Deep learning and beamforming comparison of source ranging in a laboratory tank
Journal of the Acoustical Society of America(2023)
摘要
Deep learning has been shown to be useful for ocean acoustics applications, such as source ranging. The variable nature of the ocean causes difficulty when attempting to apply trained models to new datasets. To explore ways to train supervised deep learning models that are robust to environmental variability, laboratory tank measurements can be used. A laboratory tank presents a system where uncertainty in the acoustic environment can be adjusted in a more controlled manner. In this work, recordings of ultrasonic chirps (50–200 kHz) are obtained at different source-receiver positions. Time-averaged spectral density levels are used to train one-dimensional convolutional neural networks that predict source-receiver range. The trained networks are tested on data obtained under different conditions. Data augmentation techniques are applied to increase the models’ robustness in the presence of limited uncertainty. Metrics such as root mean-squared error and mean absolute percent error are used to quantify the model’s performance. The results from deep learning with single channel recordings and multi-channel recordings are compared with traditional beamforming methods on the multi-channel data. [Work supported by the Office of Naval Research.]
更多查看译文
AI 理解论文
溯源树
样例
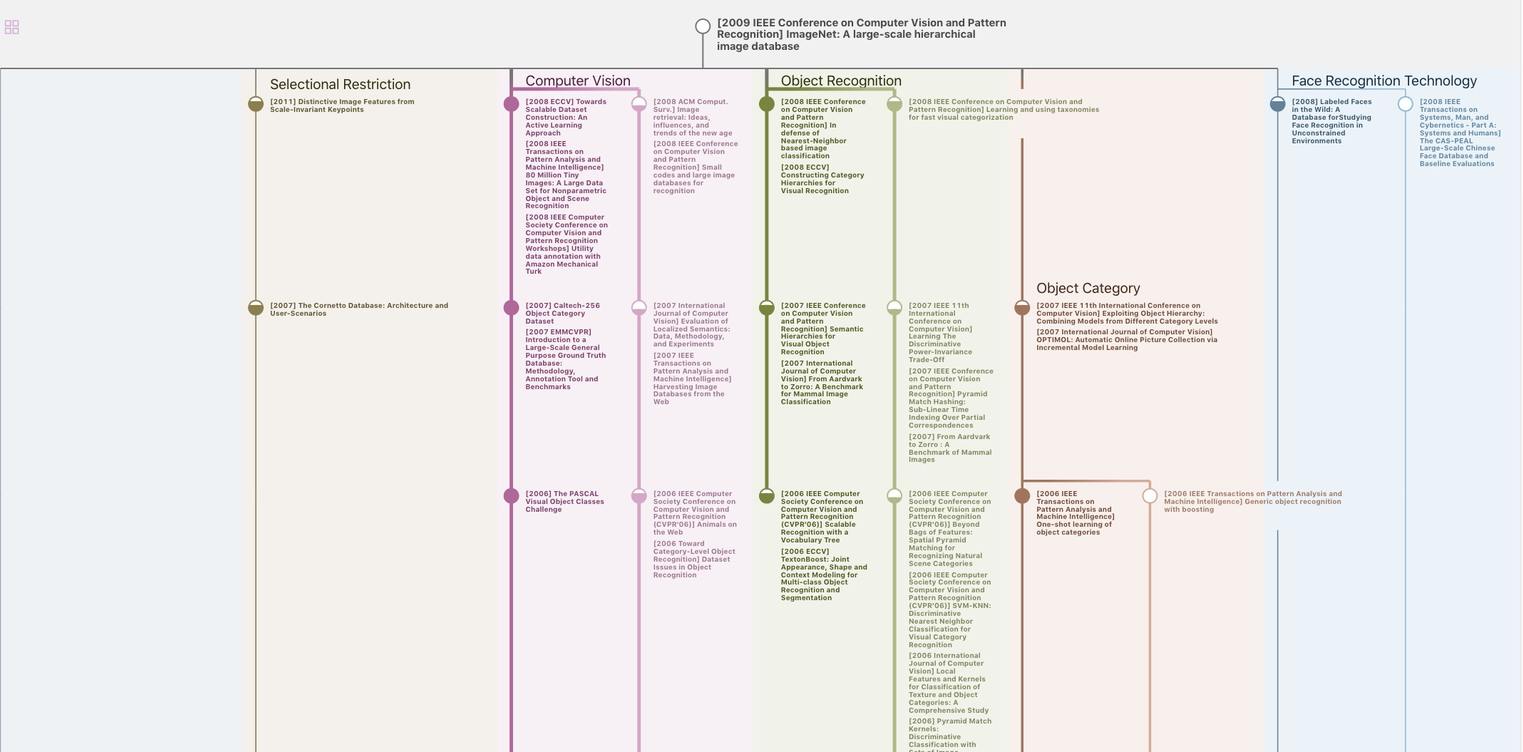
生成溯源树,研究论文发展脉络
Chat Paper
正在生成论文摘要