Differential evolution with evolutionary scale adaptation
SWARM AND EVOLUTIONARY COMPUTATION(2024)
摘要
The performance of differential evolution (DE) algorithm heavily depends on the evolutionary scale, which is controlled by the generation operations including mutation, crossover and the control parameters including mutation factor and crossover rate. Adjusting the evolutionary scale to suit different types of problems is a critical yet challenging open question in DE research. To efficiently address this issue, this paper proposes a novel DE based on evolutionary scale adaptation, termed as ESADE. First, a successful scale estimation mechanism is proposed to measure the appropriate evolutionary scale by utilizing the successful evolutionary feedback from the solution space provided by the trial vectors and the target vectors. With the appropriate evolutionary scale, an evolutionary scale adaptation mechanism pre-selects the closest or the farthest trial vector from each target vector, which corresponds to a small or large evolutionary scale respectively to match the search requirements of different evolutionary stages. The effectiveness of the ESA method is demonstrated by performance comparisons with each single baseline strategy, state-of-the-art DEs and state-of-the-art multi-strategy methods on 29 benchmark functions with three dimensionalities. Further applications on several real-world optimization problems also reveal the competitive performance of ESADE.
更多查看译文
关键词
Strategy adaptation,Multi -strategy,Mutation,Adaptive differential evolution,Global optimization
AI 理解论文
溯源树
样例
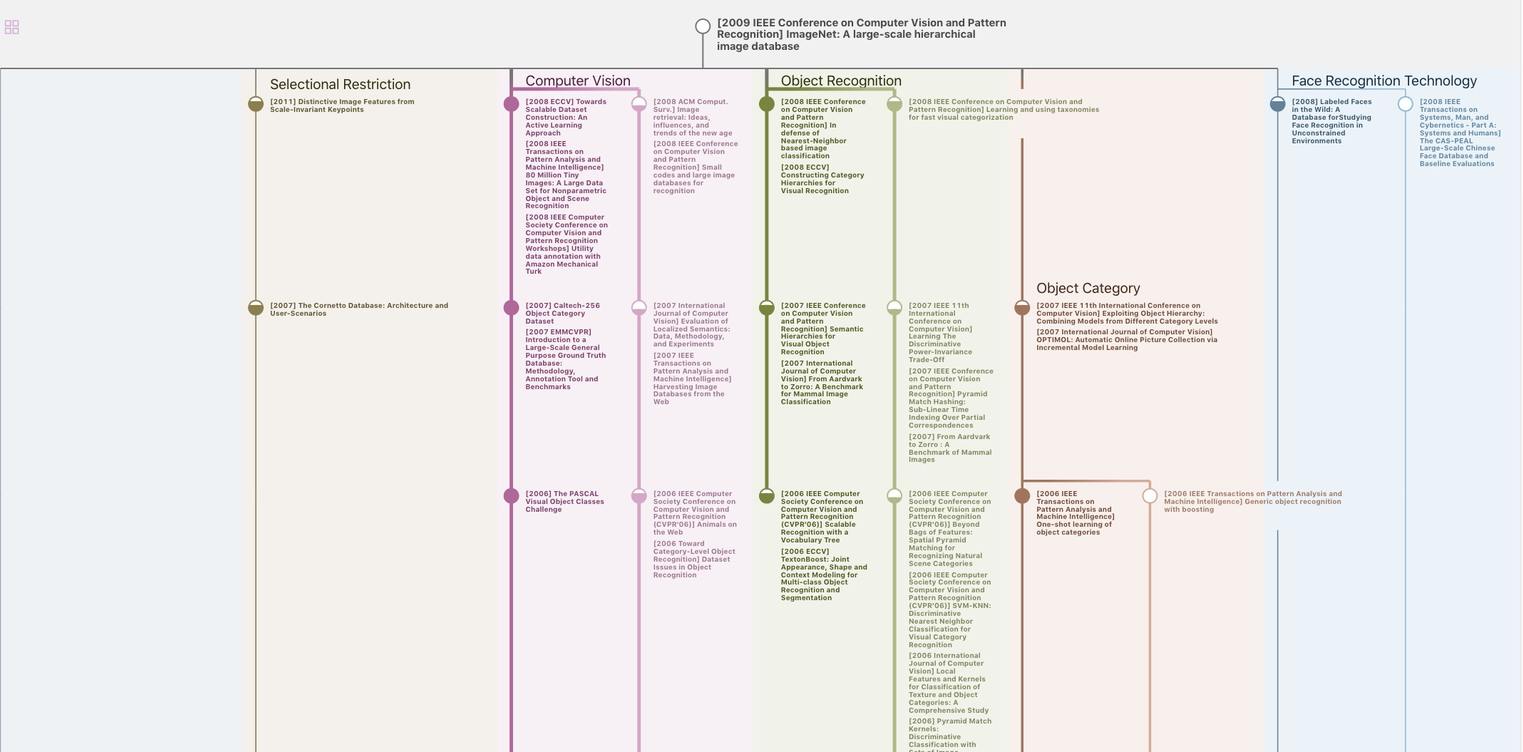
生成溯源树,研究论文发展脉络
Chat Paper
正在生成论文摘要