Cognitive-based knowledge learning framework for recommendation
KNOWLEDGE-BASED SYSTEMS(2024)
摘要
Recommender systems (RS) have been widely used in Web applications such as search engines, social media platforms and e-commerce portals. Accuracy-related metrics have always been the primary goals for RS. Most RS tend to recommend items that are most relevant or similar to users' historical records, which may narrow users' cognitive scope and lead to information cocoons. This paper proposes a Cognitive-based Knowledge Learning Framework (CKLF), which addresses this challenge by integrating cognitive psychology to the construction of the neural network. CKLF consists of two key parts: a Spreading Activation Network (SAN) and a Sequence-sensitive Attention Mechanism (SAM). The SAN uses spreading activation theory to activate diversified and high-ordered entities in an auxiliary knowledge graph, and to refine embeddings of users and items. The SAM incorporates the Ebbinghaus forgetting theory into the attention mechanism to capture evolution features of users' sequential interactions. Extensive experiments on two public benchmarks show, compared with start-of-the-art baselines, CKLF not only improves accuracy but more importantly achieves superior performance in the beyond-accuracy objectives such as diversity and serendipity. Therefore, CKLF can effectively alleviate information cocoons and improve the overall quality of RS.
更多查看译文
关键词
Recommender systems,Knowledge graph,Neural network,Attention mechanism,Beyond-accuracy objectives
AI 理解论文
溯源树
样例
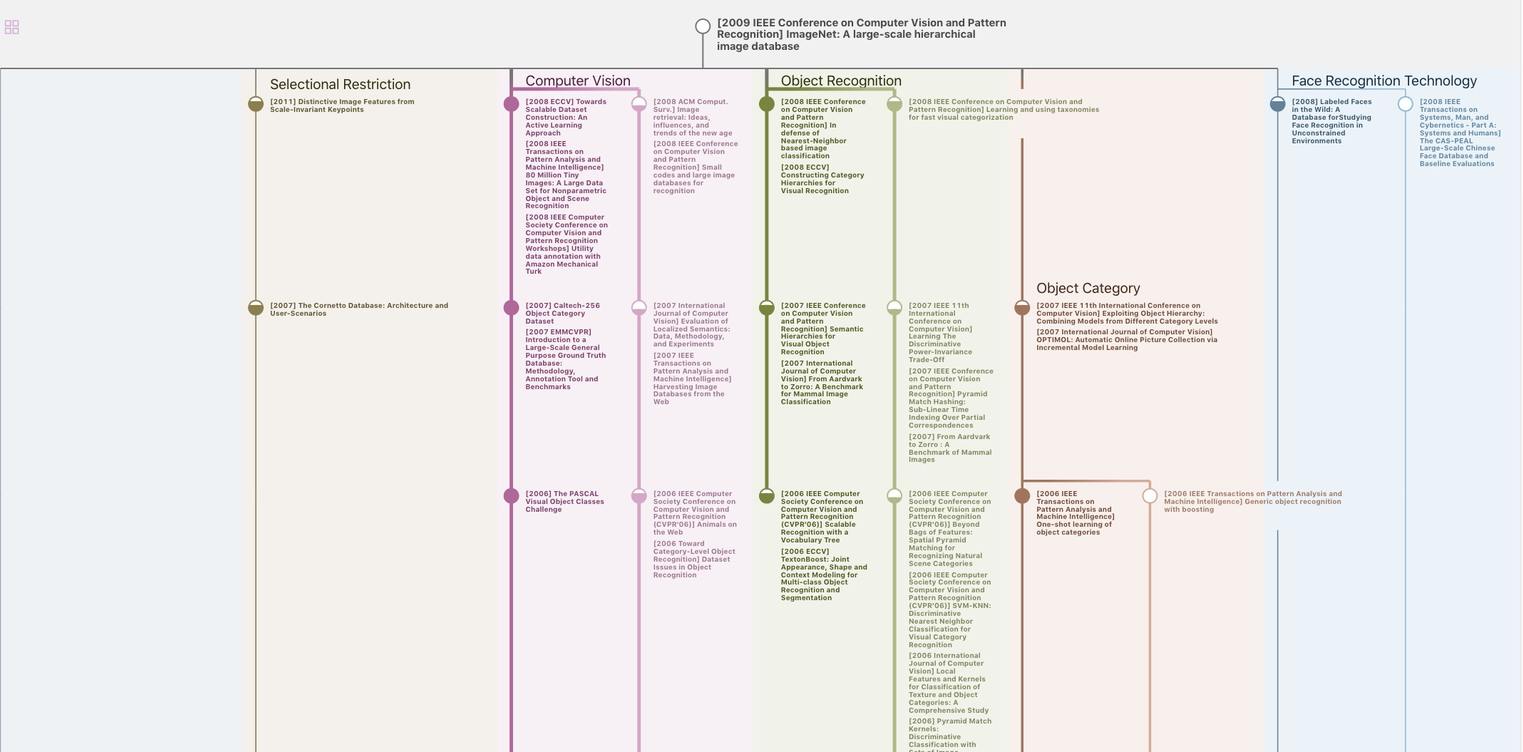
生成溯源树,研究论文发展脉络
Chat Paper
正在生成论文摘要