An Adaptive Patch Sampling Scheme for Deep Learning Based PET Image Denoising
2022 IEEE Nuclear Science Symposium and Medical Imaging Conference (NSS/MIC)(2022)
摘要
Deep learning-based approaches introduce over-smoothing effect in image denoising, degrading small-size tissue quantification. In this work, we investigated an adaptive patch sampling scheme to reduce this over-smoothing effect. Ten patients with solid lung nodules were included, each having one full-dose FDG PET image and 10 replicates of 10% low-dose PET image. For low-dose PET denoising, a patch-based strategy was used for data augmentation in training. Three networks were used: 1) 3D U-Net; 2) 3D U-Net with weighted patch sampling (U-Net-WSamp) by assigning higher sampling rate to the patches with nodule at the center during training, which can focus network training on nodule; 3) 3D U-Net with weighted loss (U-Net-WLoss), in which the L1 loss function was multiplied by a weight map with higher value for the voxels inside nodule. A "leave-one-out" approach was used to evaluate the networks across all 10 patients. The results showed all the three networks effectively reduced low-dose PET image noise. Their performances were similar in terms of normalized mean square error (NMSE), indicating that the strategy to focus training on nodules in both U-Net-WSamp and U-Net-WLoss did not degrade the denoising performance on the voxels outside nodules. U-Net-WSamp provided a slightly higher signal-to-noise ratio (SNR) than U-Net (3.44 ± 0.86 versus 3.41 ± 0.80) and U-Net-WLoss provided the highest SNR (3.57 ± 0.90). U-Net introduced over-smoothing effect and degraded nodule quantification with an absolute bias of 8.24% ± 4.13%. U-Net-WLoss was not able to reduce nodule bias (8.48% ± 3.36%). U-Net-WSamp caused less blurring effect than U-Net in the majority of the patients and reduced nodule bias to 7.86% ± 3.70%. In conclusion, the proposed adaptive patch sampling scheme can reduce the over-smoothing effect and improve nodule quantification in deep learning-based PET image denoising.
更多查看译文
关键词
Denoising,Adaptive Scheme,Adaptive Sampling,Sample Patches,Adaptive Sampling Strategy,Weight Loss,Signal-to-noise,Mean Square Error,Majority Of Patients,Lung Nodules,L1 Loss,Deep Learning-based Approaches,Weight Map,Normalized Mean Square Error,Augmented Training,Normalized Mean Square,Solid Nodules,Low-dose Images,Training Data Augmentation,3D U-Net,List-mode Data
AI 理解论文
溯源树
样例
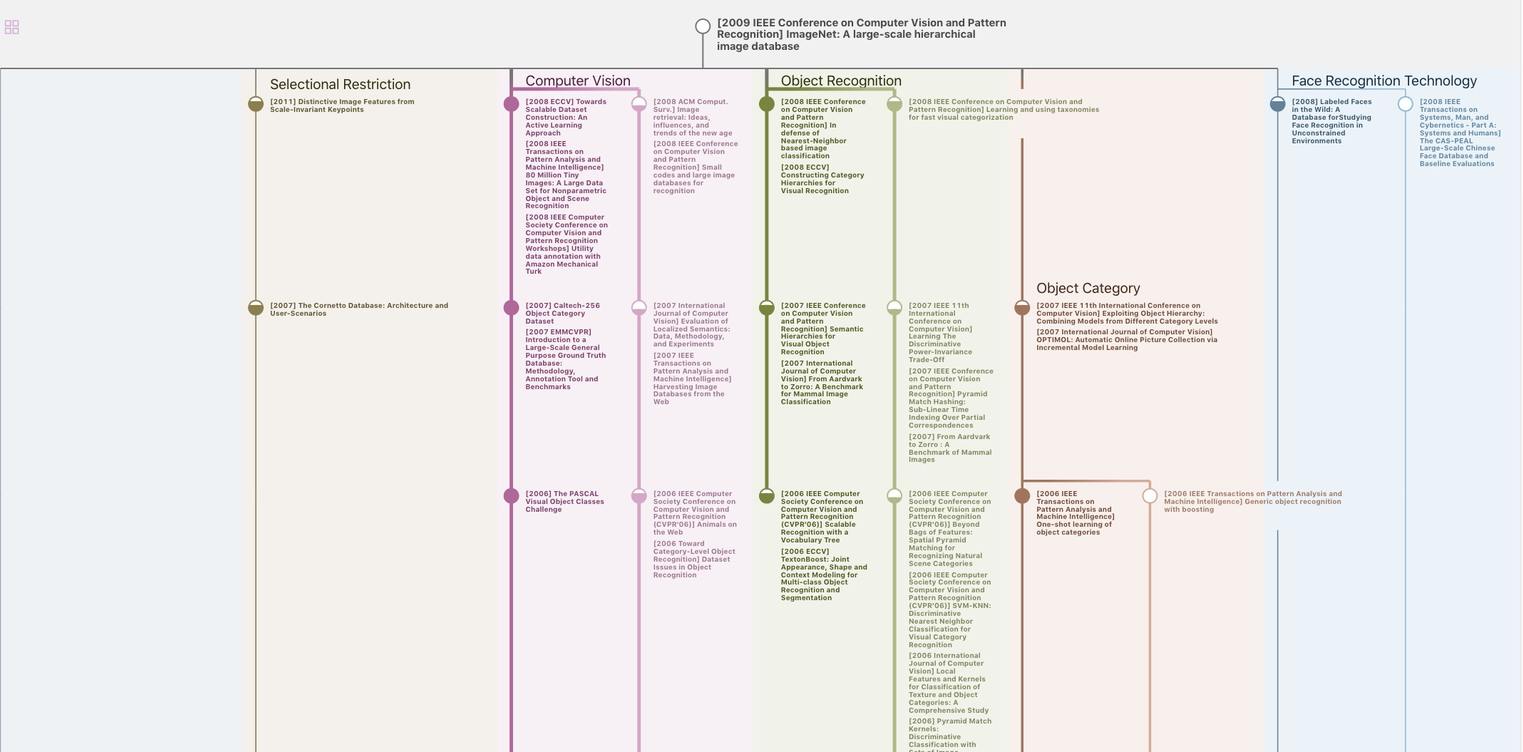
生成溯源树,研究论文发展脉络
Chat Paper
正在生成论文摘要