Prediction Pathological Structure of Computed Tomographic Colonography Polyps via Machine Learning
2022 IEEE Nuclear Science Symposium and Medical Imaging Conference (NSS/MIC)(2022)
摘要
Computed tomographic colonography (CTC) has shown to be an effective means of detecting the precursor polyps leading to colorectal cancer (CRC). Accurately diagnosing these polyps can assist the overall treatment plan and follow-up examination determination to prevent CRC. In this work, we examine a machine learning approach to predicting the three main pathological structure type of polyps, comparing hyperplastic and serrated adenomas (HS), tubular adenomas (TA), and advanced neoplasms (AN). We find that imaging textures can differentiate any two pathological groups with AUC scores ranging from 0.624-0.749 for polyps less than 10mm in size and 0.731-0.887 for polyps greater than 10mm. Classification of all three pathology groups simultaneously shows a sensitivity of 61.6% for identifying the highest risk group of advanced neoplasms.
更多查看译文
关键词
Machine Learning,Structural Pathology,CT Colonography,Colorectal Cancer,Colorectal Adenoma,Pathological Groups,Random Forest,Validation Set,Classification Performance,Machine Learning Models,Binary Classification,Texture Features,Colonoscopy,Image Texture,Colorectal Cancer Incidence,Gray Level Co-occurrence Matrix,Colorectal Polyps,Colorectal Cancer Mortality,Number Of Polyps,Large Polyps
AI 理解论文
溯源树
样例
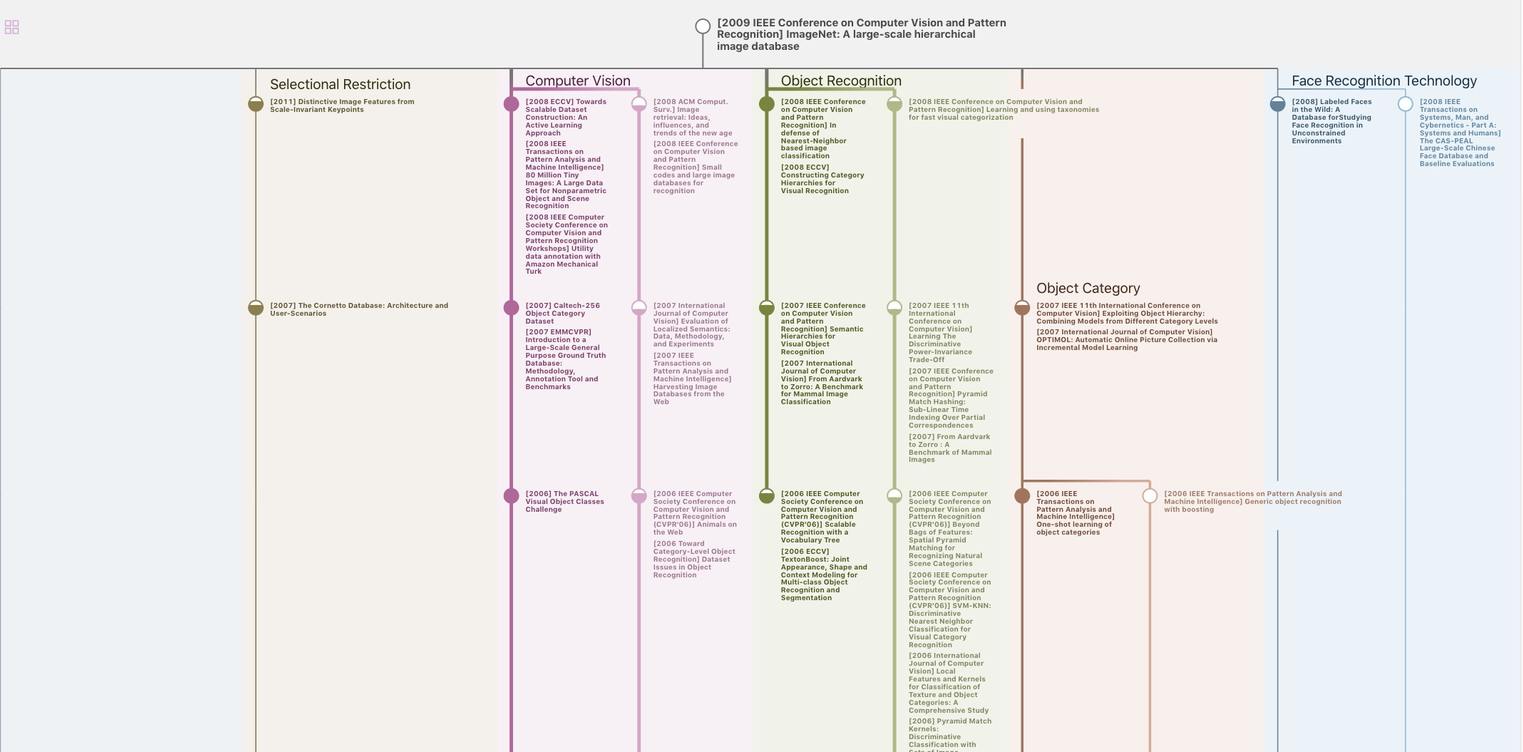
生成溯源树,研究论文发展脉络
Chat Paper
正在生成论文摘要