Graphylo: A deep learning approach for predicting regulatory DNA and RNA sites from whole-genome multiple alignments
ISCIENCE(2024)
摘要
This study focuses on enhancing the prediction of regulatory functional sites in DNA and RNA sequences, a crucial aspect of gene regulation. Current methods, such as motif overrepresentation and machine learning, often lack specificity. To address this issue, the study leverages evolutionary information and introduces Graphylo, a deep -learning approach for predicting transcription factor binding sites in the human genome. Graphylo combines Convolutional Neural Networks for DNA sequences with Graph Convolutional Networks on phylogenetic trees, using information from placental mammals' genomes and evolutionary history. The research demonstrates that Graphylo consistently outperforms both single -species deep learning techniques and methods that incorporate inter -species conservation scores on a wide range of datasets. It achieves this by utilizing a species -based attention model for evolutionary insights and an integrated gradient approach for nucleotide -level model interpretability. This innovative approach offers a promising avenue for improving the accuracy of regulatory site prediction in genomics.
更多查看译文
关键词
Transcription Factors,RNA binding proteins,Graph Convolutional Networks,phylogenetics
AI 理解论文
溯源树
样例
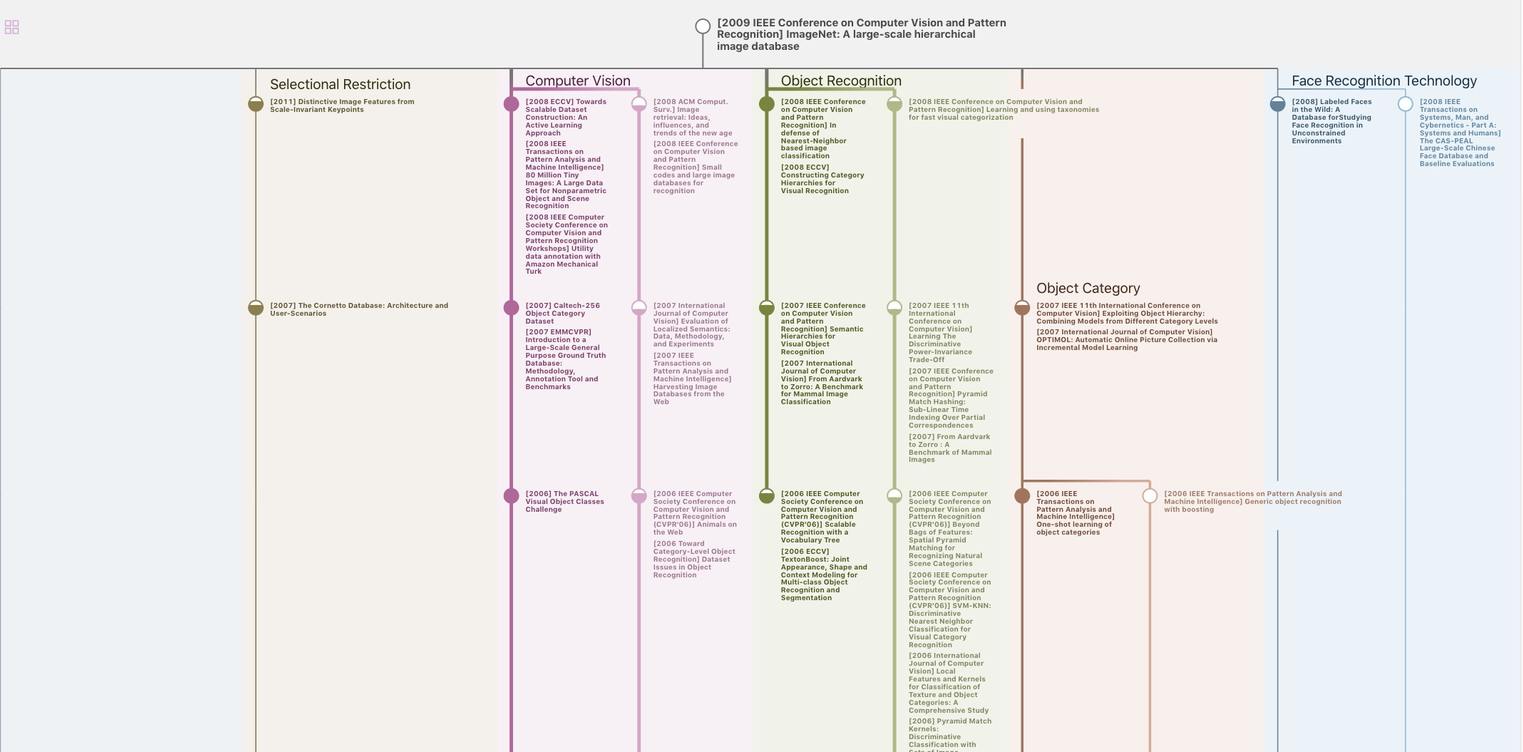
生成溯源树,研究论文发展脉络
Chat Paper
正在生成论文摘要