fastMI: A fast and consistent copula-based nonparametric estimator of mutual information
JOURNAL OF MULTIVARIATE ANALYSIS(2024)
摘要
As a fundamental concept in information theory, mutual information (??????) has been commonly applied to quantify association between random vectors. Most existing nonparametric estimators of ??????have unstable statistical performance since they involve parameter tuning. We develop a consistent and powerful estimator, called fast MI, that does not incur any parameter tuning. Based on a copula formulation, fast MI estimates ??????by leveraging Fast Fourier transform-based estimation of the underlying density. Extensive simulation studies reveal that fast MI out performs state-of-the-art estimators with improved estimation accuracy and reduced run time for large data sets. fast MI provides a powerful test for independence that exhibits satisfactory type I error control. Anticipating that it will be a powerful tool in estimating mutual information in a broad range of data, we develop an R package fast MI for broader dissemination
更多查看译文
关键词
Copula,Fast Fourier transformation,Kernel density estimation,Permutation test,Statistical dependence
AI 理解论文
溯源树
样例
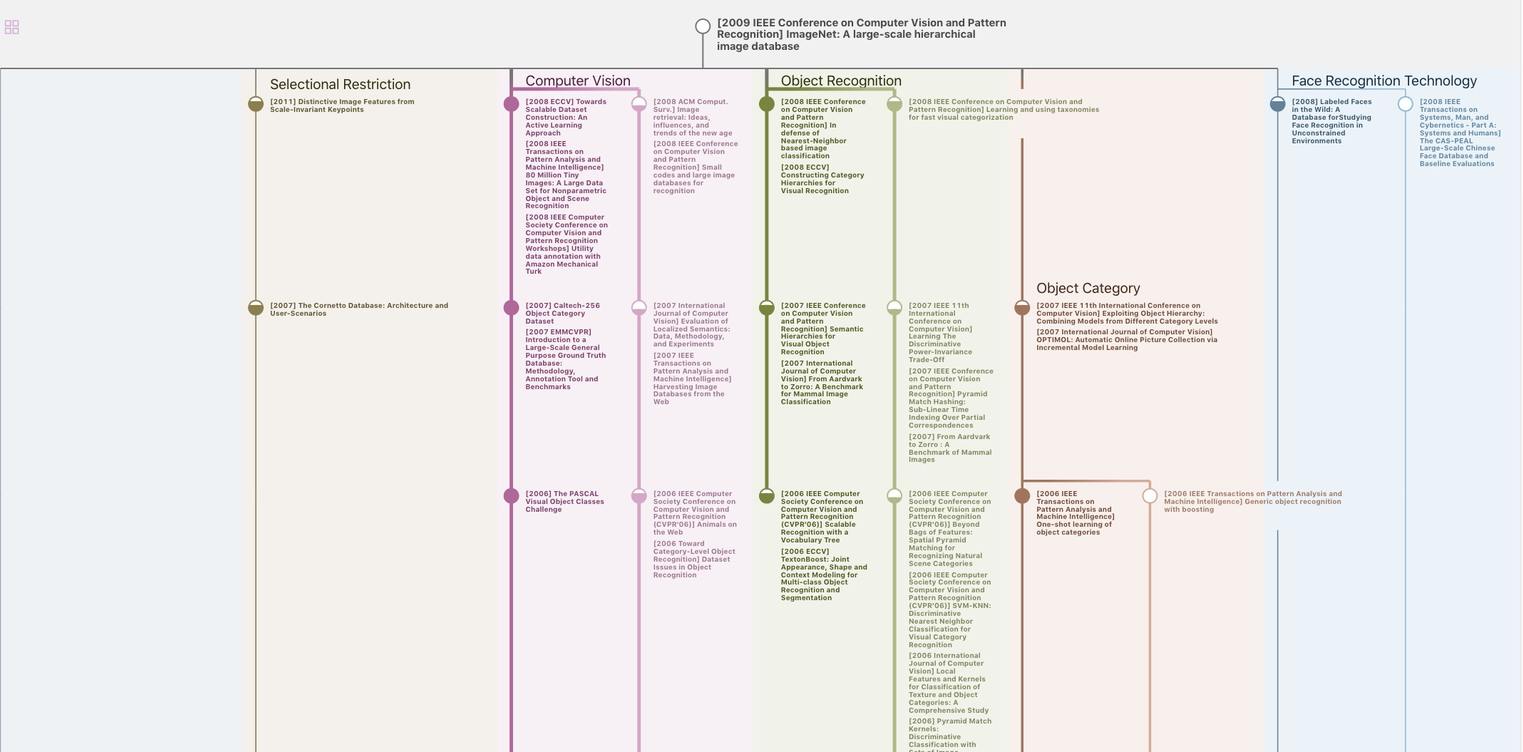
生成溯源树,研究论文发展脉络
Chat Paper
正在生成论文摘要