DDoS attack traffic classification in SDN using deep learning
Personal and Ubiquitous Computing(2024)
摘要
Software-defined networking will be a critical component of the networking domain as it transitions from a standard networking design to an automation network. To meet the needs of the current scenario, this architecture redesign becomes mandatory. Besides, machine learning (ML) and deep learning (DL) techniques provide a significant solution in network attack detection, traffic classification, etc. The DDoS attack is still wreaking havoc. Previous work for DDoS attack detection in SDN has not yielded significant results, so the author has used the most recent deep learning technique to detect the attacks. In this paper, we aim to classify the network traffic into normal and malicious classes based on features in the available dataset by using various deep learning techniques. TCP, UDP, and ICMP traffic are considered normal; however, malicious traffic includes TCP Syn Attack, UDP Flood, and ICMP Flood, all of which are DDoS attack traffic. The major contribution of this paper is the identification of novel features for DDoS attack detection. Novel features are logged into the CSV file to create the dataset, and machine learning algorithms are trained on the created SDN dataset. Various work which has already been done for DDoS attack detection either used a non-SDN dataset or the research data is not made public. A novel hybrid machine learning model is utilized to perform the classification. The dataset used by the ML/DL algorithms is a collection of public datasets on DDoS attacks as well as an experimental DDoS dataset generated by us and publicly available on the Mendeley Data repository. A Python application performs the classification of traffic into one of the classes. From the various classifiers used, the accuracy score of 99.75% is achieved with Stacked Auto-Encoder Multi-layer Perceptron (SAE-MLP). To measure the effectiveness of the SDN-DDoS dataset, the other publicly available datasets are also evaluated against the same deep learning algorithms, and traffic classification accuracy is found to be significantly higher with the SDN-DDoS dataset. The attack detection time of 216.39 s also serve as experimental evidence.
更多查看译文
关键词
Software-defined networking (SDN),Mininet emulator,DDoS attack,Dataset,Deep learning
AI 理解论文
溯源树
样例
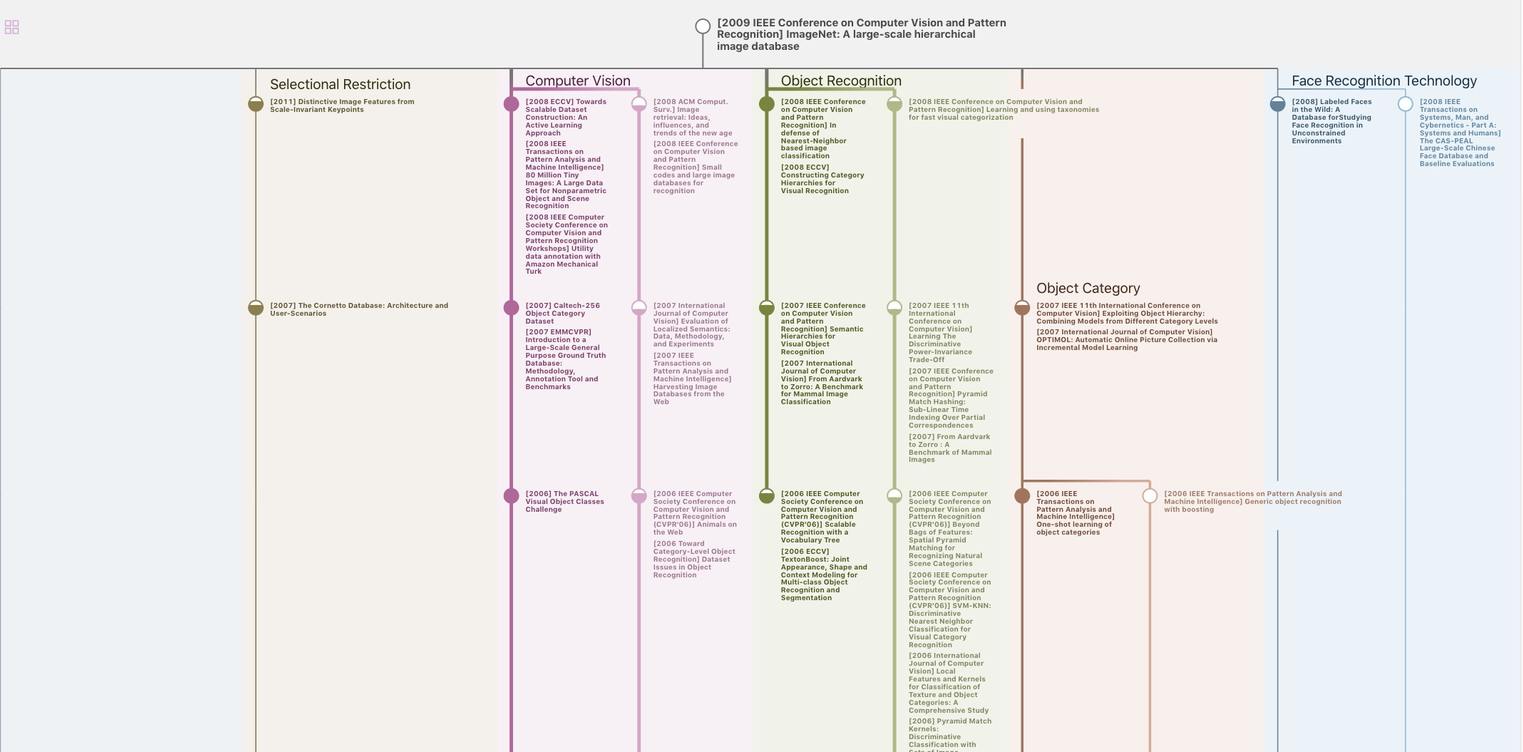
生成溯源树,研究论文发展脉络
Chat Paper
正在生成论文摘要