Accelerated exploration of heterogeneous CO2 hydrogenation catalysts by Bayesian-optimized high-throughput and automated experimentation
CHEM CATALYSIS(2024)
摘要
A closed -loop data -driven approach was used to optimize catalyst compositions for the direct transformation of carbon dioxide (CO2) into methanol by combining Bayesian optimization (BO), automated synthesis, and high -throughput catalytic performance evaluation in fixedbed reactors. The BO algorithm optimized a four -objective function simultaneously considering 8 experimental variables. In 6 weeks, 144 catalysts over 6 generations were synthesized and tested with limited manual laboratory activity. Between the first and fifth catalyst generation, the average CO2 conversion and methanol formation rates have been multiplied by 5.7 and 12.6, respectively, while simultaneously dividing the methane production rate and cost by 3.2 and 6.3, respectively. The best catalyst of the study shows an optimized composition of 1.85 wt % Cu, 0.69 wt % Zn, and 0.05 wt % Ce supported on ZrO2. Notably, the same dataset could also be reused to optimize the process toward different objectives and enable the identification of other catalyst compositions.
更多查看译文
关键词
heterogeneous catalysis,CO2,methanol,artificial intelligence,machine learning,Bayesian optimization,high-throughput experimentation,automation,robotics,self-driving labs
AI 理解论文
溯源树
样例
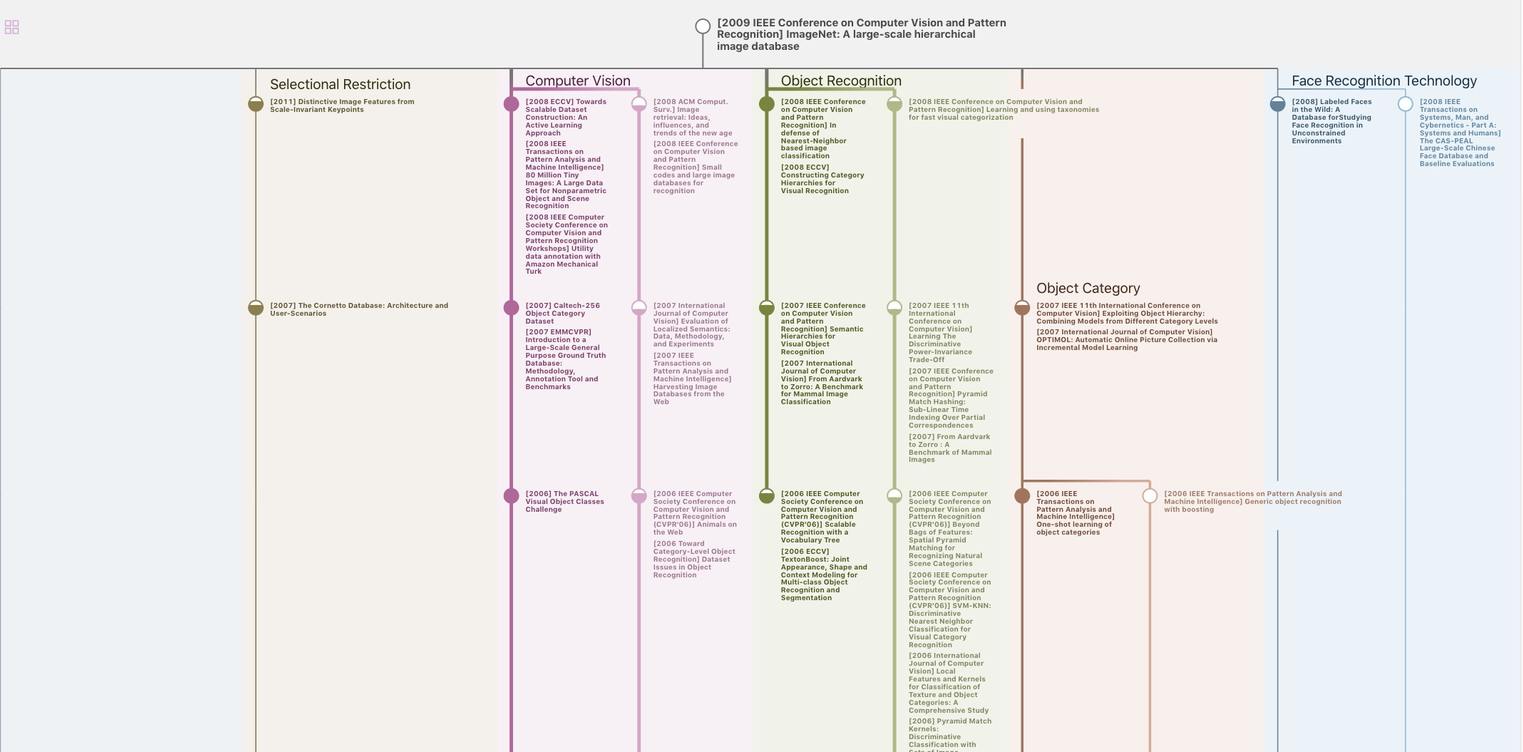
生成溯源树,研究论文发展脉络
Chat Paper
正在生成论文摘要