A two-stage CNN model for the classification and severity analysis of retinal and choroidal diseases in OCT images
International Journal of Intelligent Networks(2024)
摘要
The advancements in medical imaging techniques have brought exponential increase in the quantity and complexity of data which often require human expertise for interpretation and decision making. However, in real-world clinical settings, there is often a shortage of experts available for timely diagnosis and triage. An automated diagnostic technique aids clinicians in the precise diagnosis and effective management of diseases. In this article, a two-stage classification model using convolutional neural network (CNN) is proposed for the classification and severity analysis of retinal and choroidal diseases in optical coherence tomography (OCT) images. The proposed model can identify abnormal conditions such as Pachychoroid disorders, macular edema, and Drusen. The images are initially classified into four categories-healthy, Drusen, Pachychoroid and macular edema classes. The severity of Pachychoroid conditions, including central serous chorioretinopathy, polypoid choroidal vasculopathy, and choroidal neovascularization, is subsequently determined through a second-level classification. A modified version of the VGG16 architecture is used for the initial classification. A fine-tuned CNN with the same architecture is then employed to determine the severity of Pachychoroid diseases. Further, we make use of the UNet architecture for assessing the severity of macular edema. The proposed hybrid approach for classification and analysis achieved promising results, demonstrating consistency on par with human experts in diagnosing and grading ocular diseases.
更多查看译文
关键词
Pachychoroid,OCT classification,Edema segmentation
AI 理解论文
溯源树
样例
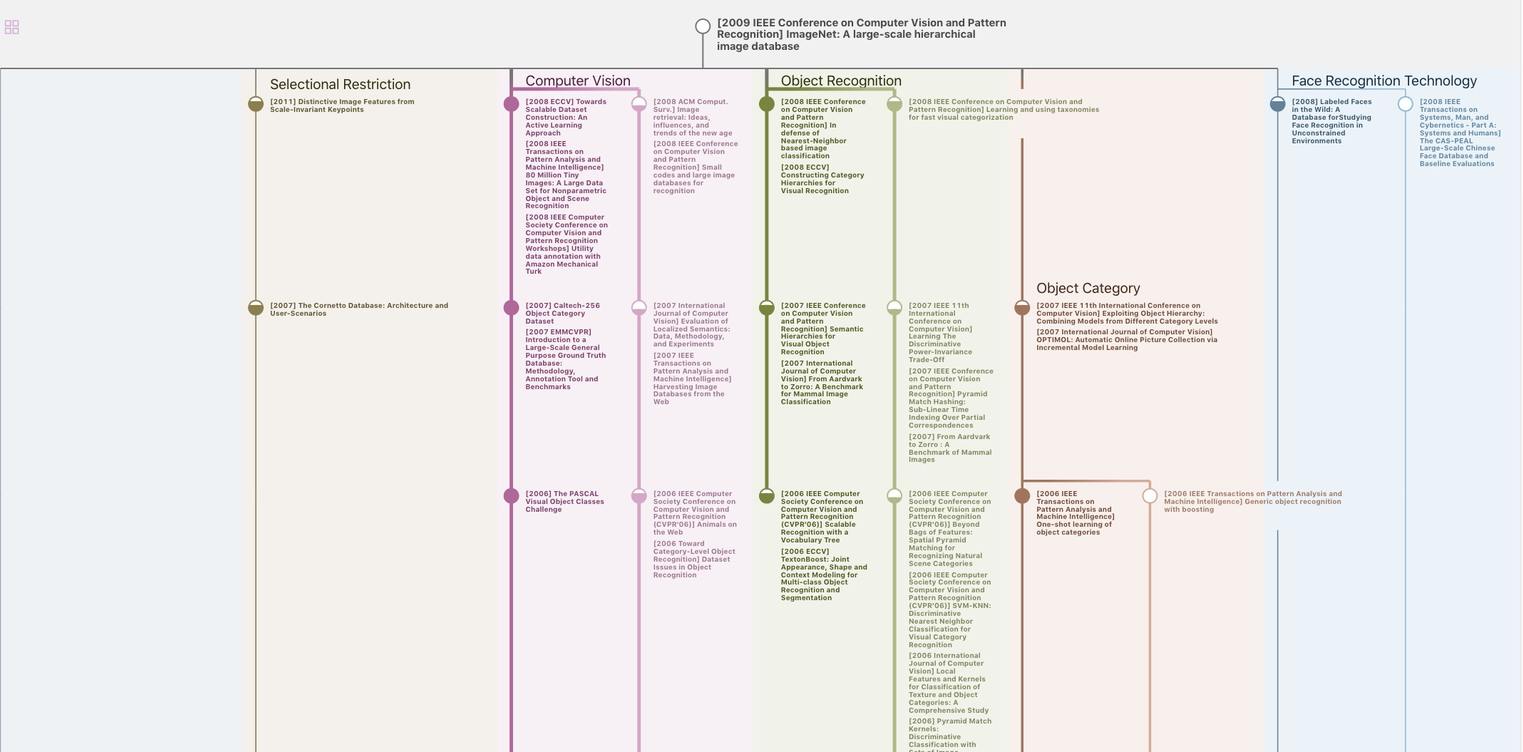
生成溯源树,研究论文发展脉络
Chat Paper
正在生成论文摘要