Reinforcement Learning-Based Cutting Parameter Dynamic Decision Method Considering Tool Wear for a Turning Machining Process
International Journal of Precision Engineering and Manufacturing-Green Technology(2024)
摘要
Cutting parameter optimization is considered as an effective way for energy consumption saving. In the machining process, the tool wear of cutting tools varies with the rise of the number of workpieces, which has a significant effect on cutting parameters decisions. However, most of existing approaches are conducted for a single workpiece, and cannot select the optimal cutting parameters based on the dynamic changes in tool wear. To this end, a reinforcement learning-based cutting parameter dynamic decision (RLCPDD) method is developed for each workpiece adaptive to the change of tool wear. Specifically, the correlation between the energy consumption, cutting parameters, and tool wear is analyzed, and a multi-objective optimization model considering tool wear is formulated. A Markov Decision Process (MDP) can be used for designing the decision-making of cutting parameters for the machining process. The developed RLCPDD is validated by comparative case study. The case study indicates that: (1) the different cutting parameters can be determined for the different tool wear of cutting tool, and (2) the dynamic decision of cutting parameters based on tool wear can further reduce energy consumption, production time, and production cost by 3.87%, 6.36%, and 6.83% compared with the PSO algorithm.
更多查看译文
关键词
Energy-efficient,Cutting parameter optimization,Tool wear,Machining process
AI 理解论文
溯源树
样例
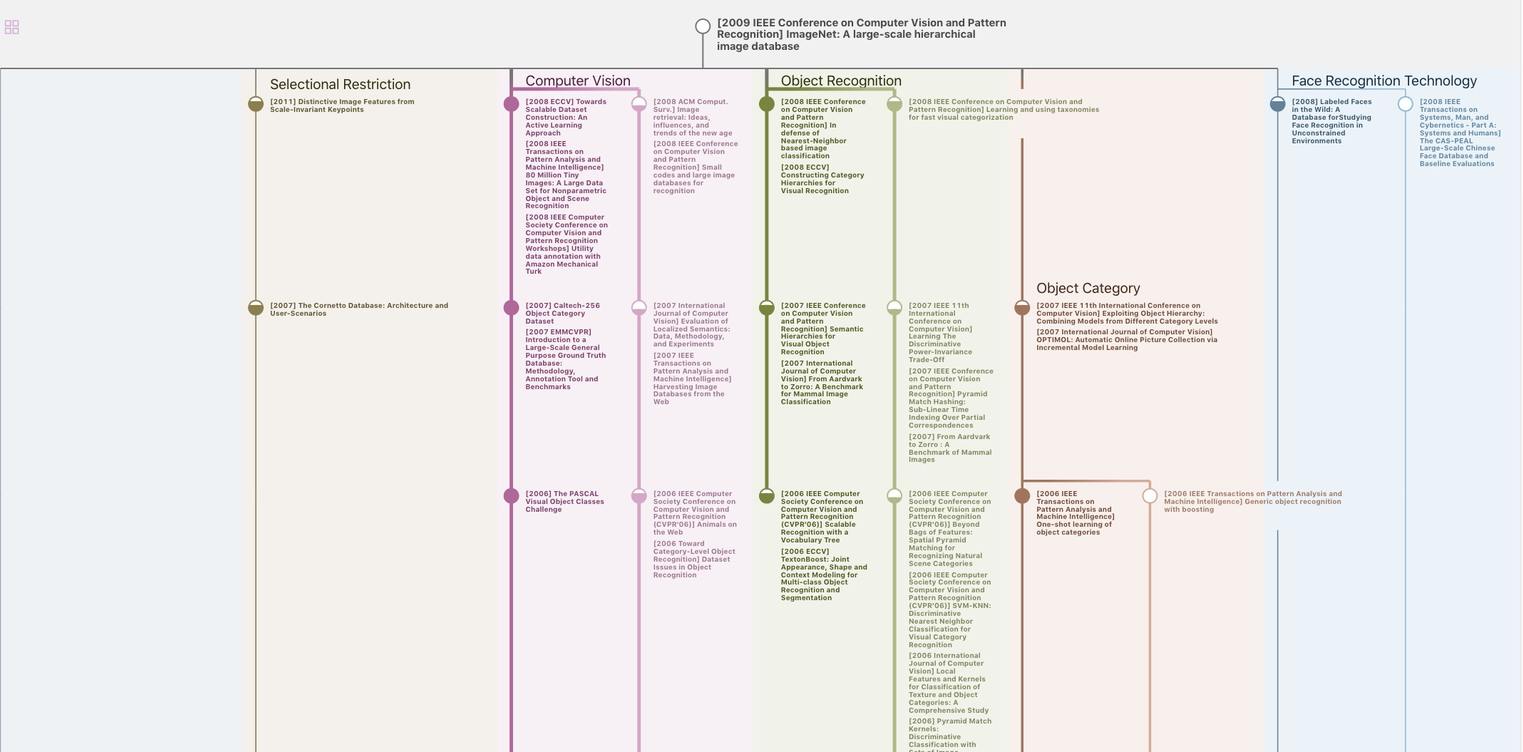
生成溯源树,研究论文发展脉络
Chat Paper
正在生成论文摘要