Tight Competitive and Variance Analyses of Matching Policies in Gig Platforms
CoRR(2024)
摘要
In this paper, we propose an online-matching-based model to tackle the two
fundamental issues, matching and pricing, existing in a wide range of
real-world gig platforms, including ride-hailing (matching riders and drivers),
crowdsourcing markets (pairing workers and tasks), and online recommendations
(offering items to customers). Our model assumes the arriving distributions of
dynamic agents (e.g., riders, workers, and buyers) are accessible in advance,
and they can change over time, which is referred to as Known
Heterogeneous Distributions (KHD).
In this paper, we initiate variance analysis for online matching algorithms
under KHD. Unlike the popular competitive-ratio (CR) metric, the variance of
online algorithms' performance is rarely studied due to inherent technical
challenges, though it is well linked to robustness. We focus on two natural
parameterized sampling policies, denoted by 𝖠𝖳𝖳(γ) and
𝖲𝖠𝖬𝖯(γ), which appear as foundational bedrock in online
algorithm design. We offer rigorous competitive ratio (CR) and variance
analyses for both policies. Specifically, we show that 𝖠𝖳𝖳(γ)
with γ∈ [0,1/2] achieves a CR of γ and a variance of γ· (1-γ) · B on the total number of matches with B being the
total matching capacity. In contrast, 𝖲𝖠𝖬𝖯(γ) with γ∈
[0,1] accomplishes a CR of γ (1-γ) and a variance of γ̅
(1-γ̅)· B with γ̅=min(γ,1/2). All CR and
variance analyses are tight and unconditional of any benchmark. As a byproduct,
we prove that 𝖠𝖳𝖳(γ=1/2) achieves an optimal CR of 1/2.
更多查看译文
AI 理解论文
溯源树
样例
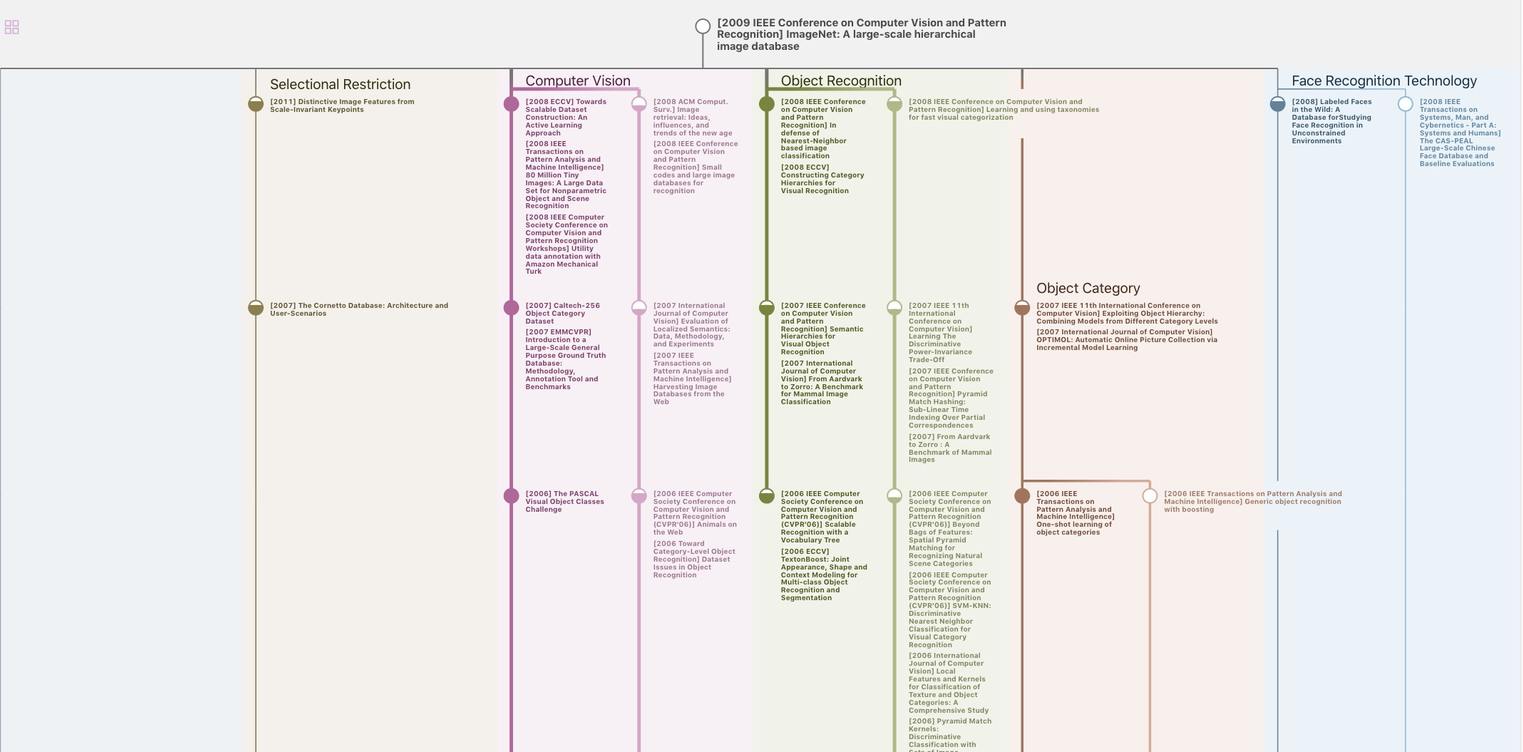
生成溯源树,研究论文发展脉络
Chat Paper
正在生成论文摘要