Multi-level cross-modal contrastive learning for review-aware recommendation
EXPERT SYSTEMS WITH APPLICATIONS(2024)
摘要
Recent studies tend to employ Contrastive Learning (CL) methods to facilitate model training by extracting self-supervised signals to mitigate data sparsity. However, existing CL-based recommendation methods have not fully exploited the rich semantic information present in multi -modal data. To address these limitations, we propose a new CL-based recommendation framework named Multi-level Cross-modal Contrastive Learning (MCCL), which aims to construct multi-level contrastive learning to fully exploit the intra- and inter-modal semantic information in a self-supervised manner. Specifically, we innovatively consider user interaction and semantic review as two distinct semantic modalities, and devise two modal-specific contrastive learning strategies to enhance intra-modal learning. Furthermore, we leverage the semantic consistency between modalities to construct a multi-level cross-modal contrastive learning framework. Finally, a multi-task learning method is employed for collaborative optimization across multiple tasks. We verify the efficacy of MCCL via comprehensive experiments on three real-world datasets. MCCL achieves a significant performance improvement over the state-of-the-art baseline models.
更多查看译文
关键词
Recommendation systems,Contrastive learning,Cross-modal semantic consistency,Graph neural network,Bert pre-trained model
AI 理解论文
溯源树
样例
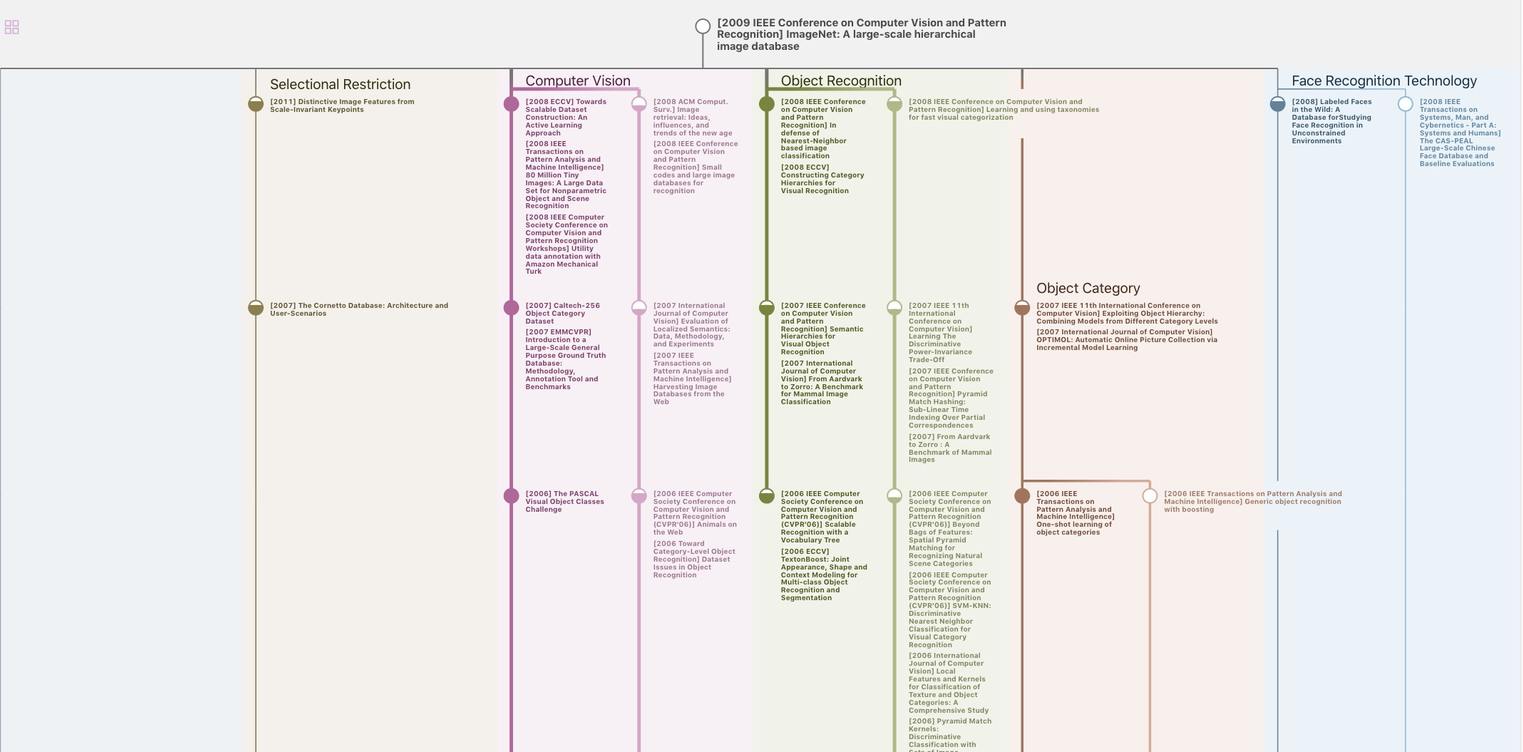
生成溯源树,研究论文发展脉络
Chat Paper
正在生成论文摘要