Speaker voice normalization for end-to-end speech translation
Expert Systems with Applications(2024)
摘要
Speaker voices exhibit acoustic variation. Our preliminary experiments reveal that normalized voice can significantly improve end-to-end speech translation. To mitigate the negative impact of acoustic voice variation across speakers on speech translation, we propose SVN-ST, a Speaker-Voice-Normalized end-to-end Speech Translation framework. In SVN-ST, we use synthetic speech inputs generated from a Text-to-Speech system to complement raw speech inputs. In order to explore synthetic speech inputs, we introduce two essential components for SVN-ST: an alignment adapter at the encoder side and a normalized speech knowledge distillation module at the decoder side. The former forces the representations of raw speech inputs to be close to those of synthetic (normalized) speech inputs while the latter attempts to guide the translations of raw speech inputs with those yielded from synthetic speech inputs. Two additional losses are also defined to equip with the two components. Experimental results on the MuST-C benchmark dataset demonstrate that SVN-ST outperforms previous state-of-the-art end-to-end non-normalized speech translation systems by 0.4 BLEU and cascaded speech translation systems by 2.3 BLEU. On the Covost 2 testset, SVN-ST also outperforms other normalized speech methods on robustness. Further analyses suggest that our model effectively aligns speech representations from different speakers, enhances robustness, and significantly improves sentence-level translation quality.
更多查看译文
关键词
Machine translation,Speech translation,Speaker normalization
AI 理解论文
溯源树
样例
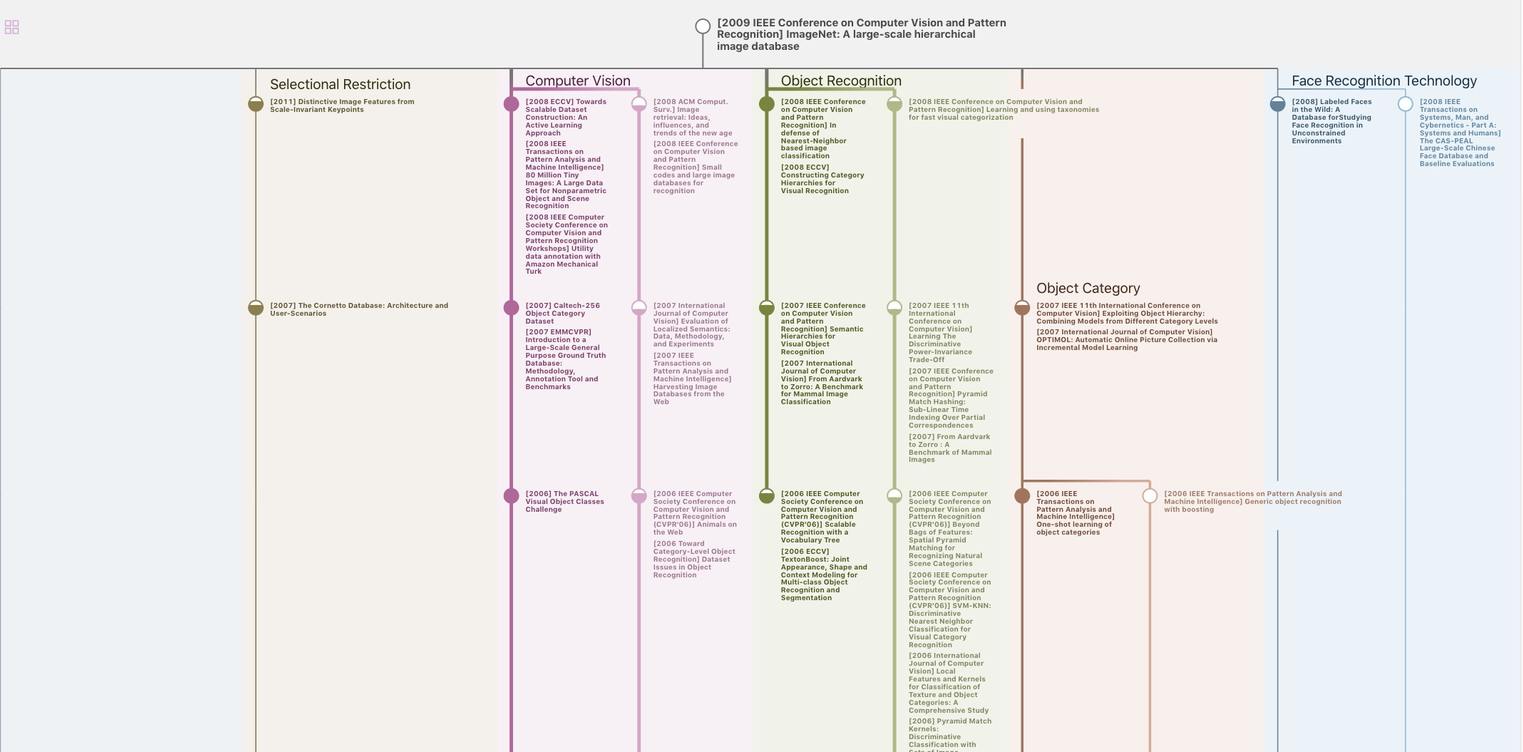
生成溯源树,研究论文发展脉络
Chat Paper
正在生成论文摘要