Estimating hydroponic lettuce phenotypic parameters for efficient resource allocation
COMPUTERS AND ELECTRONICS IN AGRICULTURE(2024)
摘要
Crop growth monitoring is pivotal in optimizing management strategies and maximizing greenhouse production. Traditionally, crop monitoring is carried out manually, which makes it unfeasible to collect data daily to get actionable insights for high yield. This study presents an innovative, non-destructive approach to predict lettuce growth parameters, including leaf area, fresh weight, dry weight, plant diameter, and plant height. The proposed methodology capitalizes on the capabilities of a semantic segmentation model, specifically, a lightweight DeepLabv3 + network that integrates MobileNetv2. This model showcases exceptional performance with a mean IoU score of 0.9979, accuracy of 0.9985, and a segmentation speed of 0.075fps. Furthermore, the study assesses the performance of the deep learning regression model in predicting lettuce phenotypic parameters, achieving R-2 values of 0.968, 0.953, 0.943, 0.906, and 0.965 for fresh weight, leaf area, dry weight, plant diameter, and plant height, respectively. To underscore the model's robustness, it is subjected to validation under various treatment conditions, encompassing variations in nutrients and temperature. Our findings revealed that the treatment involving high nutrient temperature and medium N contents (Temp: 30 degrees C, Nitrogen: 150 ppm) yielded the highest fresh and dry weights. These validations substantiate the efficacy of the predictive model for hydroponic lettuce, and this innovative approach holds promise for data aggregation and predictive analytics to assist growers in decision-making for resource optimization.
更多查看译文
关键词
Deep learning,Depth image,Multimodal,Lettuce phenotyping,Regression,Resource allocation
AI 理解论文
溯源树
样例
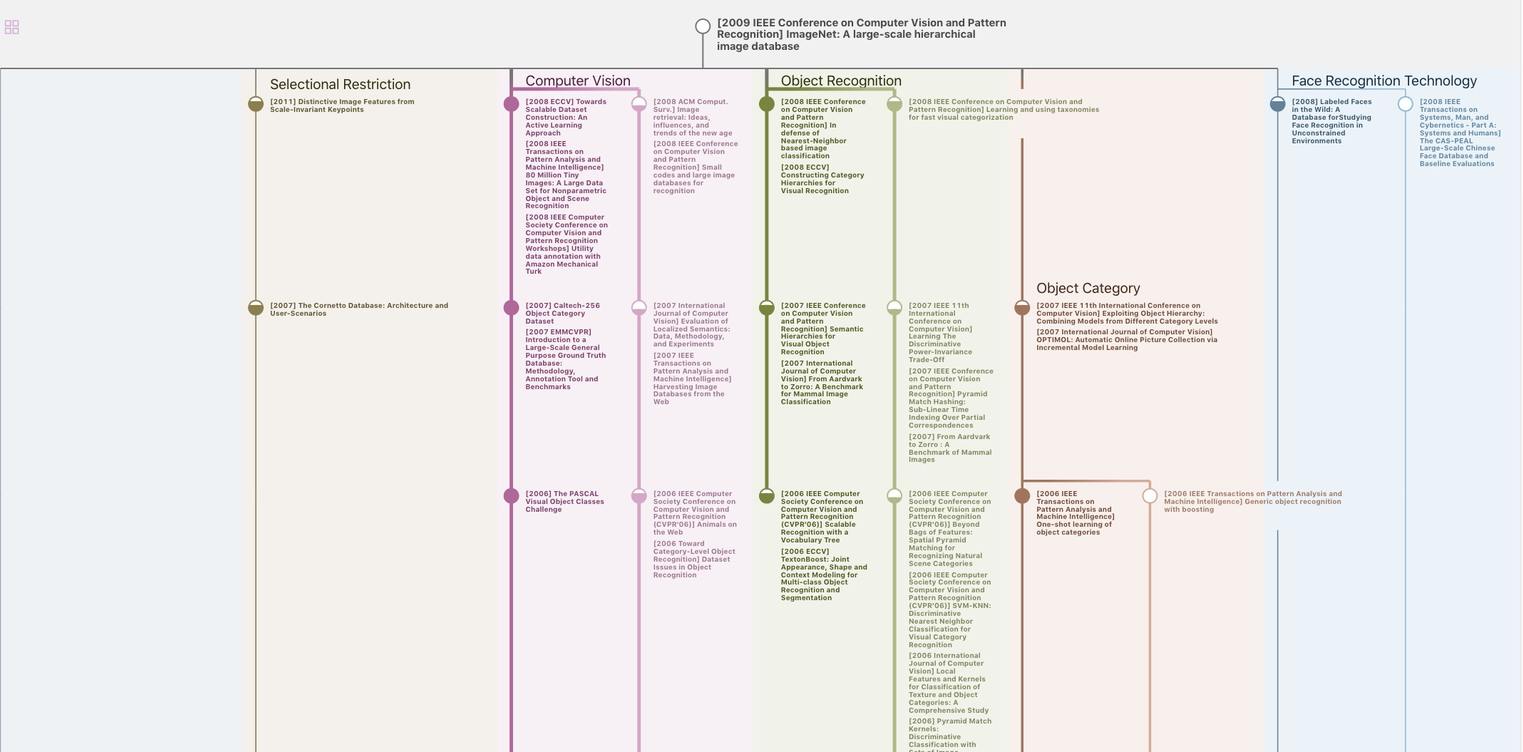
生成溯源树,研究论文发展脉络
Chat Paper
正在生成论文摘要