Estimation of Soil Moisture Content Based on Fractional Differential and Optimal Spectral Index
AGRONOMY-BASEL(2024)
摘要
Applying hyperspectral remote sensing technology to the prediction of soil moisture content (SMC) during the growth stage of soybean emerges as an effective approach, imperative for advancing the development of modern precision agriculture. This investigation focuses on SMC during the flowering stage under varying nitrogen application levels and film mulching treatments. The soybean canopy's original hyperspectral data, acquired at the flowering stage, underwent 0-2-order differential transformation (with a step size of 0.5). Five spectral indices exhibiting the highest correlation with SMC were identified as optimal inputs. Three machine learning methods, namely support vector machine (SVM), random forest (RF), and back propagation neural network (BPNN), were employed to formulate the SMC prediction model. The results indicate the following: (1) The correlation between the optimal spectral index of each order, obtained after fractional differential transformation, and SMC significantly improved compared to the original hyperspectral reflectance data. The average correlation coefficient between each spectral index and SMC under the 1.5-order treatment was 0.380% higher than that of the original spectral index, with mNDI showing the highest correlation coefficient at 0.766. (2) In instances of utilizing the same modeling method with different input variables, the SMC prediction model's accuracy follows the order: 1.5 order > 2.0 order > 1.0 order > 0.5 order > original order. Conversely, with consistent input variables and a change in the modeling method, the accuracy order becomes RF > SVM > BPNN. When comprehensively assessing model evaluation indicators, the 1.5-order differential method and RF method emerge as the preferred order differential method and model construction method, respectively. The R-2 for the optimal SMC estimation model in the modeling set and validation set were 0.912 and 0.792, RMSEs were 0.005 and 0.004, and MREs were 2.390% and 2.380%, respectively. This study lays the groundwork for future applications of hyperspectral remote sensing technology in developing soil moisture content estimation models for various crop growth stages and sparks discussions on enhancing the accuracy of these different soil moisture content estimation models.
更多查看译文
关键词
soybean,hyperspectrum,fractional order differentiation,optimal spectral index,soil moisture content
AI 理解论文
溯源树
样例
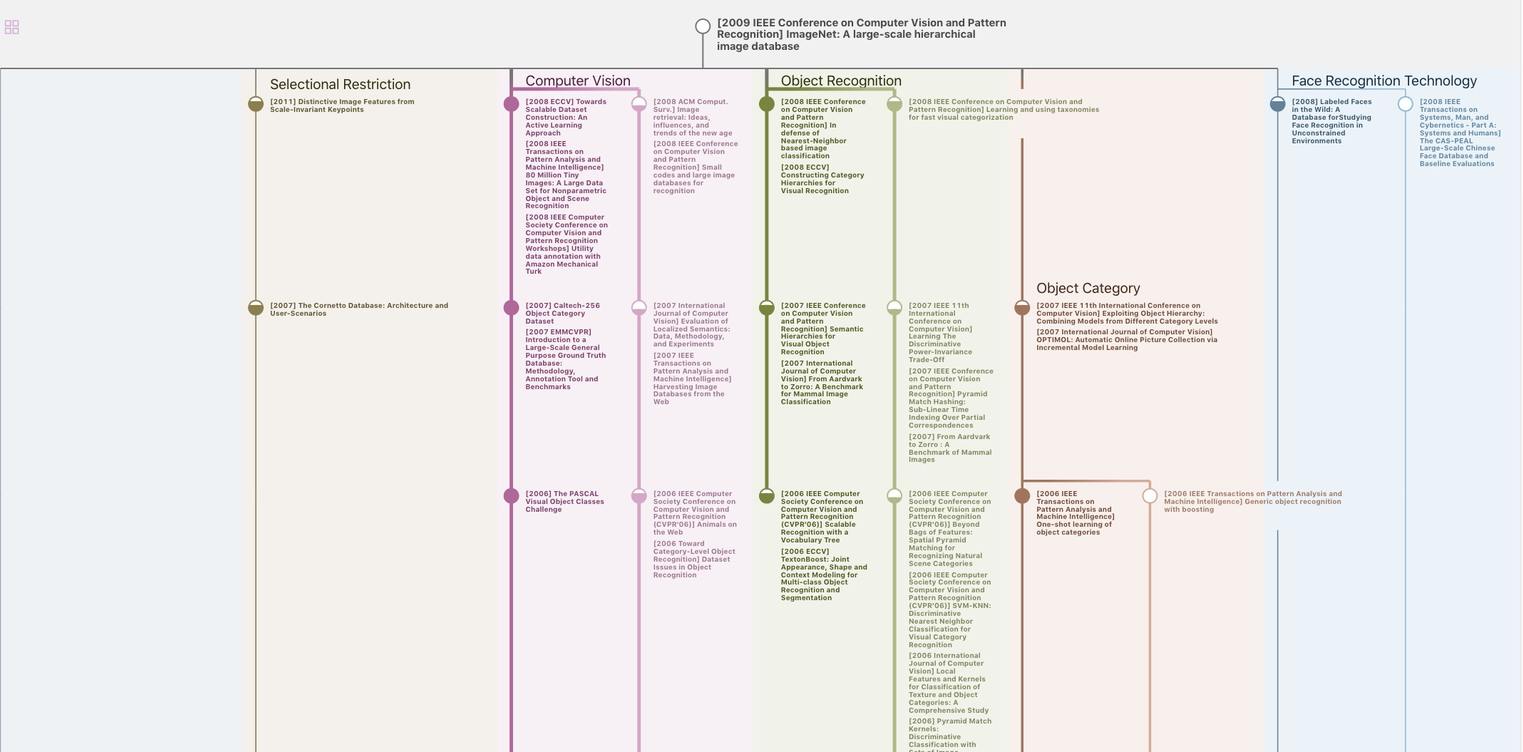
生成溯源树,研究论文发展脉络
Chat Paper
正在生成论文摘要