Making Sense of Machine Learning: A Review of Interpretation Techniques and Their Applications
APPLIED SCIENCES-BASEL(2024)
摘要
Transparency in AI models is essential for promoting human-AI collaboration and ensuring regulatory compliance. However, interpreting these models is a complex process influenced by various methods and datasets. This study presents a comprehensive overview of foundational interpretation techniques, meticulously referencing the original authors and emphasizing their pivotal contributions. Recognizing the seminal work of these pioneers is imperative for contextualizing the evolutionary trajectory of interpretation in the field of AI. Furthermore, this research offers a retrospective analysis of interpretation techniques, critically evaluating their inherent strengths and limitations. We categorize these techniques into model-based, representation-based, post hoc, and hybrid methods, delving into their diverse applications. Furthermore, we analyze publication trends over time to see how the adoption of advanced computational methods within various categories of interpretation techniques has shaped the development of AI interpretability over time. This analysis highlights a notable preference shift towards data-driven approaches in the field. Moreover, we consider crucial factors such as the suitability of these techniques for generating local or global insights and their compatibility with different data types, including images, text, and tabular data. This structured categorization serves as a guide for practitioners navigating the landscape of interpretation techniques in AI. In summary, this review not only synthesizes various interpretation techniques but also acknowledges the contributions of their original authors. By emphasizing the origins of these techniques, we aim to enhance AI model explainability and underscore the importance of recognizing biases, uncertainties, and limitations inherent in the methods and datasets. This approach promotes the ethical and practical use of interpretation insights, empowering AI practitioners, researchers, and professionals to make informed decisions when selecting techniques for responsible AI implementation in real-world scenarios.
更多查看译文
关键词
Artificial Intelligence,explainable AI,interpretable machine learning,interpretation techniques
AI 理解论文
溯源树
样例
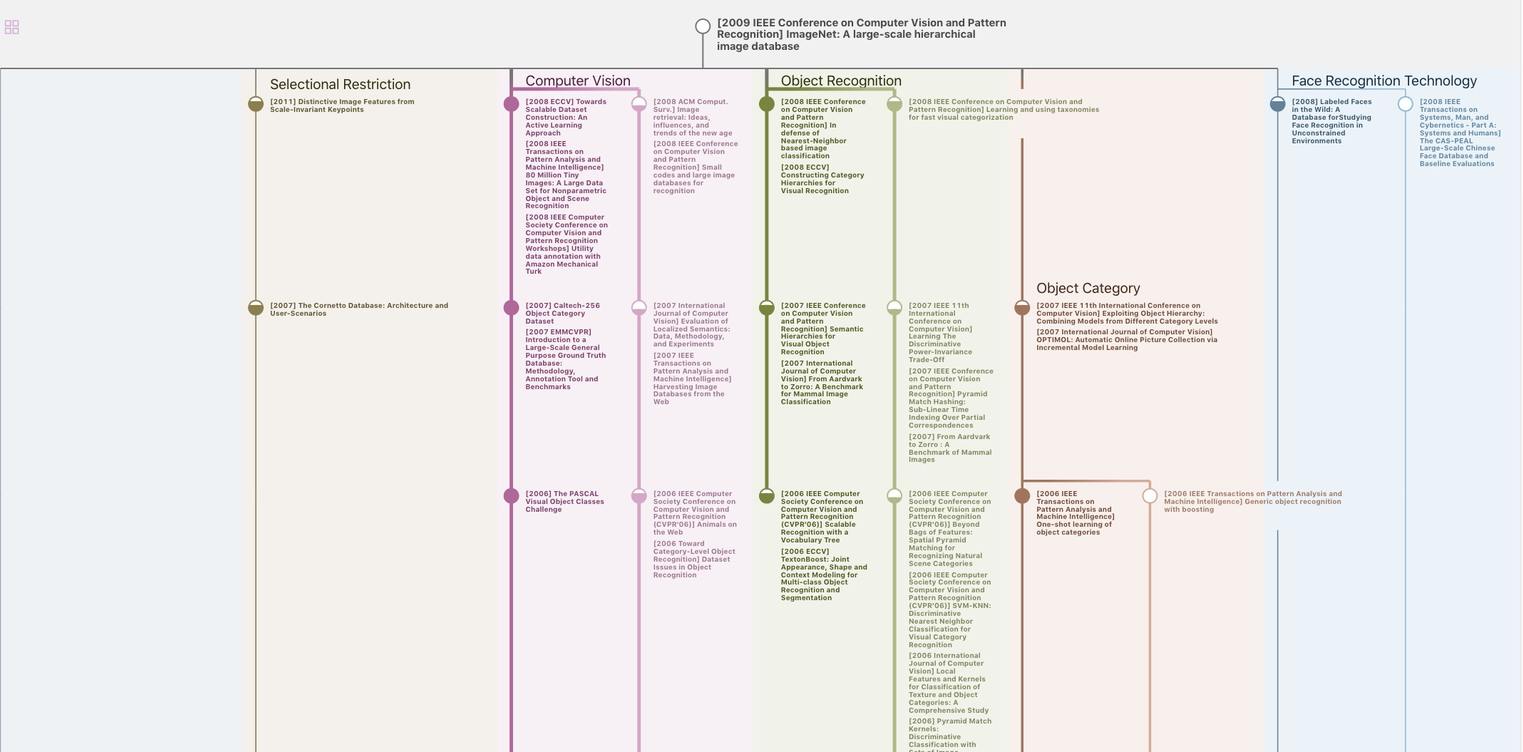
生成溯源树,研究论文发展脉络
Chat Paper
正在生成论文摘要