Deep learning methods for early detection of Alzheimer’s disease using structural MR images: A survey
Neurocomputing(2024)
摘要
In this paper, we present an extensive review of the most recent works for Alzheimer’s disease (AD) prediction, particularly Moderate Cognitive Impairment (MCI) conversion prediction. We aimed to identify the most useful brain-magnetic resonance imaging (MRI) biomarkers as well as the most successful deep learning frameworks used for prediction. To achieve this, we analysed more than 130 works and 7 review articles. A closer look revealed that the hippocampus is an important region of interest (ROI) is affected early by AD and that many related features help detect the disease in its early stages. However, considered alone, this ROI is not sufficient enough to ensure high prediction performance. Therefore, many other brain regions can also provide additional information to improve prediction accuracy. In relation to state-of-the-art deep neural networks, the U-Net represents the most efficient architecture for hippocampus segmentation. The best Dice Similarity Coefficient (DSC) value, equal to 94%, was achieved by the RESU-Net architecture. The best results for MCI conversion prediction were obtained for two models that identify significant landmarks from the whole brain for classification. The multi-stream convolutional neural network achieved the best AUC and specificity of 94.39% and 99.70%, respectively. Finally, a region ensemble model delivered the best accuracy of 85.90%, demonstrating the need for further research to address this challenging problem.
更多查看译文
关键词
Alzheimer’s disease,Structural MRI,Deep learning,Hippocampus,Progressive MCI,Stable MCI
AI 理解论文
溯源树
样例
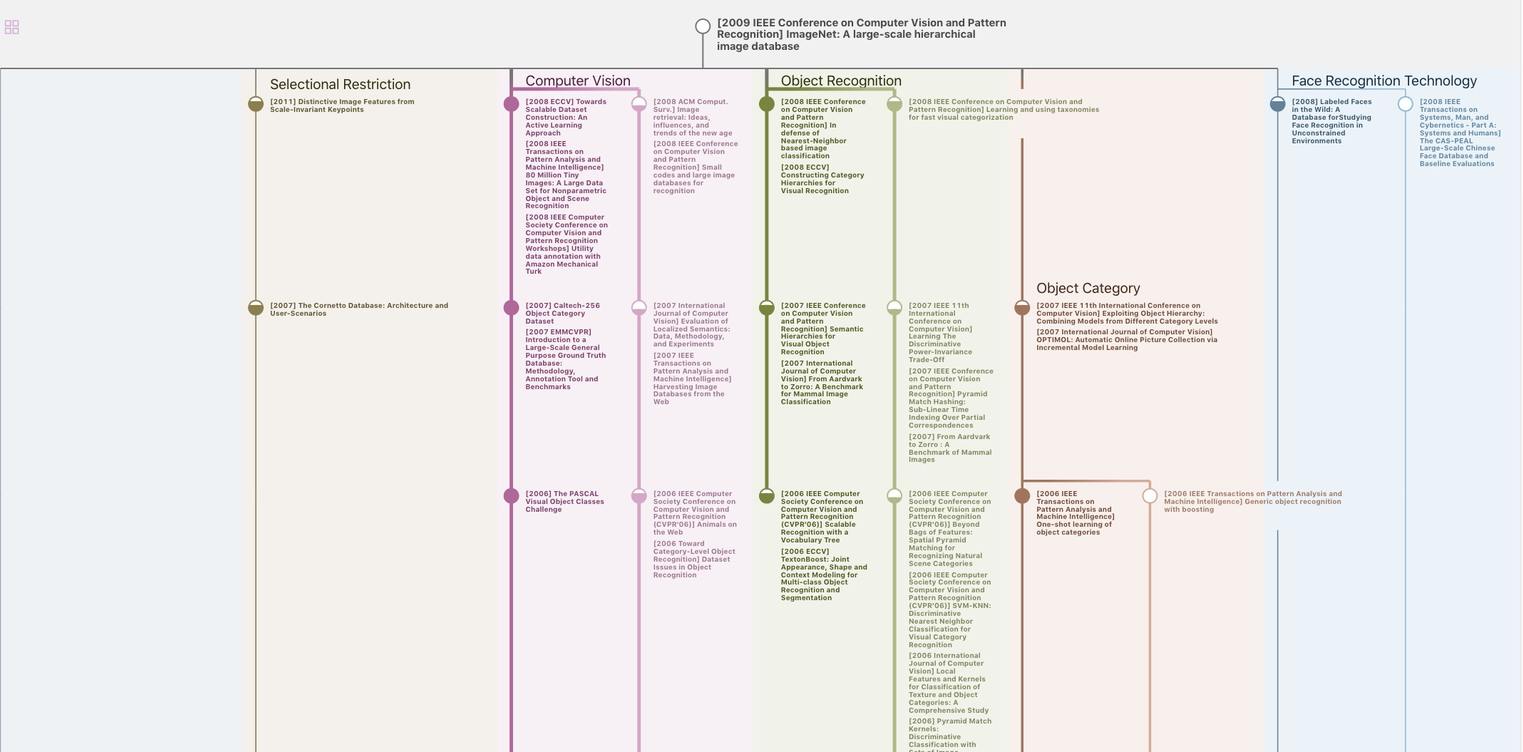
生成溯源树,研究论文发展脉络
Chat Paper
正在生成论文摘要