A Pilot Study of Attenuation Correction for Static and Dynamic 13N-Ammonia Cardiac PET Using a Conditional Generative Adversarial Network
2022 IEEE Nuclear Science Symposium and Medical Imaging Conference (NSS/MIC)(2022)
摘要
Attenuation correction (AC) of myocardial perfusion PET remains challenging in routine clinical practice due to the potential misalignment of PET and CT data, which results in image artifacts and inaccurate quantitative parameters in PET/CT. In this work, a 3D conditional generative adversarial network (cGAN) was used to directly generate attenuation-corrected PET (PET-AC) images from non-attenuation-corrected PET (PET-NAC) images. This work included 60 patients who underwent rest
13
N-ammonia PET/CT examinations. For each patient, one static PET and twenty-one dynamic PET frames were acquired with both AC and NAC. The network was firstly trained by 3D paired static
13
N-ammonia PET-NAC and CT-based AC PET (PET-CTAC) images in an end-to-end fashion (PET-DLAC). Then the pre-trained network was fine-tuned (FT) by 3D paired dynamic PET-NAC and PET-CTAC frames (PET-DLAC-FT). Five-fold cross-validation was adopted to train and test the network. Qualitative and quantitative analyses were performed by voxel-wise analysis on the standard intake values (SUV), e.g., RMSE, PSNR, SSIM, and joint histogram analysis versus the PET-CTAC images as reference. Furthermore, quantitative kinetic parameters and time activity curves of the dynamic PET were performed to evaluate the PET-DLAC-FT method. Qualitative and quantitative results show the proposed PET-DLAC and PET-DLAC-FT methods reduce attenuation artifacts significantly and achieve comparable performances with clinical CTAC. Transfer learning-based AC is feasible for dynamic cardiac PET.
更多查看译文
关键词
Generative Adversarial Networks,Attenuation Correction,Conditional Generative Adversarial Network,Root Mean Square Error,Peak Signal-to-noise Ratio,Standardized Uptake Value,Image Artifacts,Voxel-wise Analysis,Time-activity Curves,Dynamic Frames,Inaccurate Parameters,Convolutional Neural Network,Data Augmentation,Transverse Plane,Dynamic Imaging,Kinetic Imaging
AI 理解论文
溯源树
样例
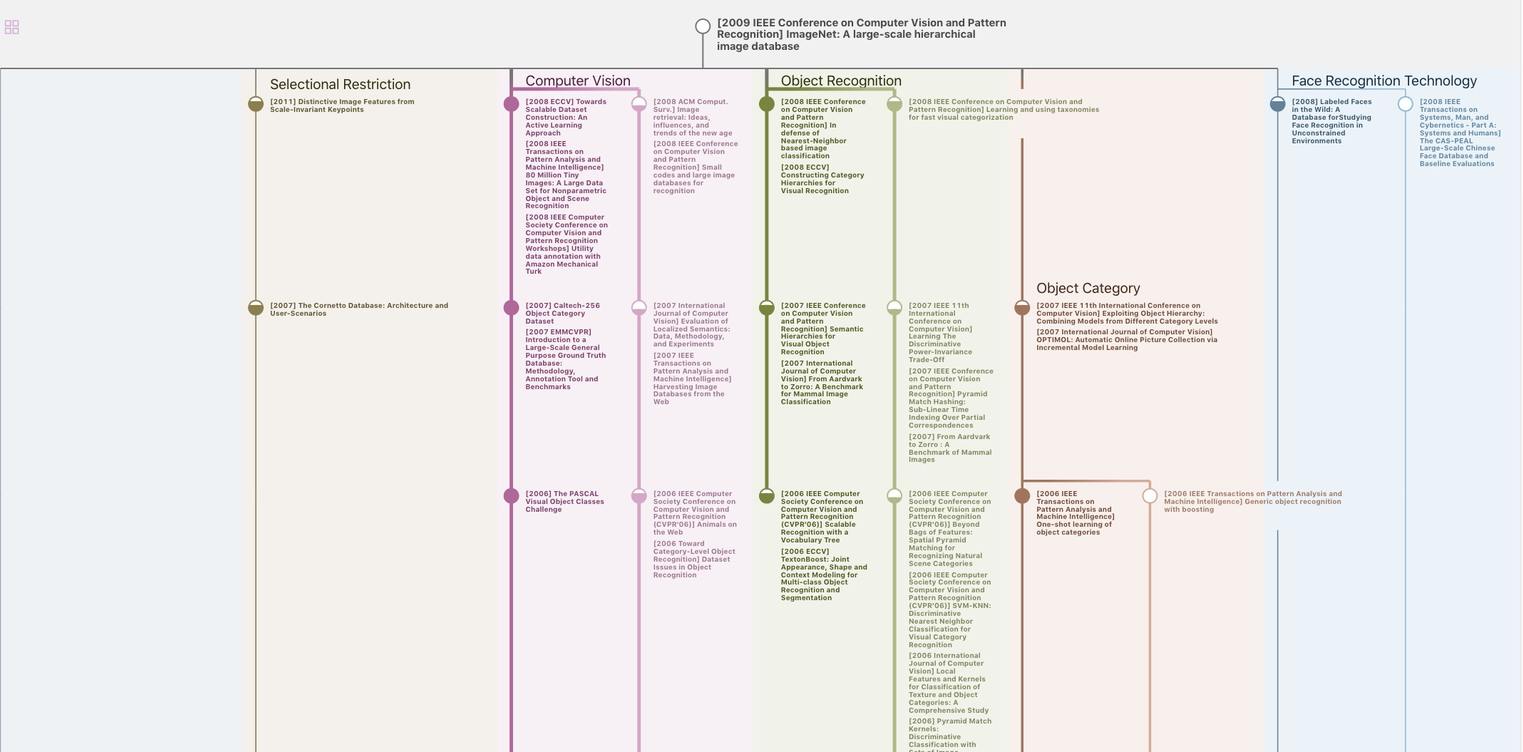
生成溯源树,研究论文发展脉络
Chat Paper
正在生成论文摘要