QGFormer: Queries-guided transformer for flexible medical image synthesis with domain missing
EXPERT SYSTEMS WITH APPLICATIONS(2024)
摘要
Domain missing poses a common challenge in medical clinical practice, limiting diagnostic accuracy compared to the complete multi -domain images that provide complementary information. We propose QGFormer to address this issue by flexibly imputing missing domains from any available source domain using a single model, which is challenging due to (1) the inherent limitation of CNNs to capture long-range dependencies, (2) the difficulty in modeling the interand intra-domain dependencies of multi -domain images, and (3) inefficiencies in fusing domain -specific features associated with missing domains. To tackle these challenges, we introduce two spatial-domanial attentions (SDAs), which establish intra-domain (spatial dimension) and interdomain (domain dimension) dependencies independently or jointly. QGFormer, constructed based on SDAs, comprises three components: Encoder, Decoder and Fusion. The Encoder and Decoder form the backbone, modeling contextual dependencies to create a hierarchical representation of features. The QGFormer Fusion then adaptively aggregates these representations to synthesize specific missing domains from coarse to fine, guided by learnable domain queries. This process is interpretable because the attention scores in Fusion indicate how much attention the target domains pay to different inputs and regions. In addition, the scalable architecture enables QGFormer to segment tumors with domain missing by replacing domain queries with segment queries. Extensive experiments demonstrate that our approach achieves consistent improvements in multi -domain imputation, cross -domain image translation and multitask of synthesis and segmentation.
更多查看译文
关键词
Medical image synthesis,Multi-domain imputation,Adaptive fusion,Attention,Segmentation
AI 理解论文
溯源树
样例
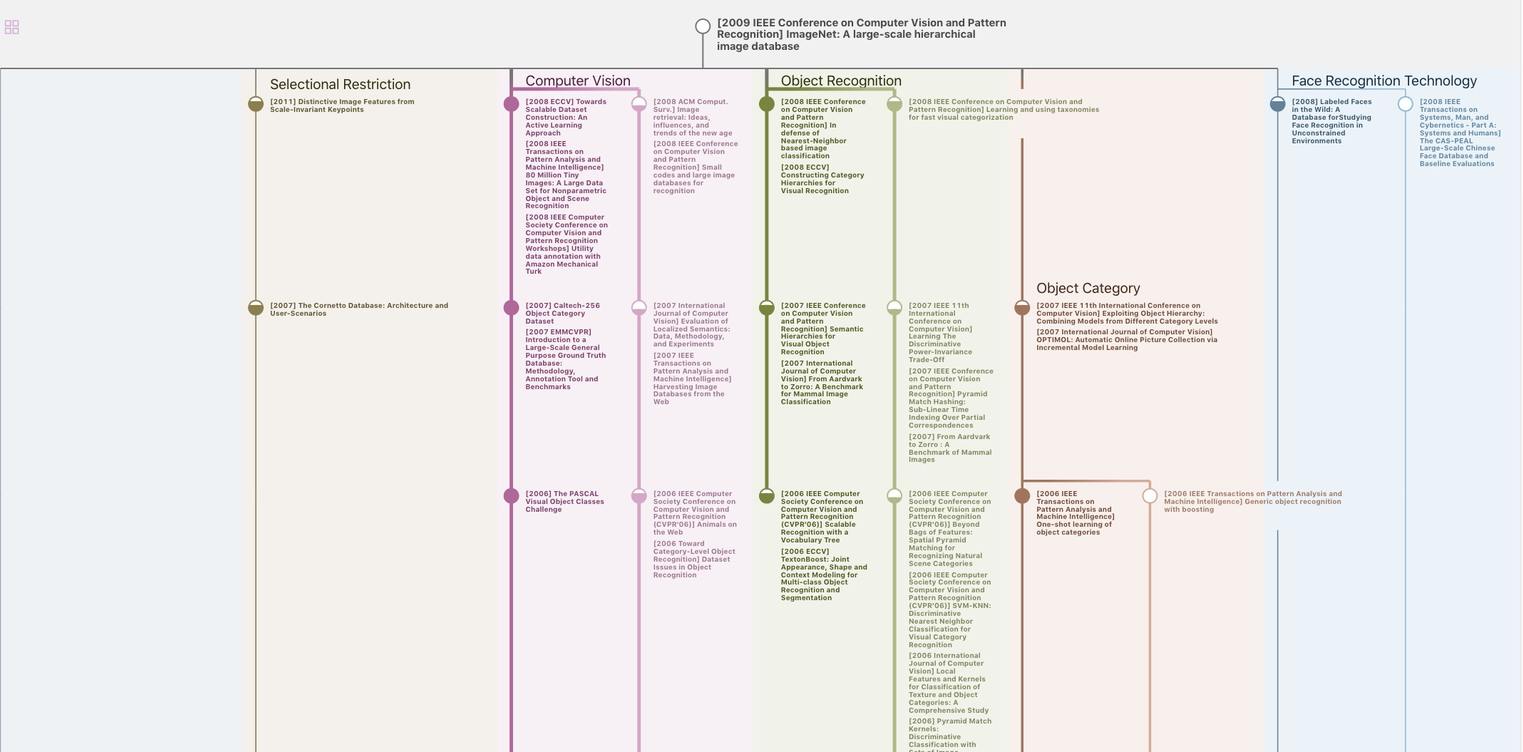
生成溯源树,研究论文发展脉络
Chat Paper
正在生成论文摘要