A DDPG-based energy efficient federated learning algorithm with SWIPT and MC-NOMA
ICT Express(2023)
摘要
Federated learning (FL) has emerged as a promising distributed machine learning technique. It has the potential to play a key role in future Internet of Things (IoT) networks by ensuring the security and privacy of user data combined with efficient utilization of communication resources. This paper addresses the challenge of maximizing energy efficiency in FL systems. We employed simultaneous wireless information and power transfer (SWIPT) and multi-carrier non-orthogonal multiple access (MC-NOMA) techniques. Also, we jointly optimized power allocation and central processing unit (CPU) resource allocation to minimize latency-constrained energy consumption. We formulated an optimization problem using a Markov decision process (MDP) and utilized a deep deterministic policy gradient (DDPG) reinforcement learning algorithm to solve our MDP problem. We tested the proposed algorithm through extensive simulations and confirmed it converges in a stable manner and provides enhanced energy efficiency compared to conventional schemes.
更多查看译文
关键词
Deep reinforcement learning,Federated learning,Multi-carrier non-orthogonal multiple access,SWIPT
AI 理解论文
溯源树
样例
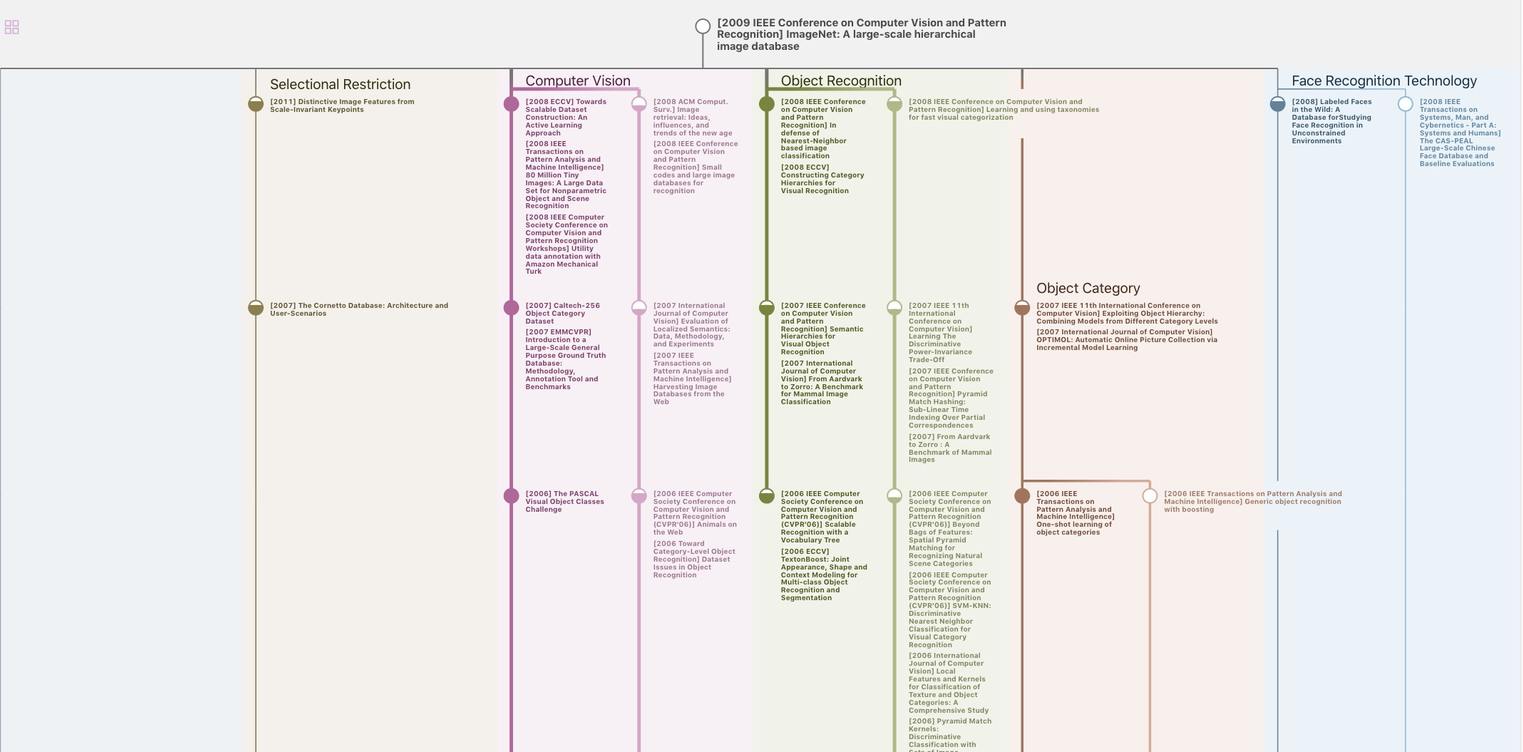
生成溯源树,研究论文发展脉络
Chat Paper
正在生成论文摘要