diGRASS: Directed Graph Spectral Sparsification via Spectrum-Preserving SymmetrizationJust Accepted
ACM Transactions on Knowledge Discovery from Data(2022)
摘要
Recent spectral graph sparsification research aims to construct ultra-sparse subgraphs for preserving the original graph spectral (structural) properties, such as the first few Laplacian eigenvalues and eigenvectors, which has led to the development of a variety of nearly-linear time numerical and graph algorithms. However, there is very limited progress for spectral sparsification of directed graphs. In this work, we prove the existence of nearly-linear-sized spectral sparsifiers for directed graphs under certain conditions. Furthermore, we introduce a practically-efficient spectral algorithm (diGRASS) for sparsifying real-world, large-scale directed graphs leveraging spectral matrix perturbation analysis. The proposed method has been evaluated using a variety of directed graphs obtained from real-world applications, showing promising results for solving directed graph Laplacians, spectral partitioning of directed graphs, and approximately computing (personalized) PageRank vectors.
更多查看译文
关键词
spectral graph theory,spectral graph sparsification,directed graphs,Laplacian solver,PageRank.
AI 理解论文
溯源树
样例
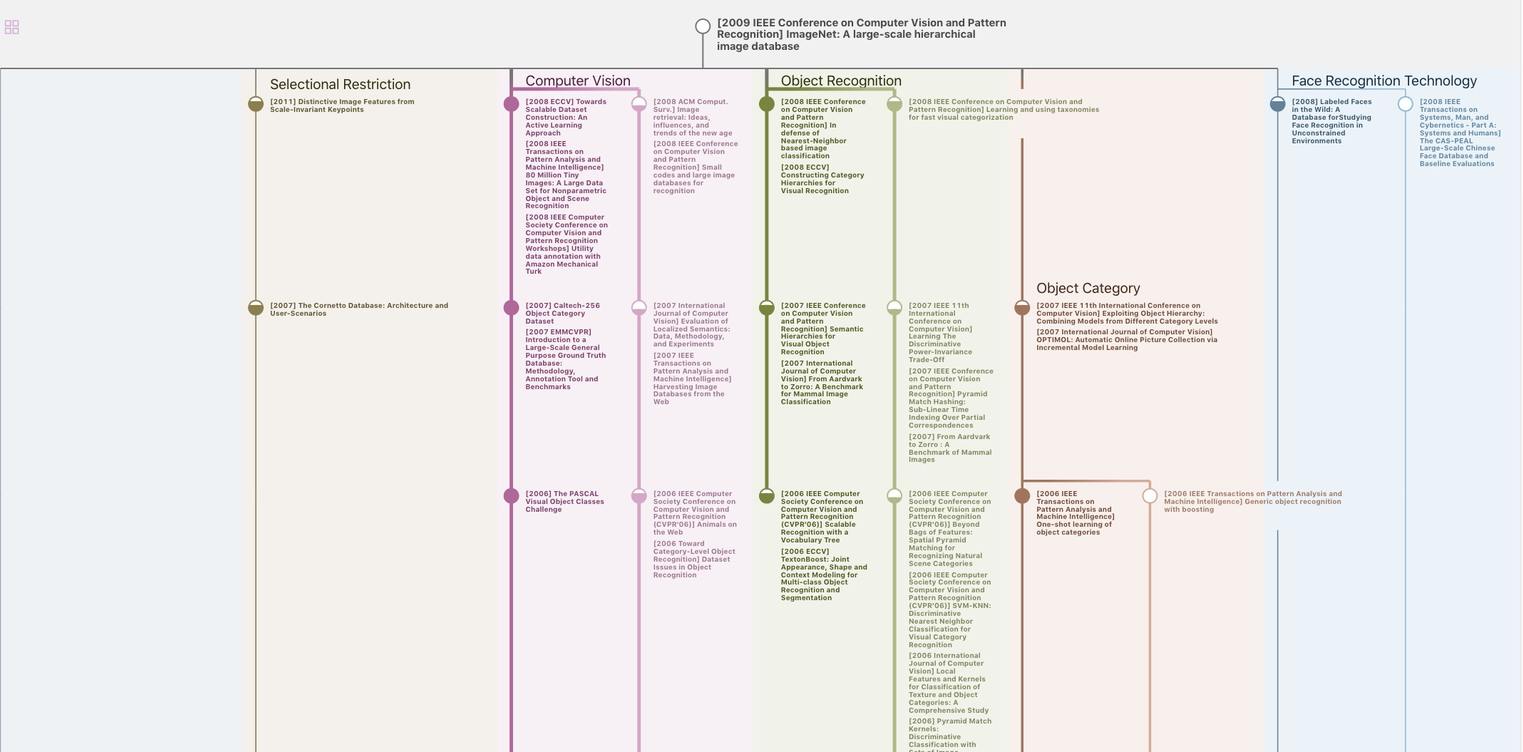
生成溯源树,研究论文发展脉络
Chat Paper
正在生成论文摘要