A deep learning framework for historical manuscripts writer identification using data-driven features
Multimedia Tools and Applications(2024)
摘要
Writer identification form historical manuscripts presents a challenging problem with significant implications for understanding the authorship of ancient texts. In this paper, we propose a novel deep learning framework tailored for the task of historical manuscripts writer identification. Our approach leverages data-driven features, harnessing the power of neural networks to extract and learn discriminative patterns from handwritten historical documents. The key innovation of our framework lies in its ability to automatically discover and utilize relevant features from data to profile the writer, eliminating the need for manual feature engineering. Our methodology encompasses three well-defined steps: initially, manuscript preprocessing involves image denoising using advanced techniques such as non-local means and total-variation, followed by binarization using a Canny-edge detector. In the subsequent phase, we employ Harris corner detector for automatic key-point detection and clustering, allowing us to identify the regions of interest within the documents. Lastly, the features extracted from these regions are subjected to classification through transfer learning, utilizing a deep learning-based model specifically trained on the extracted patches. To achieve the final document-level identification, we enhance the system accuracy by implementing a majority vote scheme, where the aggregated decisions from multiple patches contribute to the ultimate classification outcome. We validate our approach on “ICDAR 2017” dataset, spanning different periods and writing styles of historical manuscripts. Experimental results demonstrate the superior performance of our method in accurately identifying the authors of historical documents, surpassing existing techniques. Moreover, our framework exhibits robustness in scenarios where limited training data is available. This work not only contributes to the field of historical manuscripts analysis but also highlights the potential of deep learning in solving intricate problems in the realm of document analysis and authorship attribution. Our framework offers a promising avenue for scholars and historians to gain deeper insights into the authors of historical texts, opening new doors for historical research and preservation.
更多查看译文
关键词
Writer identification,Historical manuscripts,Feature extraction,Key-points detection,Clustering,Deep learning
AI 理解论文
溯源树
样例
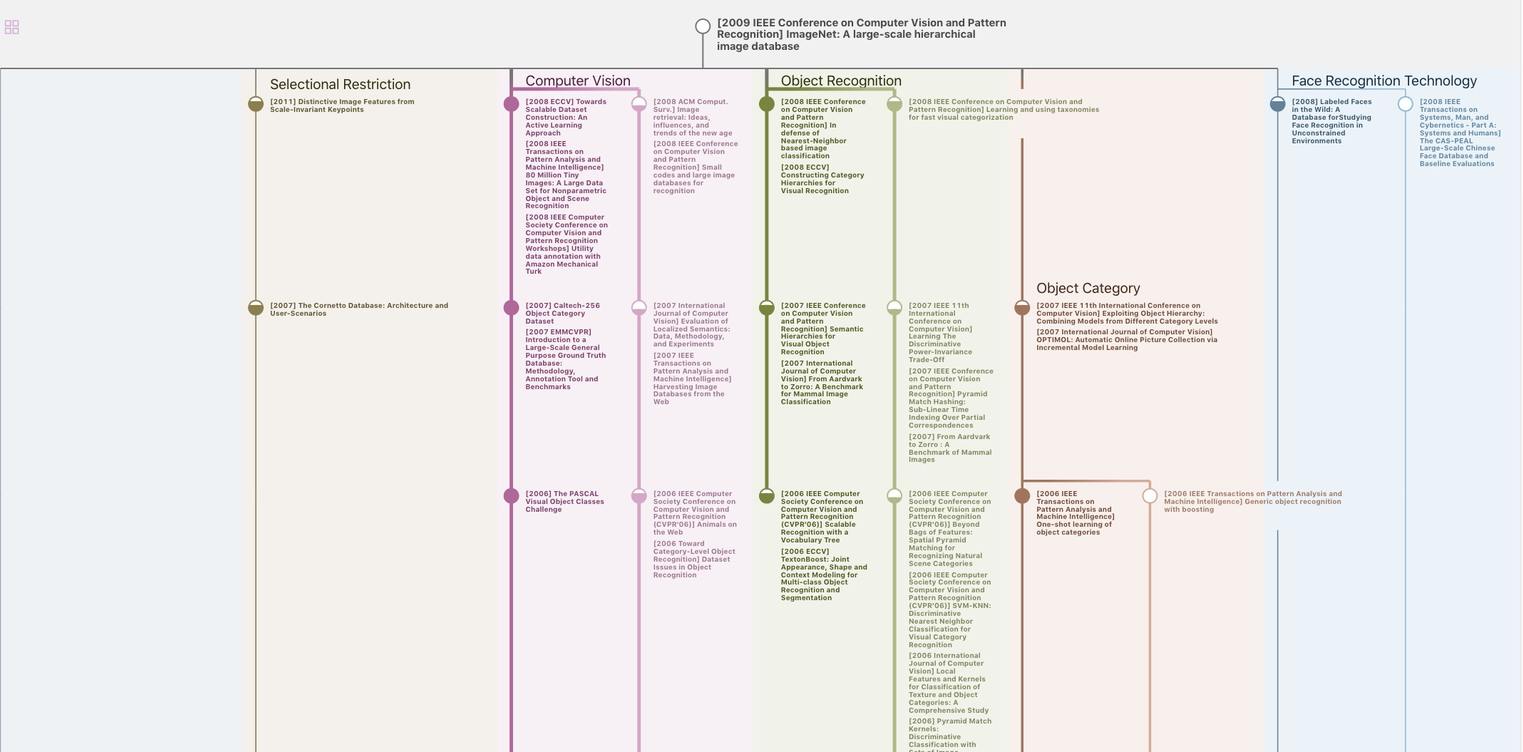
生成溯源树,研究论文发展脉络
Chat Paper
正在生成论文摘要