Soft computing-based reliability analysis of simply supported beam: a comparative study of hybrid ANN models
Asian Journal of Civil Engineering(2024)
摘要
The article presents a state-of-the-art of machine learning (ML)-based reliability analysis for predicting the safety performance of a simply supported beam subjected to a uniformly distributed load. For this purpose, state-of-the-art hybrid artificial neural network (ANN) models are used using four optimization algorithms: ABC (Artificial Bee Colony), ACO (Ant Colony Optimization), ALO (Ant Lion Optimization), and TLBO (Teaching Learning Based Optimization). For the study, 800 datasets of a simply supported beam were generated, where load intensity (w) and modulus of elasticity (E) are the input parameters and deflection (δ) is the predictor parameter. The authenticity of the dataset is established using descriptive statistics, a histogram, and the correlation matrix. The training and testing performances of the model are analysed using various well-established performance parameters, rank analysis, and AIC criteria. The comparative analysis of the performances is provided using rank analysis, while the visual, reader-friendly analysis is provided using Taylor diagrams. Reliability indices (RI) and probability of failure (POF) are calculated for the actual values of deflection using FORM and compared with ML modelling-based reliability indices. The values of RI and POF for ANN-ALO, ANN-ACO and ANN-TLBO are closer to the actual, while ANN-ABC yields over-prediction. Therefore, it is concluded that the hybrid ANNs simulated in the study are robust and reliable tools for reliability-based design of a simply supported beam, however, the performance of ANN-ABC is less satisfactory compared to others.
更多查看译文
关键词
Reliability analysis,Machine learning,Hybrid ANN,Simply supported beam
AI 理解论文
溯源树
样例
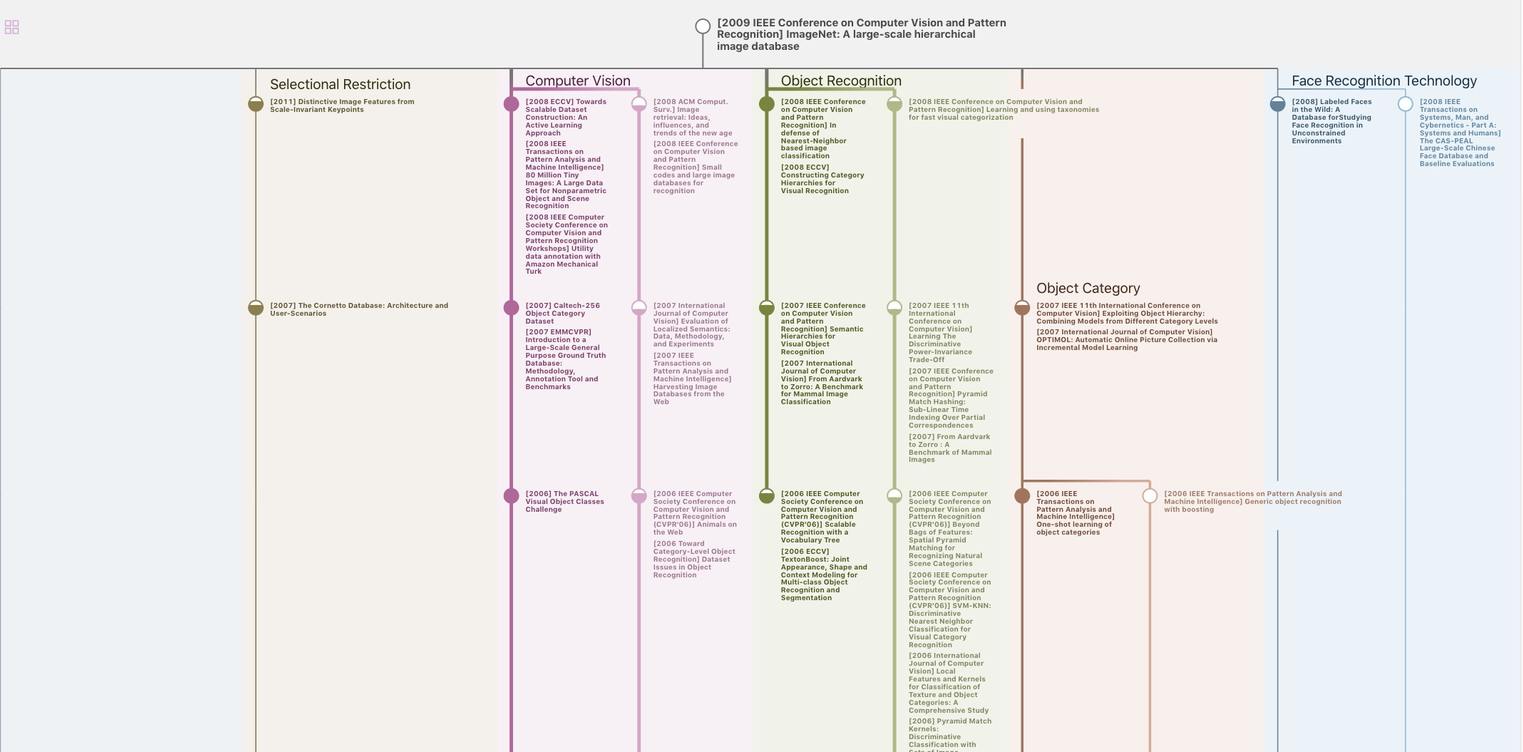
生成溯源树,研究论文发展脉络
Chat Paper
正在生成论文摘要