Lightweight cross-guided contextual perceptive network for visible-infrared urban road scene parsing
INFRARED PHYSICS & TECHNOLOGY(2024)
摘要
Visible-infrared urban road scene parsing is attracting increasing attention because it can extract complementary cues from the visible and infrared imaging modalities. However, most existing parsing methods adopt complicated models, which incur large computational costs and limit real-time performance. Moreover, parsing methods may inadequately explore and apply high-level semantic information, considerably undermining the parsing accuracy. To solve these problems, we introduce a lightweight high-performance network called crossguided contextual perceptive network (CCPNet). A lightweight backbone equipped with adaptive refined fusion modules reduces the size of CCPNet. Additionally, a cross-guided contextual perceptive module extracts and enhances semantic cues from high-level features. Experimental results indicate that CCPNet achieves stateof-the-art performance for visible-infrared scene parsing with few parameters (7.34 million), a small model (29.9 MB), and real-time inference (50.03 fps). The CCPNet code and results are available at: https://github. com/Jinfu0913/CCPNet.
更多查看译文
关键词
Visible-infrared Scene parsing,Lightweight network,Cross-guided contextual perception,Adaptive refined fusion
AI 理解论文
溯源树
样例
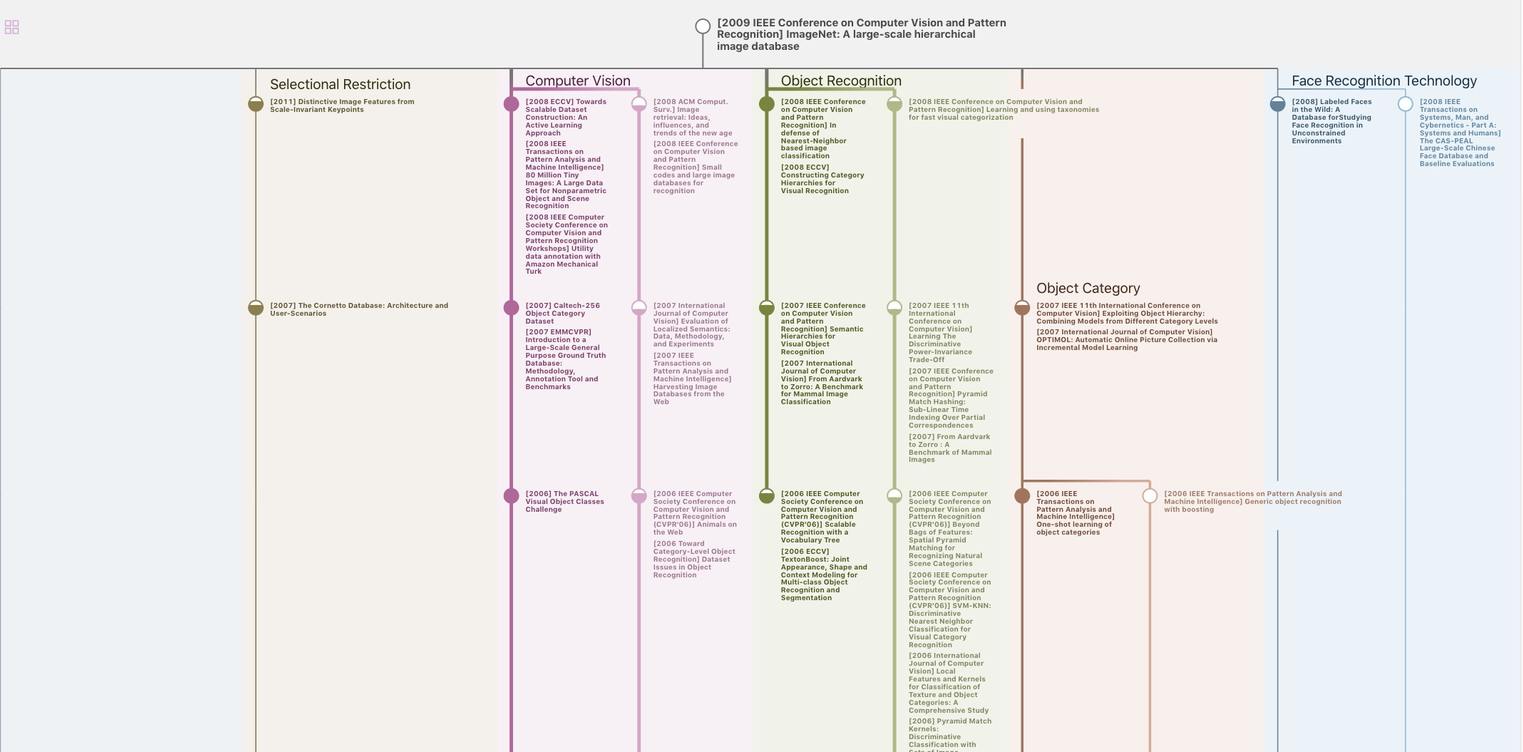
生成溯源树,研究论文发展脉络
Chat Paper
正在生成论文摘要