SpellGCN: Incorporating Phonological and Visual Similarities into Language Models for Chinese Spelling Check.
58TH ANNUAL MEETING OF THE ASSOCIATION FOR COMPUTATIONAL LINGUISTICS (ACL 2020)(2020)
摘要
Chinese Spelling Check (CSC) is a task to detect and correct spelling errors in Chinese natural language. Existing methods have made attempts to incorporate the similarity knowledge between Chinese characters. However, they take the similarity knowledge as either an external input resource or just heuristic rules. This paper proposes to incorporate phonological and visual similarity knowledge into language models for CSC via a specialized graph convolutional network (SpellGCN). The model builds a graph over the characters, and SpellGCN is learned to map this graph into a set of inter-dependent character classifiers. These classifiers are applied to the representations extracted by another network, such as BERT, enabling the whole network to be end-to-end trainable. Experiments 1 are conducted on three human-annotated datasets. Our method achieves superior performance against previous models by a large margin.
更多查看译文
关键词
chinese spelling check,visual similarities,language models
AI 理解论文
溯源树
样例
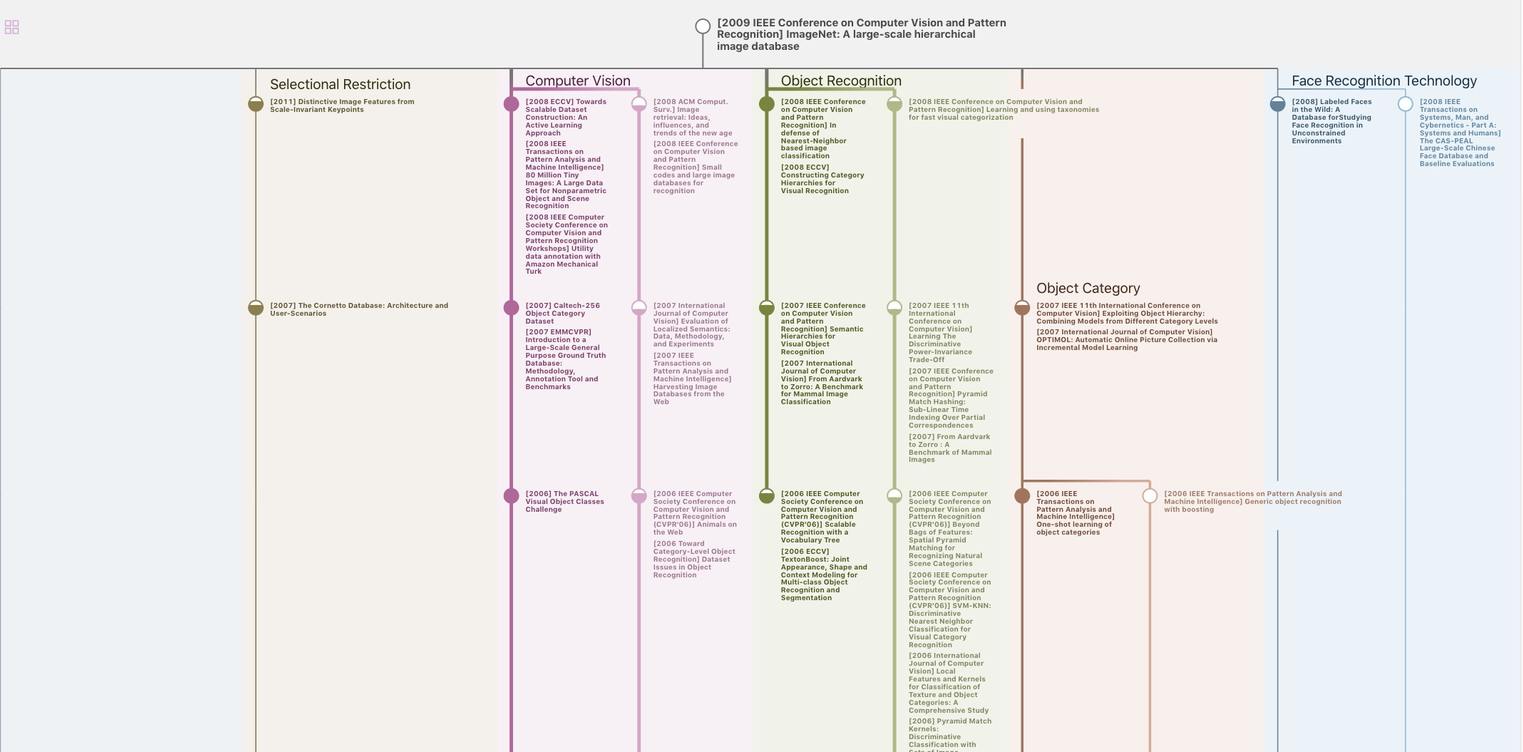
生成溯源树,研究论文发展脉络
Chat Paper
正在生成论文摘要