analysis have made it possible to accurately define such sequence recognition in a biophysically interpretable yet mechanism-agonistic way for individual TFs. The fact that such sequence-to-affinity models are now available for hundreds of TFs provides new avenues for predicting how the DNA binding specificity of a TF changes when its protein sequence is mutated. To this end, we developed an analytical framework based on a tetrahedron embedding that can be applied at the level of a given structural TF family. Using bHLH as a test case, we demonstrate that we can systematically map dependencies between the protein sequence of a TF and base preference within the DNA binding site. We also develop a regression approach to predict the quantitative energetic impact of mutations in the DNA binding domain of a TF on its DNA binding specificity, and perform SELEX-seq assays on mutated TFs to experimentally validate our results. Our results point to the feasibility of predicting the functional impact of disease mutations and allelic variation in the cell-wide TF repertoire by leveraging high-quality functional information across sets of homologous wild-type proteins.
更多查看译文
AI 理解论文
溯源树
样例
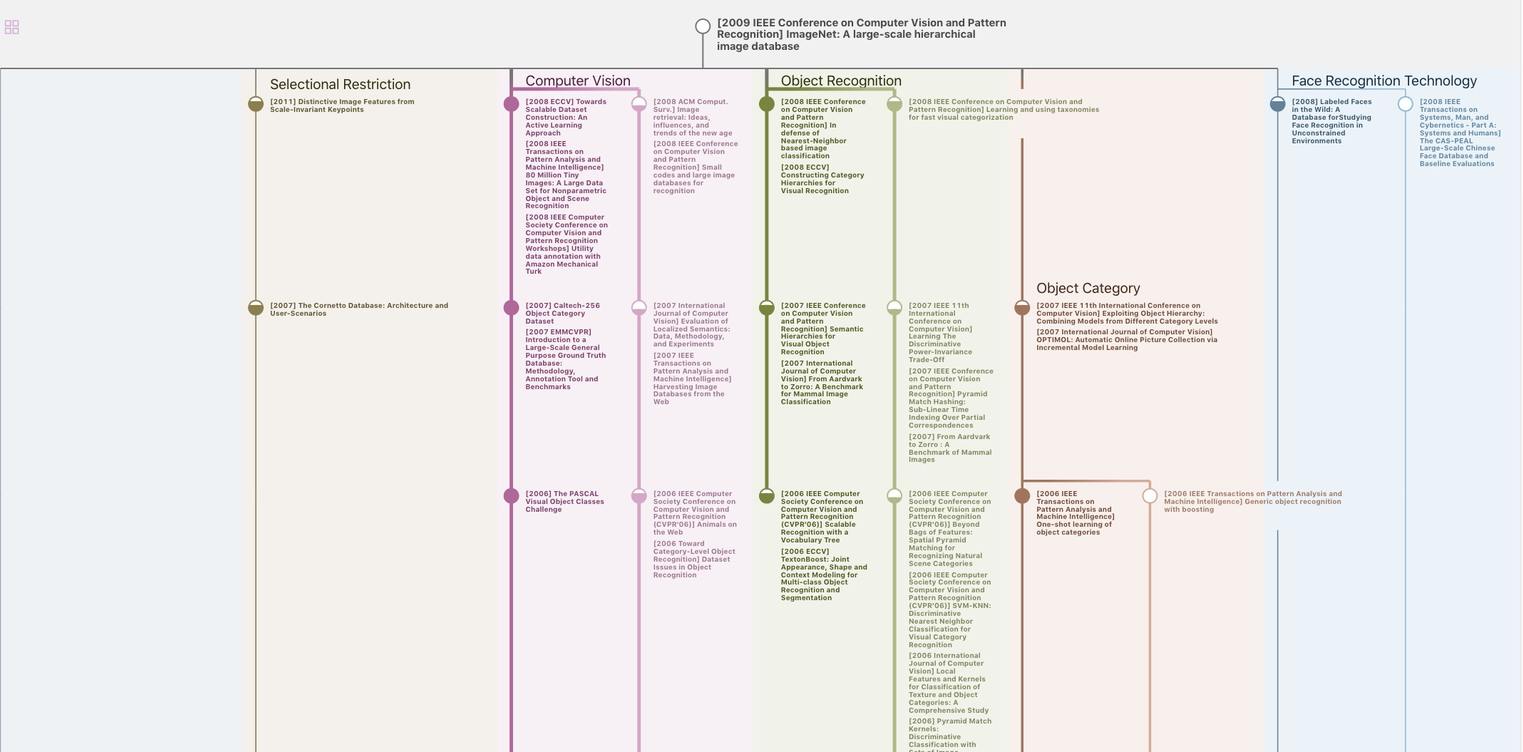
生成溯源树,研究论文发展脉络
Chat Paper
正在生成论文摘要