A Comparative Analysis of Transformer-based Protein Language Models for Remote Homology Prediction
14TH ACM CONFERENCE ON BIOINFORMATICS, COMPUTATIONAL BIOLOGY, AND HEALTH INFORMATICS, BCB 2023(2023)
摘要
Protein language models based on the transformer architecture are increasingly shown to learn rich representations from protein sequences that improve performance on a variety of downstream protein prediction tasks. These tasks encompass a wide range of predictions, including prediction of secondary structure, subcellular localization, evolutionary relationships within protein families, as well as superfamily and family membership. There is recent evidence that such models also implicitly learn structural information. In this paper we put this to the test on a hallmark problem in computational biology, remote homology prediction. We employ a rigorous setting, where, by lowering sequence identity, we clarify whether the problem of remote homology prediction has been solved. Among various interesting findings, we report that current state-of-the-art, large models are still underperforming in the "twilight zone" of very low sequence identity.
更多查看译文
关键词
remote homology,transformer,large language model
AI 理解论文
溯源树
样例
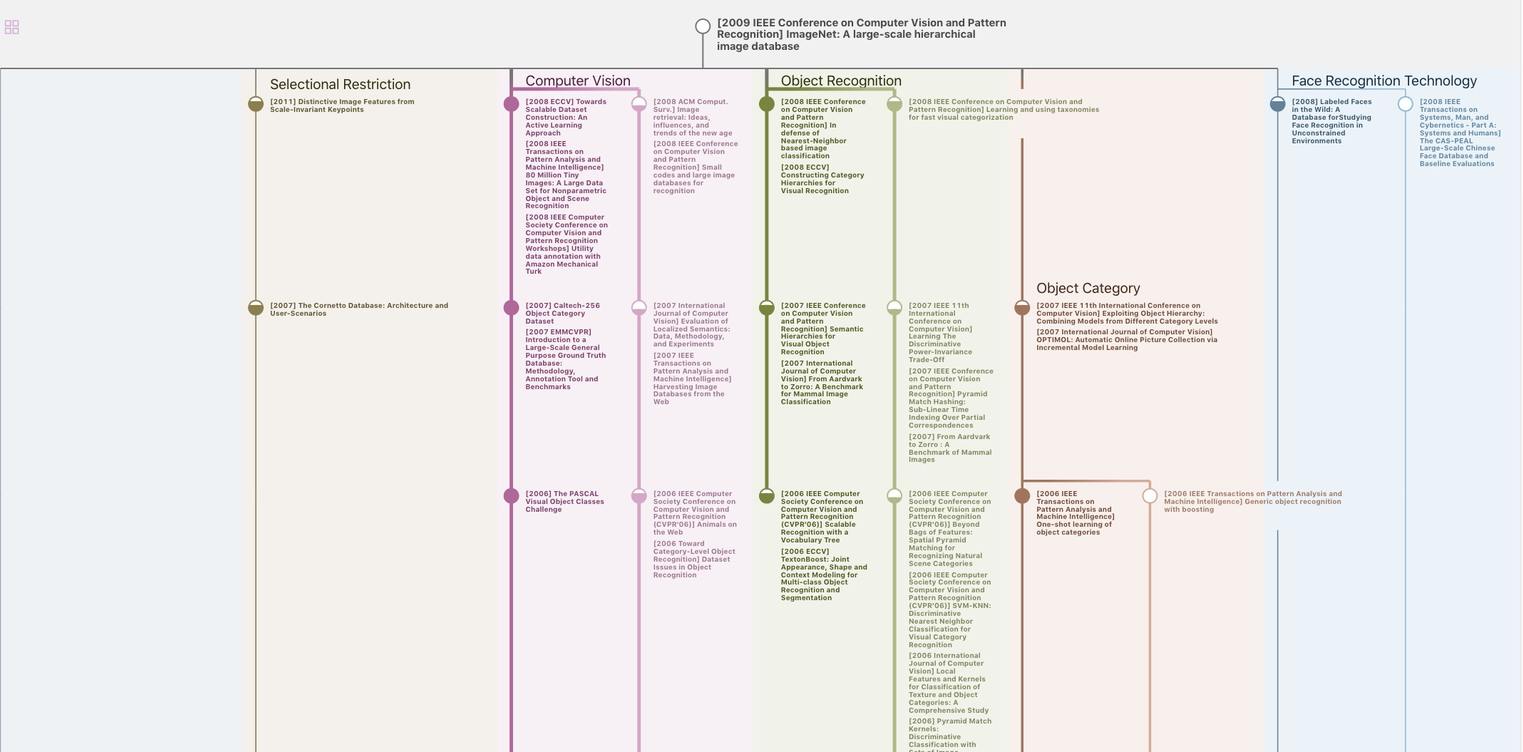
生成溯源树,研究论文发展脉络
Chat Paper
正在生成论文摘要