Collaborative Multi-Institutional Prostate Lesion Segmentation from MR images Using Deep Federated Learning Framework
2022 IEEE Nuclear Science Symposium and Medical Imaging Conference (NSS/MIC)(2022)
摘要
To develop a robust and generalizable deep learning (DL) model gathering a massive and heterogenous dataset is crucial as the DL performances could be varied across different acquisition and reconstruction settings in the real clinical situation. Furthermore, sharing data with third parties is highly limited because of legal, ethical, security, and privacy issues. To address the aforementioned challenges, Federated learning (FL) allows one to train a DL model without sharing the data between different centers in a distributed and decentralized manner. In the current study, we developed deep FL-based models for intraprostatic lesion segmentation using FL approaches and compared their results by center based. Altogether 400 histologically proven prostate cancer patients with T2-weighted MRI images from eight different centers were enrolled. Dynamic data augmentation techniques for flipping left/right, elastic deformation, and random cropping was adopted. Two-stage cascaded U-Net consisting of modified 3D U-Ne, and Dual Attention 2D U-Net were implemented as the core of DL segmentation. MRI images and a prostate mask were used as input in this network. In addition, Federated Averaging (FedAvg) algorithm was implemented in this study. All evaluations were performed on 30% of test sets. In terms of dice and Jaccard coefficient, CeBa and achieved 0.77 ± 0.06 vs. 0.84 ± 0.05 (CI95%: 0.76 - 0.79 vs. 0.82 - 0.85) and 0.63 ± 0.09 vs. 0.72 ± 0.08 and (CI95%:0.61 - 0.65 vs. 0.70 - 0.74). We set out to develop a DL-based automated algorithm capable of segmenting intra-prostatic lesions using T2W MR images. Due to the complex structure and low-contrast nature of the intraprostatic lesion in T2W MR images, our proposed algorithm performed very well compared to manual segmentation across different centers. Our FL algorithms outperformed center base algorithms in which each center developed a model using their local dataset, which addresses data sharing between different centers.
更多查看译文
关键词
Federated learning,Deep learning,MRI,Prostate
AI 理解论文
溯源树
样例
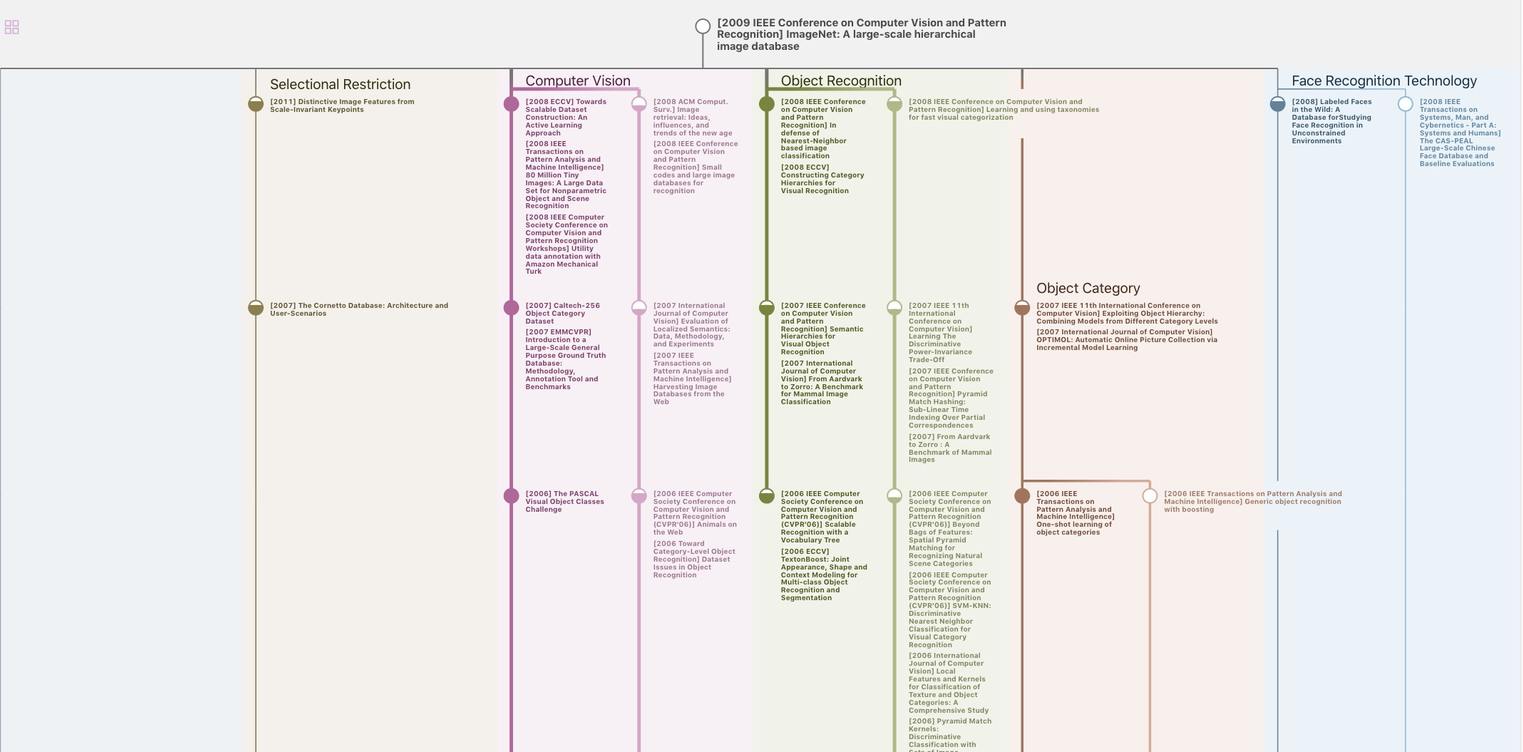
生成溯源树,研究论文发展脉络
Chat Paper
正在生成论文摘要