Mapping the Design Space of Teachable Social Media Feed Experiences
CoRR(2024)
摘要
Social media feeds are deeply personal spaces that reflect individual values
and preferences. However, top-down, platform-wide content algorithms can reduce
users' sense of agency and fail to account for nuanced experiences and values.
Drawing on the paradigm of interactive machine teaching (IMT), an interaction
framework for non-expert algorithmic adaptation, we map out a design space for
teachable social media feed experiences to empower agential, personalized feed
curation. To do so, we conducted a think-aloud study (N=24) featuring four
social media platforms – Instagram, Mastodon, TikTok, and Twitter – to
understand key signals users leveraged to determine the value of a post in
their feed. We synthesized users' signals into taxonomies that, when combined
with user interviews, inform five design principles that extend IMT into the
social media setting. We finally embodied our principles into three feed
designs that we present as sensitizing concepts for teachable feed experiences
moving forward.
更多查看译文
AI 理解论文
溯源树
样例
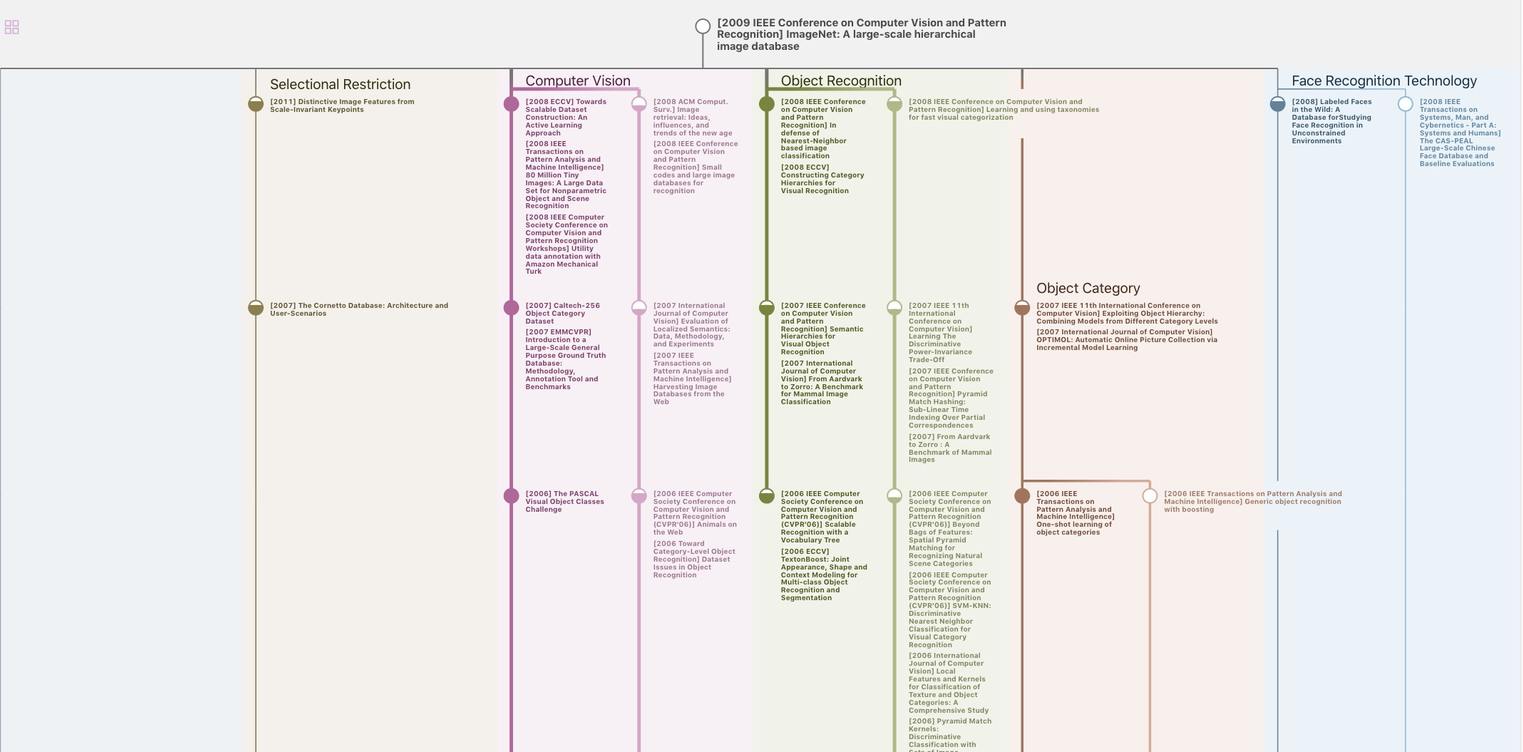
生成溯源树,研究论文发展脉络
Chat Paper
正在生成论文摘要