Asynchronous Robust Aggregation Method with Privacy Protection for IoV Federated Learning
WORLD ELECTRIC VEHICLE JOURNAL(2024)
摘要
Due to the wide connection range and open communication environment of internet of vehicle (IoV) devices, they are susceptible to Byzantine attacks and privacy inference attacks, resulting in security and privacy issues in IoV federated learning. Therefore, there is an urgent need to study IoV federated learning methods with privacy protection. However, the heterogeneity and resource limitations of IoV devices pose significant challenges to the aggregation of federated learning model parameters. Therefore, this paper proposes an asynchronous robust aggregation method with privacy protection for federated learning in IoVs. Firstly, we design an asynchronous grouping robust aggregation algorithm based on delay perception, combines intra-group truth estimation with inter-group delay aggregation, and alleviates the impact of stragglers and Byzantine attackers. Then, we design a communication-efficient and security enhanced aggregation protocol based on homomorphic encryption, to achieve asynchronous group robust aggregation while protecting data privacy and reducing communication overhead. Finally, the simulation results indicate that the proposed scheme could achieve a maximum improvement of 41.6% in model accuracy compared to the baseline, which effectively enhances the training efficiency of the model while providing resistance to Byzantine attacks and privacy inference attacks.
更多查看译文
关键词
federated learning,parameter aggregation,privacy protection,internet of vehicles,Byzantine attacker
AI 理解论文
溯源树
样例
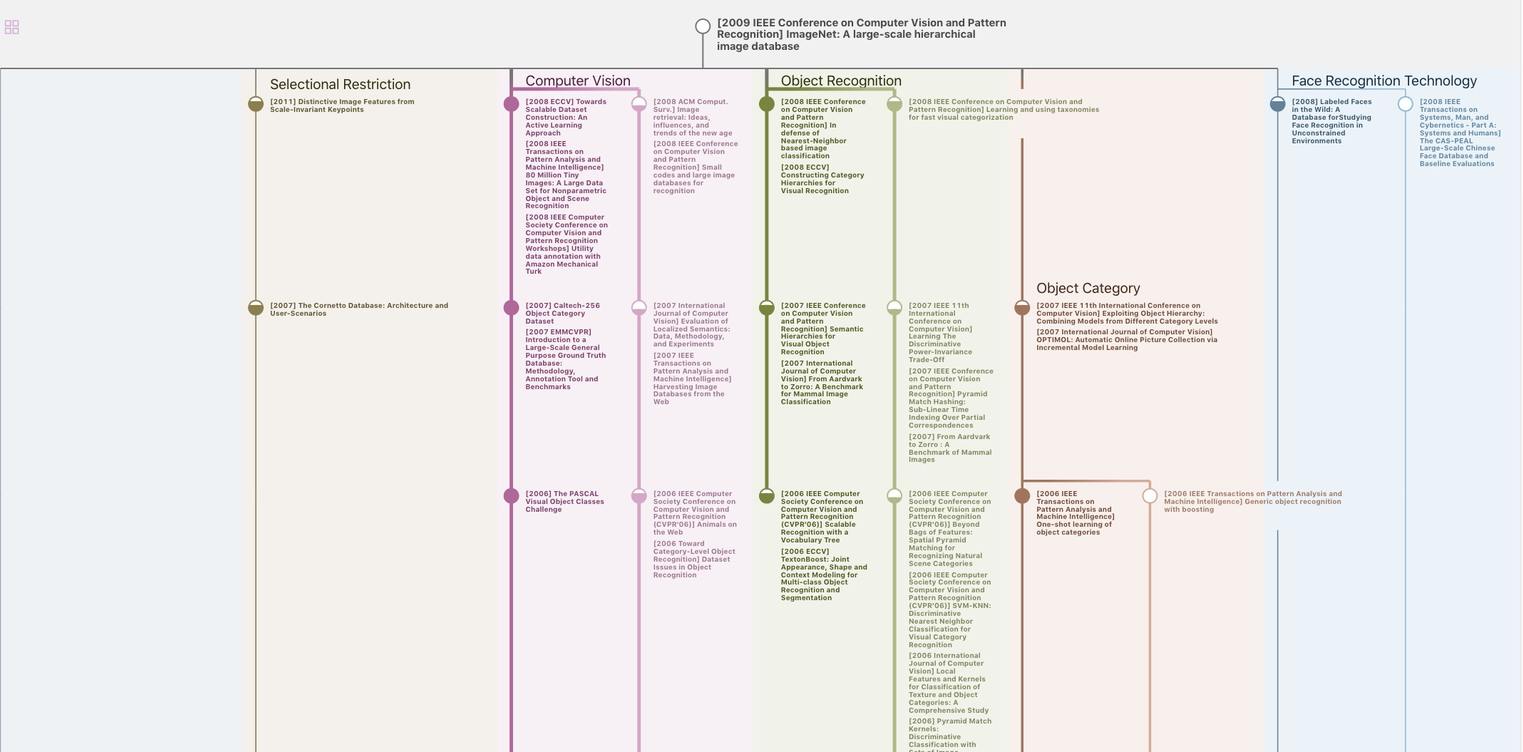
生成溯源树,研究论文发展脉络
Chat Paper
正在生成论文摘要