Predicting Protein Structure Using Structural Feature-based Hybrid Genetic Algorithm.
2023 7th International Symposium on Computer Science and Intelligent Control (ISCSIC)(2023)
摘要
Over the last decades, the performance of protein structure prediction (PSP) has been greatly improved. However, predicting accurate structures with single sequence information remains challenging for the proteins without any homologous sequence. Angle Probability List (APL) deriving from the Protein Data Bank (PDB), representing structural feature of amino acid residues, has been reported to help determine conformational preferences of all residues when applying PSP method. In this work, we proposed a structural feature-based hybrid genetic algorithm (SF-HGA) to tackle PSP problem. The algorithm combines global optimization of GA with fine search by Monte Carlo minimization. The SFHGA took advantage of APL and three filtering operators to generate initial population. The population was divided into three subpopulations, and each adopted different evolutionary patterns for maitaining the diversity of the individuals. A local search strategy based on Monte Carlo minimization was constructed to enhance optimizing capability. The SF-HGA was verified on benchmarking dataset with 24 diverse proteins. The results shows that our method can predict three-dimensional structure with similar folding to the experimental ones for most of target sequences. Furthermore, the SF-HGA achieved comparable solutions to the competitive methods, and outperformed them in some cases. The SF-HGA can be considered an effective contribution to the PSP field. Especially, the method is advantageous to PSP in the absence of available homologous template or multiple sequence alignment.
更多查看译文
关键词
Protein structure,conformational sampling,structural feature,local search
AI 理解论文
溯源树
样例
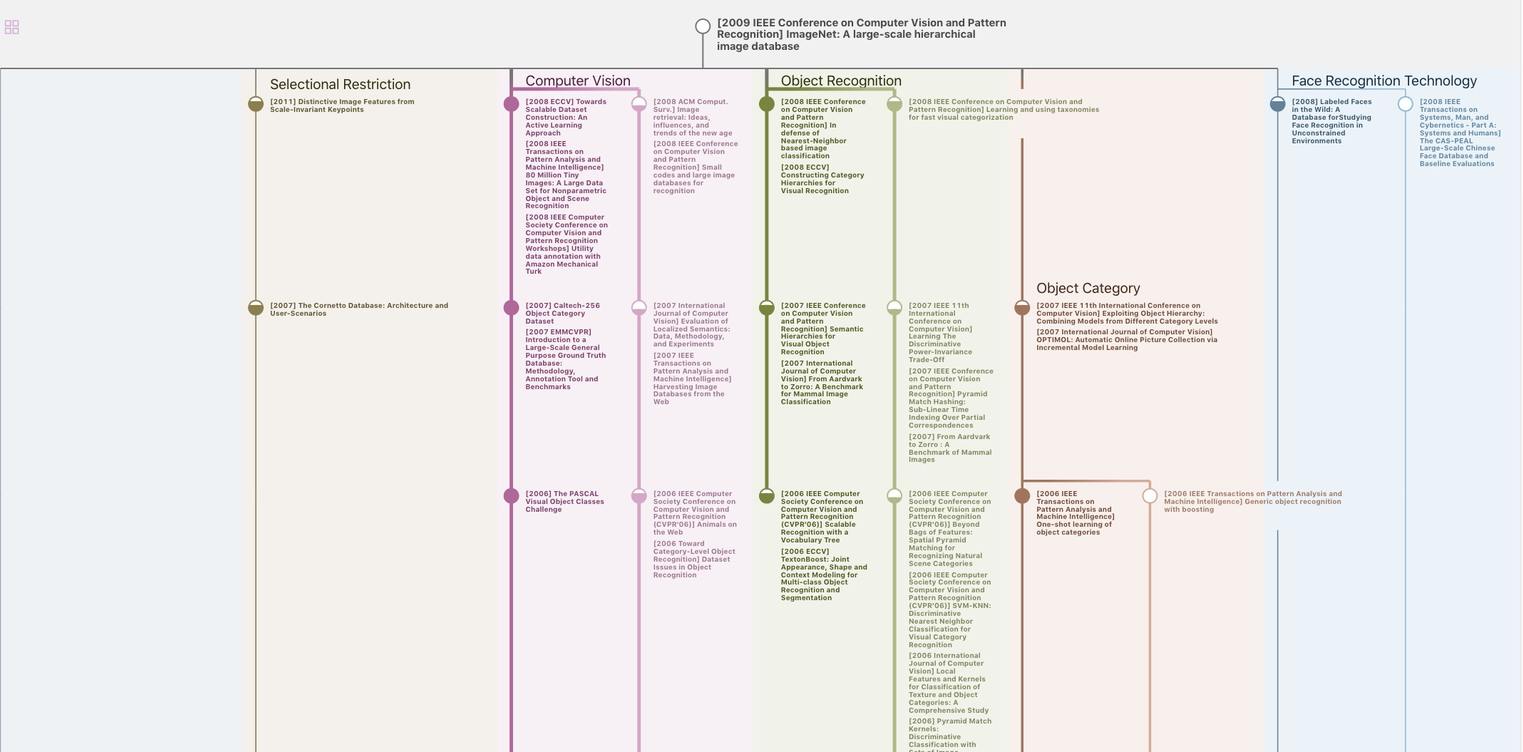
生成溯源树,研究论文发展脉络
Chat Paper
正在生成论文摘要