Evolutionary Optimization with Simplified Helper Task for High-dimensional Expensive Multiobjective ProblemsJust Accepted
ACM Transactions on Evolutionary Learning and Optimization(2023)
摘要
In recent years, surrogate-assisted evolutionary algorithms (SAEAs) have been sufficiently studied for tackling computationally expensive multiobjective optimization problems (EMOPs), as they can quickly estimate the qualities of solutions by using surrogate models to substitute for expensive evaluations. However, most existing SAEAs only show promising performance for solving EMOPs with no more than 10 dimensions, and become less efficient for tackling EMOPs with higher dimensionality. Thus, this article proposes a new SAEA with a simplified helper task for tackling high-dimensional EMOPs. In each generation, one simplified task will be generated artificially by using random dimension reduction on the target task (i.e., the target EMOPs). Then, two surrogate models are trained for the helper task and the target task, respectively. Based on the trained surrogate models, evolutionary multitasking optimization is run to solve these two tasks, so that the experiences of solving the helper task can be transferred to speed up the convergence of tackling the target task. Moreover, an effective model management strategy is designed to select new promising samples for training the surrogate models. When compared to five competitive SAEAs on four well-known benchmark suites, the experiments validate the advantages of the proposed algorithm on most test cases.
更多查看译文
关键词
Surrogate-assisted evolutionary algorithm,expensive multiobjective optimization problem,evolutionary multitasking,model management
AI 理解论文
溯源树
样例
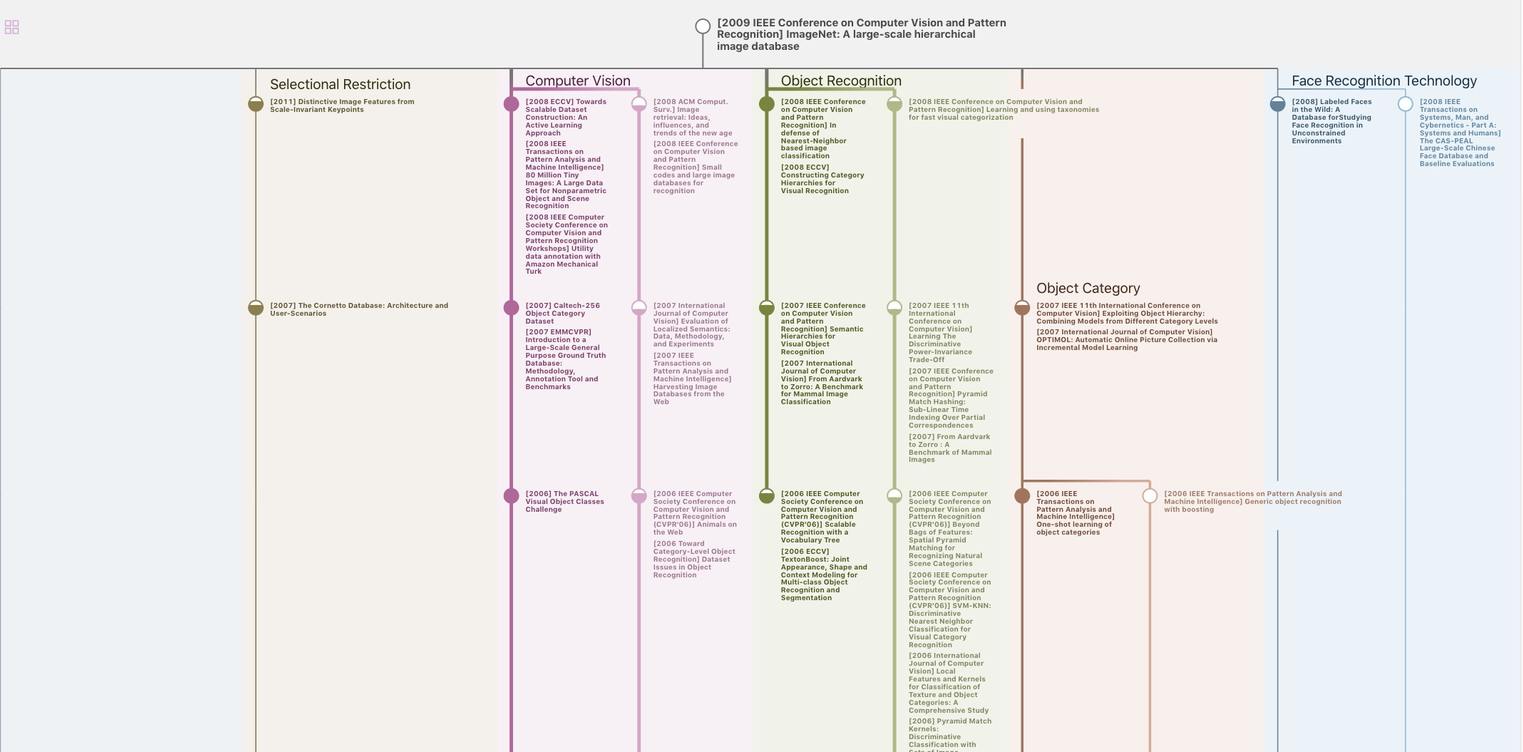
生成溯源树,研究论文发展脉络
Chat Paper
正在生成论文摘要