Integrated surrogate framework for reactive transport simulation of uranium in situ leaching with generative models
JOURNAL OF HYDROLOGY(2024)
摘要
The heterogeneity of uranium-bearing aquifers plays a crucial role in governing the migration and leaching of uranium. Uncertainty quantification (UQ) based on the Monte Carlo method is usually used to deal with the issue of aquifer heterogeneity. However, the substantial computational costs of this method arise from a large number of calls to the uranium reactive transport simulation; therefore, surrogate models are developed to reduce these costs. This study leverages a conditional deep convolutional generative adversarial network (cDC-GAN) and an adversarial autoencoder-based kriging regression (AAE-KRG) to develop an integrated surrogate framework for uranium reactive transport simulation. cDC-GAN and AAE-KRG are designed to achieve the cross-domain mapping of the permeability coefficient field to the uranium spatial distribution and leaching concentration over time, respectively. The results demonstrate a structural similarity exceeding 0.9 between the cDC-GAN and the numerical simulation, with a mean square error generally less than 0.002. These findings suggest that the cDC-GAN exhibits high accuracy and stability in terms of replacing the numerical simulation to obtain the spatial-temporal evolution of the uranium plume. Additionally, cDC-GAN is a dynamic surrogate model with the capability of interpolation because uranium spatial distributions with multiple time steps are used as training data during the training process. AAE-KRG also exhibits high accuracy and stability in terms of predicting the leaching concentration by permeability coefficient fields, as evidenced by determination coefficients generally exceeding 0.95. By comparing the computational costs, it is clear that cDC-GAN and AAE-KRG are suitable for processing spatial distribution and time series data, respectively. The trained surrogate models are used to undertake UQ of the uranium leaching area and leaching concentration. The results indicate that the uncertainty of the leaching area in the field-test model increases with time, and the increasing rate reaches a maximum in the middle period (201-300 d). The uncertainty of the leaching concentration increases with time in the early period, decreases after peaking at 137 d, and stabilizes in the middle and late periods. It is observed that the change characteristics of the uncertainty are consistent with the values of the leaching area or concentration. Furthermore, the spatial distribution pattern of uranium pollution is determined based on stochastic simulation results. The uncertainty intervals and the pollution risk status at multiple time steps are quickly obtained by surrogate models, which is helpful for scheme optimization in uranium mining. Owing to its characteristics of being datadriven and highly adaptable to nonlinear data, the integrated surrogate framework has applications beyond uranium in situ leaching.
更多查看译文
关键词
Deep learning,Uranium in situ leaching,Heterogeneity,Reactive transport simulation,Surrogate model
AI 理解论文
溯源树
样例
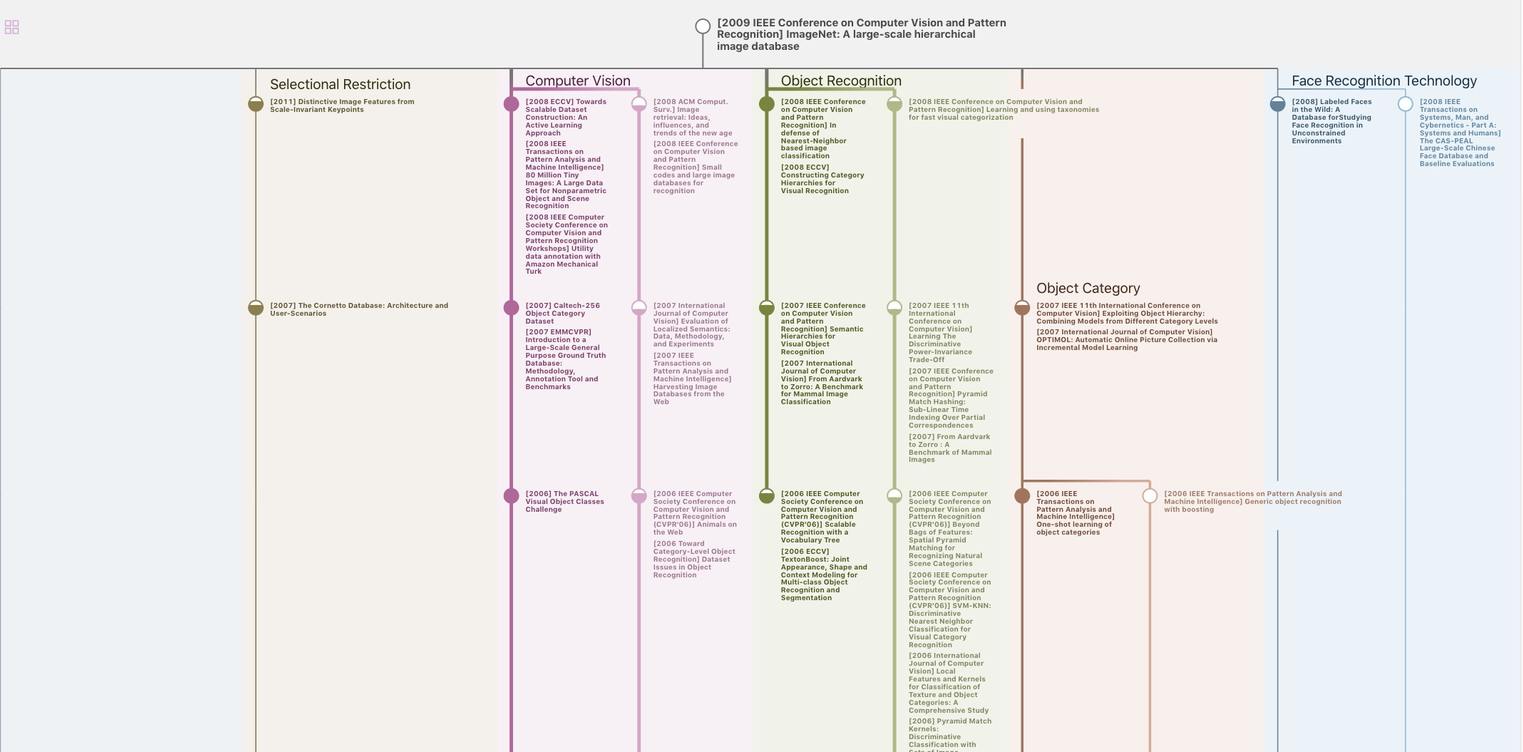
生成溯源树,研究论文发展脉络
Chat Paper
正在生成论文摘要