Deep_Learning_Based_Air_Conditioning_Set_Temperature_Prediction_with_Meteorological_Data
2023 IEEE Sustainable Power and Energy Conference (iSPEC)(2023)
摘要
Air conditioning makes up a substantial portion of building energy consumption. Analyzing the correlation between air conditioning set temperature, load, as well as meteorological factors is critical for studying load scheduling strategies of air conditioning. Among them, the air conditioning set temperature is an essential factor that affects building energy consumption and user comfort. Predicting the air conditioning set temperature reasonably based on meteorological conditions and load is a challenging problem. This paper presents a deep learning approach integrating a convolutional neural network (CNN) with long short-term memory (LSTM) for modeling and forecasting air conditioning set temperatures. The hybrid CNN-LSTM approach introduced in this study utilizes the CNN to extract local features of meteorological factors and air conditioning load. Then, it performs a time series analysis using the LSTM model. The proposed approach is employed for analyzing air conditioning operational data collected in a specific region within Zhejiang Province, China, and the prediction results are compared in four other ways: recurrent neural network, backpropagation, LSTM, and bidirectional long short-term memory. The results validate the superior prediction accuracy of the proposed method compared to the four alternative deep learning models. Furthermore, the generalization capability of the proposed CNN-LSTM model is evaluated by conducting tests on a separate dataset of users belonging to the same category. It provides a reference for the implementation of non-intrusive air conditioning control.
更多查看译文
关键词
Air conditioning load,Setting temperature prediction,Deep learning,CNN-LSTM
AI 理解论文
溯源树
样例
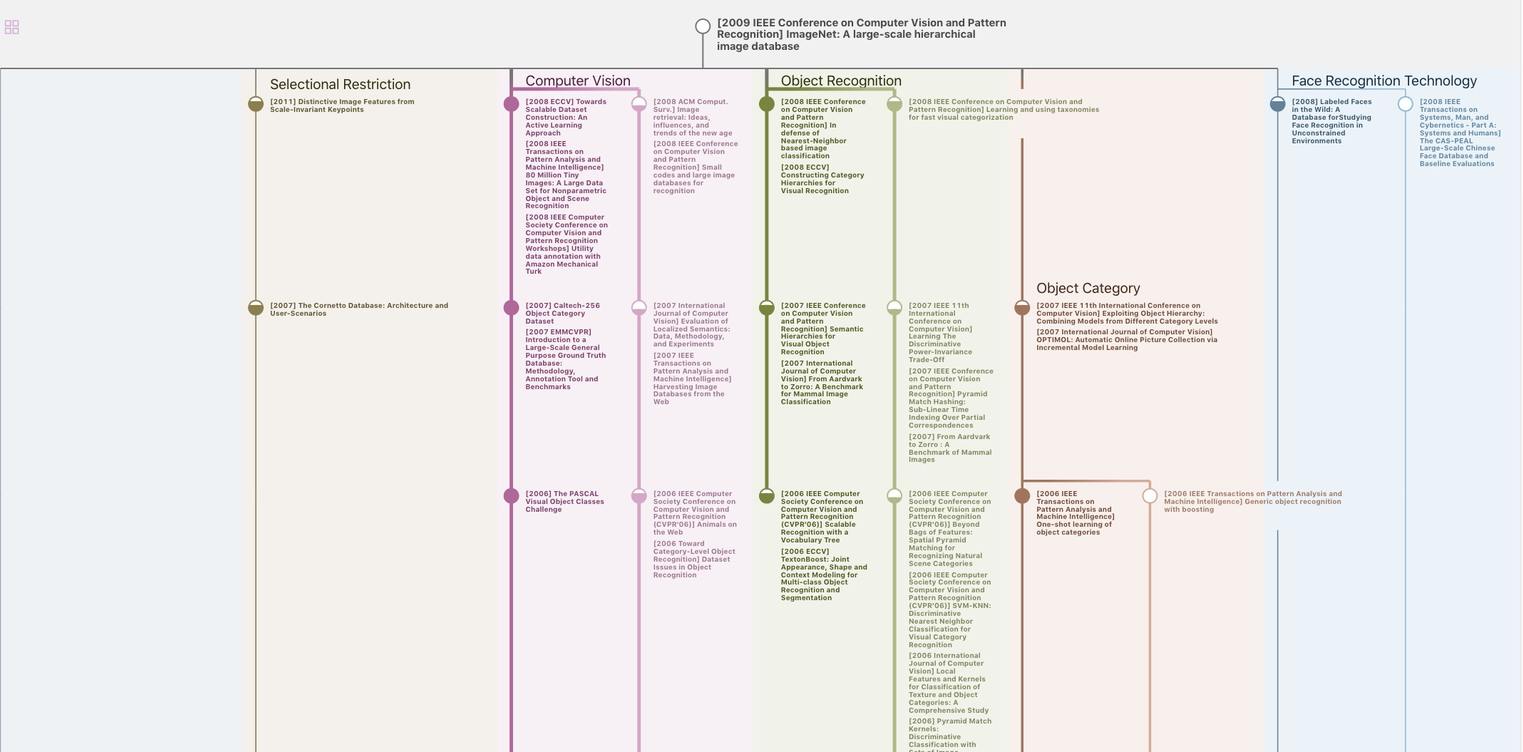
生成溯源树,研究论文发展脉络
Chat Paper
正在生成论文摘要