Regularizing cross-attention learning for end-to-end speech translation with ASR and MT attention matrices
Expert Systems with Applications(2024)
摘要
The cross-attention mechanism enables Transformer to capture correspondences between the input and output. However, in the domain of end-to-end (E2E) speech-to-text translation (ST), the learned cross-attention weights often struggle to accurately correspond with actual alignments, given the need to align speech and text across different modalities and languages. In this paper, we present a simple yet effective method called regularized cross-attention learning, for end-to-end speech translation in a multitask learning (MTL) framework. RCAL leverages the knowledge from auxiliary automatic speech recognition (ASR) and machine translation (MT) tasks to generate a teacher cross-attention matrix, serving as prior alignment knowledge to enhance cross-attention learning within the ST task. An additional loss function is introduced as part of the MTL framework to facilitate this process. We conducted experiments on the MuST-C benchmark dataset to evaluate the effectiveness of RCAL. The results demonstrate that the proposed approach yields significant improvements over the baseline, with an average enhancement of +0.8 BLEU across four translation directions in two experimental settings, outperforming state-of-the-art E2E and cascaded speech translation models. Further analysis and visualization reveal that the model with RCAL effectively learns high-quality alignment information from auxiliary ASR and ST tasks, thereby improving the ST alignment quality. Moreover, the experiments with different sizes of MT and ST data provide strong evidence supporting our model’s robustness in various scenarios.
更多查看译文
关键词
End-to-end speech-to-text translation,Transformer,Multitask learning,Cross-attention learning
AI 理解论文
溯源树
样例
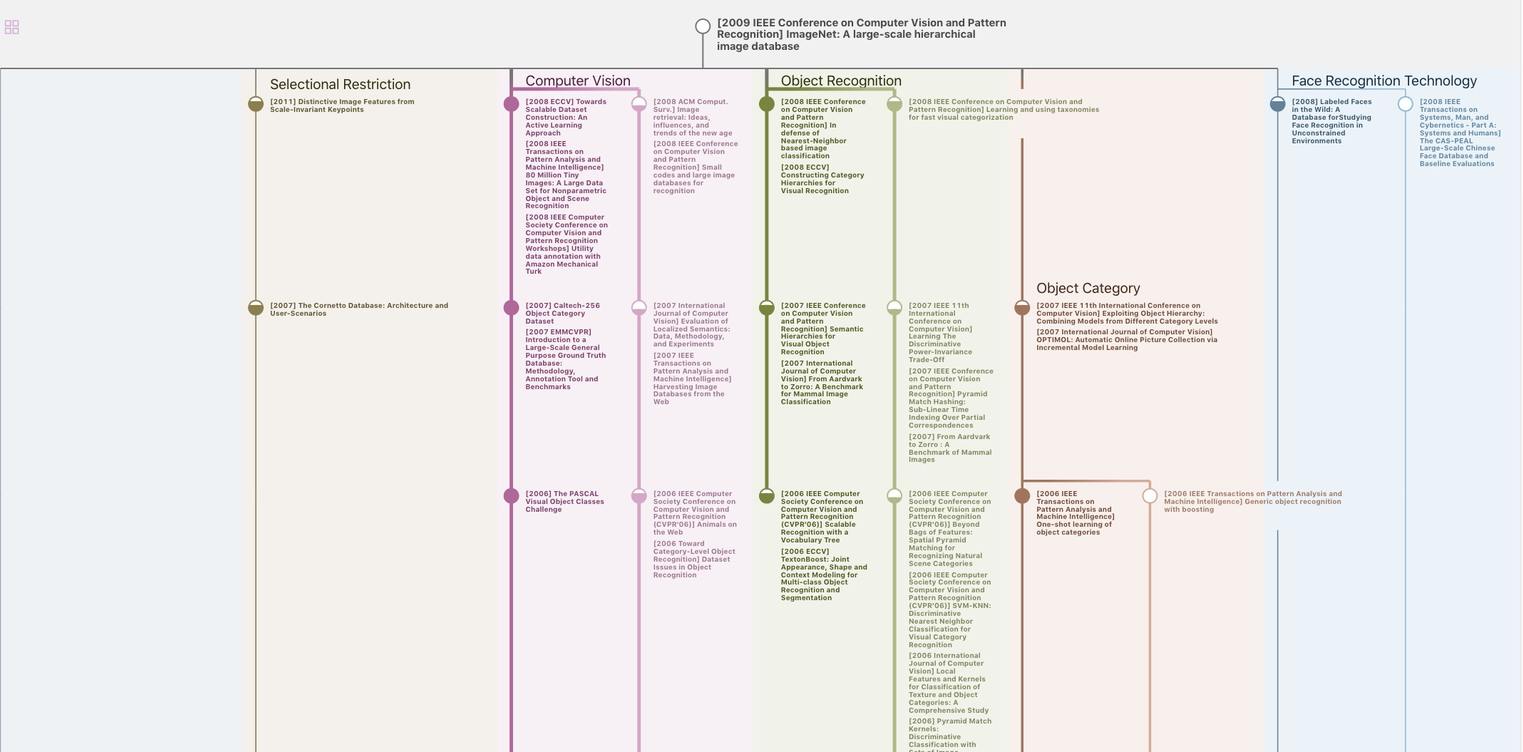
生成溯源树,研究论文发展脉络
Chat Paper
正在生成论文摘要