Research on differential pulse voltammetry detection method for low concentration glucose based on machine learning model
INTERNATIONAL JOURNAL OF ELECTROCHEMICAL SCIENCE(2024)
摘要
In the electrochemical detection process, determining the optimal experimental conditions for the standard curve often requires multiple iterations. A low-concentration glucose detection method that combines electrochemical principles with machine learning was introduced to address the challenge of determining the optimal reactant addition in electrochemical detection. We developed a model that incorporates electrochemical curve features and various experimental conditions as variables. Concentration regression analysis was conducted using five machine learning models: Multiple Linear Regression (MLR), Decision Trees (DT),Artificial Neural Networks (ANN), Random Forest (RF), and Extreme Gradient Boosting (XGBoost). The results show that the performance of the XGBoost model surpasses that of the other models. Compared to the second place-Random Forest model, it reduces the Mean Absolute Error (MAE) by 21.6 % and the Root Mean Square Error (RMSE) by 25.3 %. This method can be used for low-concentration glucose detection, addressing the challenge of determining the optimal experimental conditions. It mitigates the impact of experimental conditions on detection results, enhances concentration determination accuracy, and provides new methods for determining the optimal experimental conditions and improving detection accuracy in the field of detection and analysis.
更多查看译文
关键词
Glucose detection,Electrochemical detection of multiple,interference factors,Machine learning,XGBoost model,Artificial Neural Networks
AI 理解论文
溯源树
样例
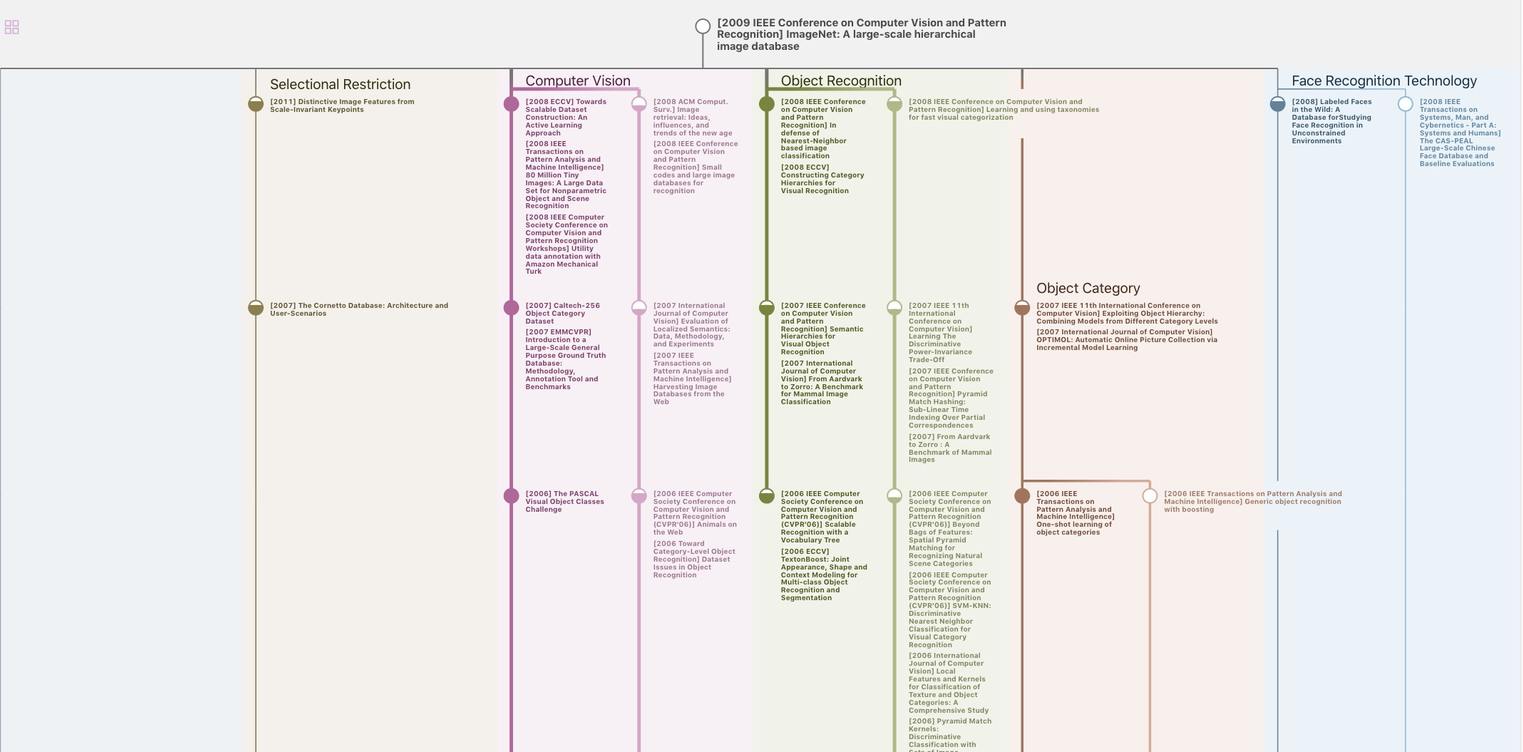
生成溯源树,研究论文发展脉络
Chat Paper
正在生成论文摘要