Emission-Based Attenuation Correction for Small Animal PET/MR Using Conditional Generative Adversarial Networks
2022 IEEE Nuclear Science Symposium and Medical Imaging Conference (NSS/MIC)(2022)
摘要
In this work, we applied conditional generative adversarial network (cGAN) for emission-based attenuation correction (AC) in small animals and evaluated the performance of our solution on the generated attenuation map and the AC reconstructed images. The non-attenuated and scatter corrected reconstructed image was fed into the cGAN that was trained to generate the corresponding attenuation map. This generated attenuation map was then converted into a pCT and fed into the image reconstruction software one more time to correct the generated PET image for photon attenuation. Using our method, we can generate high quality PET images without performing a CT scan, PSNR of 43.49dB and SSIM of 0.998 compared to the CT-based AC PET.
更多查看译文
关键词
Small Animal,Generative Adversarial Networks,Attenuation Correction,Conditional Generative Adversarial Network,Image Reconstruction,Positron Emission Tomography Imaging,Peak Signal-to-noise Ratio,Soft Tissue,Network Training,Quantitative Comparison,Point Spread Function,Air Cavity
AI 理解论文
溯源树
样例
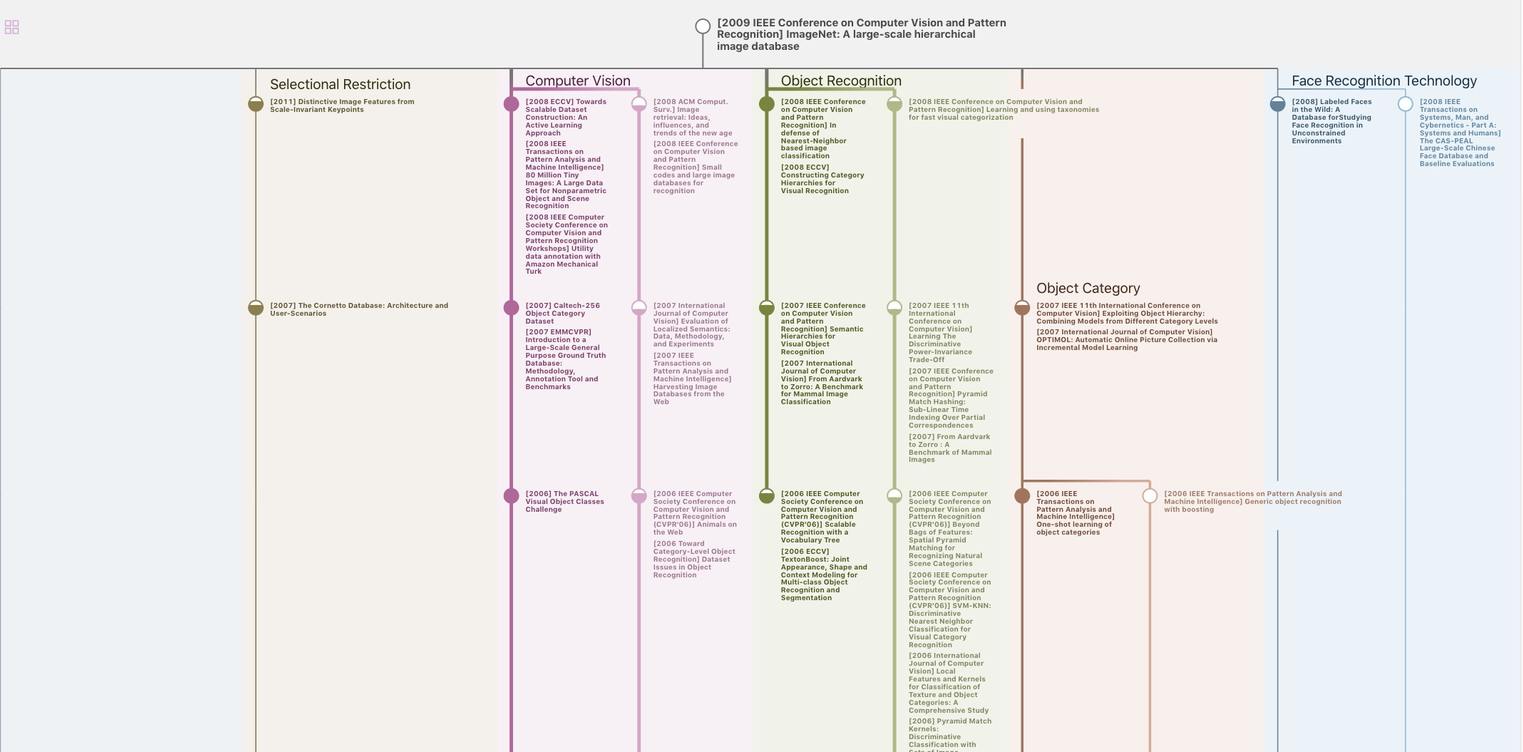
生成溯源树,研究论文发展脉络
Chat Paper
正在生成论文摘要