Tokamak plasma disruption precursor onset time study based on semi-supervised anomaly detection
Nuclear Engineering and Technology(2023)
摘要
Plasma disruption in tokamak experiments is a challenging issue that causes damage to the device. Reliable prediction methods are needed, but the lack of full understanding of plasma disruption limits the effectiveness of physics-driven methods. Data-driven methods based on supervised learning are commonly used, and they rely on labelled training data. However, manual labelling of disruption precursors is a time-consuming and challenging task, as some precursors are difficult to accurately identify. The mainstream labelling methods assume that the precursor onset occurs at a fixed time before disruption, which leads to mislabeled samples and suboptimal prediction performance. In this paper, we present disruption prediction methods based on anomaly detection to address these issues, demonstrating good prediction performance on J-TEXT and EAST. By evaluating precursor onset times using different anomaly detection algorithms, it is found that labelling methods can be improved since the onset times of different shots are not necessarily the same. The study optimizes precursor labelling using the onset times inferred by the anomaly detection predictor and test the optimized labels on supervised learning disruption predictors. The results on J-TEXT and EAST show that the models trained on the optimized labels outperform those trained on fixed onset time labels.
更多查看译文
关键词
Tokamak,Disruption prediction,Machine learning,Anomaly detection,Precursor onset time
AI 理解论文
溯源树
样例
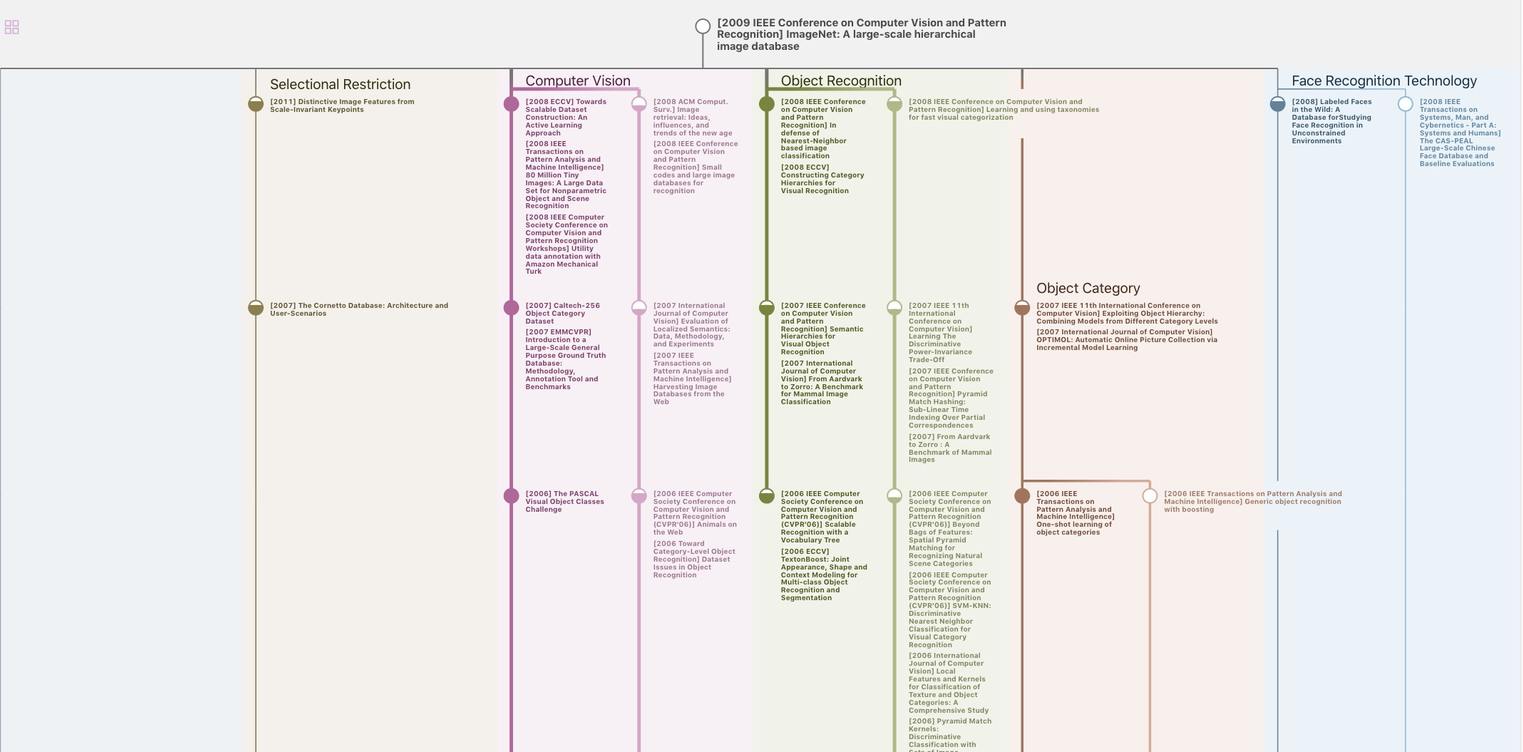
生成溯源树,研究论文发展脉络
Chat Paper
正在生成论文摘要