A comparison of knowledge-based dose prediction approaches to assessing head and neck radiotherapy plan quality
medrxiv(2024)
摘要
PURPOSE: Recent studies demonstrate deep learning dose prediction algorithms may produce results like those of traditional knowledge-based planning tools. In this exploratory study, we compared 2D DVH-based knowledge-based planning tools and METHODS: Pre-validated 2D and 3D dose prediction models were applied to 58 patients with head and neck cancer treated under RTOG 0522 obtained from The Cancer Imaging Archive (TCIA). The 2D model was used to predict dose-volume histogram bands for seven organs at risk (OARs; brainstem, spinal cord, oral cavity, larynx, mandible, right parotid, and left parotid). A 3D dose prediction model was used to predict 3D dose distributions, based on computed tomography images, OAR contours, planning target volumes and prescriptions. The mean and D1% to the seven OARs for the 2D and 3D dose prediction models were compared. Further post predictive analysis was done to quantify the predicted 3D dose sparing for all normal tissues. RESULTS: The two models predicted similar dose sparing to the OARs, with a mean difference of 1.4± 5.5 Gy across all evaluated dose metrics. When looking at the sparing of non-OAR normal tissue regions, the 3D model predicted a mean dose reduction to normal tissue regions of 6.4±3.0 Gy when compared with the clinical dose. CONCLUSION: 2D and 3D dose predictions are comparable at predicting dose reductions to OARs. The 3D approach allows for dose visualization, which may support further sparing of normal tissues not typically drawn as OARs on head and neck plans.
### Competing Interest Statement
This project was performed by the radiation planning team at MD Anderson, which receives funding from the NCI, the Cancer Prevention & Research Institute of Texas, the Wellcome Trust, and Varian Medical Systems. A premarket notification 510(k) for the Radiation Planning Assistant has been cleared for commercial distribution by the U.S. Food and Drug Administration, however, it is yet to be marketed.
### Funding Statement
This study was partially funded by the Cancer Prevention & Research Institute of Texas and Varian Medical Systems.
### Author Declarations
I confirm all relevant ethical guidelines have been followed, and any necessary IRB and/or ethics committee approvals have been obtained.
Yes
The details of the IRB/oversight body that provided approval or exemption for the research described are given below:
TCIA data was used after required application.
I confirm that all necessary patient/participant consent has been obtained and the appropriate institutional forms have been archived, and that any patient/participant/sample identifiers included were not known to anyone (e.g., hospital staff, patients or participants themselves) outside the research group so cannot be used to identify individuals.
Yes
I understand that all clinical trials and any other prospective interventional studies must be registered with an ICMJE-approved registry, such as ClinicalTrials.gov. I confirm that any such study reported in the manuscript has been registered and the trial registration ID is provided (note: if posting a prospective study registered retrospectively, please provide a statement in the trial ID field explaining why the study was not registered in advance).
Yes
I have followed all appropriate research reporting guidelines, such as any relevant EQUATOR Network research reporting checklist(s) and other pertinent material, if applicable.
Yes
All treatment plan data is available online at
更多查看译文
AI 理解论文
溯源树
样例
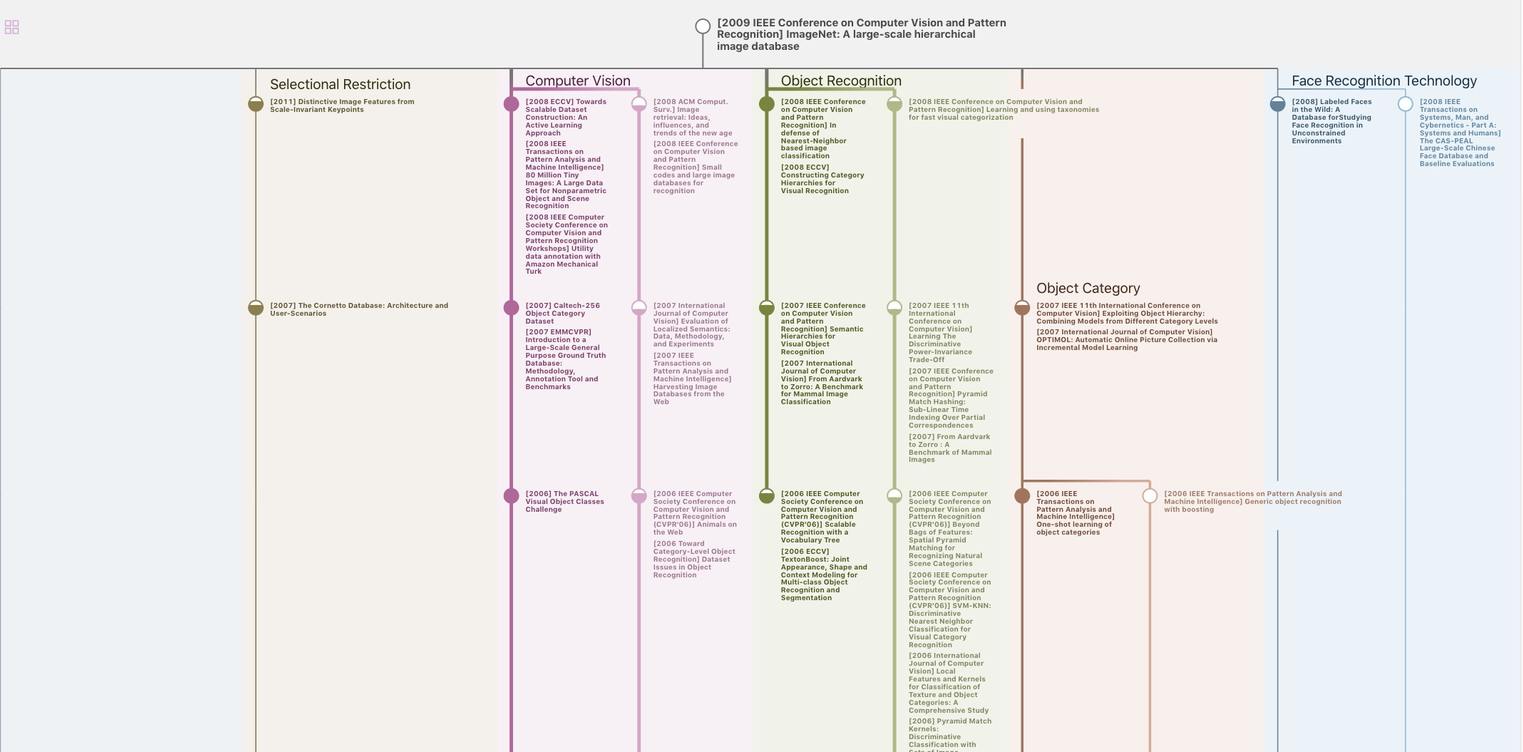
生成溯源树,研究论文发展脉络
Chat Paper
正在生成论文摘要