Ensemble Heuristic-Metaheuristic Feature Fusion Learning for Heart Disease Diagnosis Using Tabular Data
ALGORITHMS(2024)
摘要
Heart disease is a global health concern of paramount importance, causing a significant number of fatalities and disabilities. Precise and timely diagnosis of heart disease is pivotal in preventing adverse outcomes and improving patient well-being, thereby creating a growing demand for intelligent approaches to predict heart disease effectively. This paper introduces an ensemble heuristic-metaheuristic feature fusion learning (EHMFFL) algorithm for heart disease diagnosis using tabular data. Within the EHMFFL algorithm, a diverse ensemble learning model is crafted, featuring different feature subsets for each heterogeneous base learner, including support vector machine, K-nearest neighbors, logistic regression, random forest, naive bayes, decision tree, and XGBoost techniques. The primary objective is to identify the most pertinent features for each base learner, leveraging a combined heuristic-metaheuristic approach that integrates the heuristic knowledge of the Pearson correlation coefficient with the metaheuristic-driven grey wolf optimizer. The second objective is to aggregate the decision outcomes of the various base learners through ensemble learning. The performance of the EHMFFL algorithm is rigorously assessed using the Cleveland and Statlog datasets, yielding remarkable results with an accuracy of 91.8% and 88.9%, respectively, surpassing state-of-the-art techniques in heart disease diagnosis. These findings underscore the potential of the EHMFFL algorithm in enhancing diagnostic accuracy for heart disease and providing valuable support to clinicians in making more informed decisions regarding patient care.
更多查看译文
关键词
heart disease diagnosis,ensemble learning,feature selection,heuristics,metaheuristics,Pearson correlation coefficient (PCC),grey wolf optimizer (GWO)
AI 理解论文
溯源树
样例
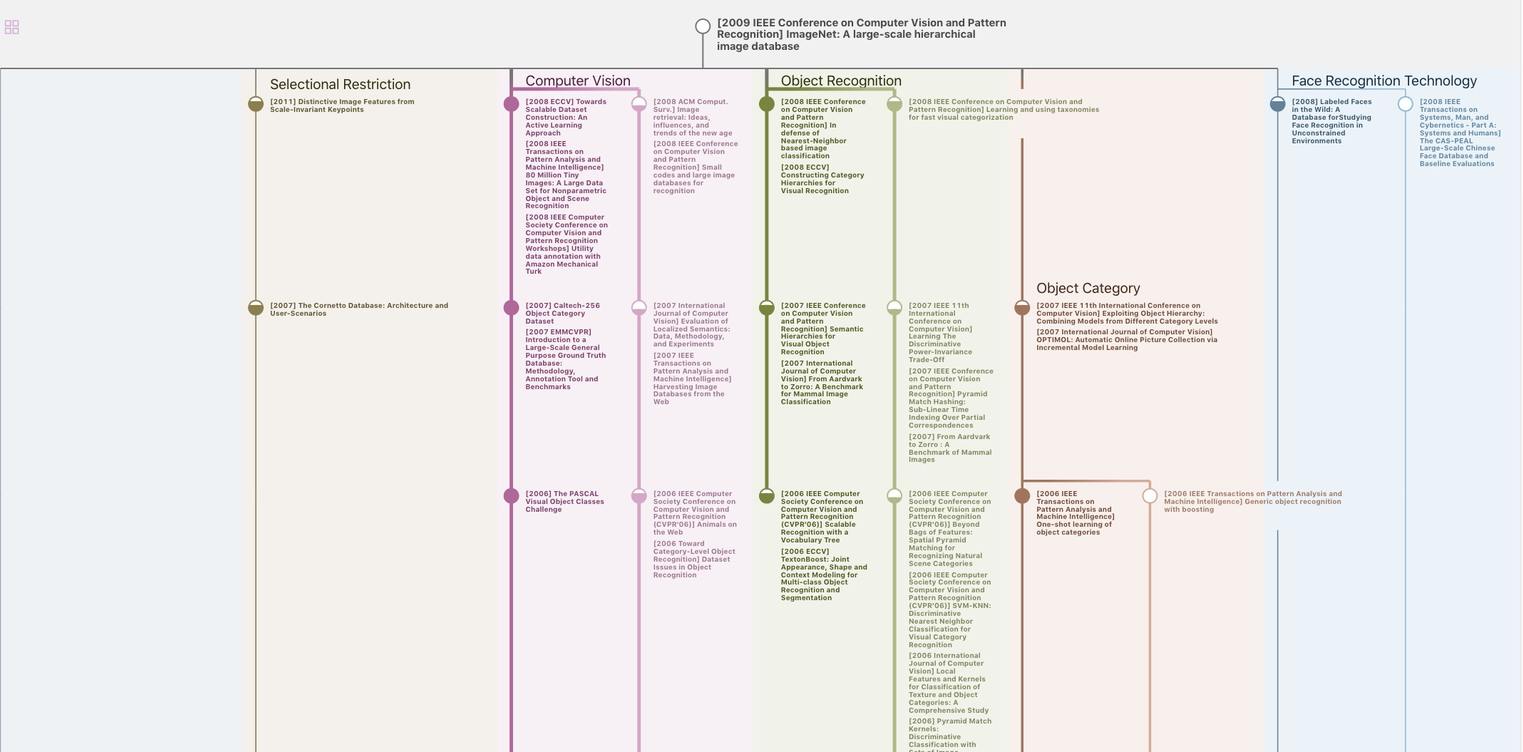
生成溯源树,研究论文发展脉络
Chat Paper
正在生成论文摘要