Semantic-aware Transformer for shadow detection
COMPUTER VISION AND IMAGE UNDERSTANDING(2024)
摘要
Shadow detection is significant for scene understanding. Ambiguities in a shadow image, such as shadow-like non-shadow regions and shadow regions with non-shadow patterns, are still very challenging for prevalent CNN-based methods. This work attempts to alleviate this problem from a new perspective of shape semantics, and then proposes a Semantic-aware Transformer (SaT) in a multi-task learning manner. Concretely, we first propose a shadow detection network based on the recent progress of Transformer architecture, allowing us to capture significant global interactions between contexts. Next, we design a multi-task learning framework, combining shadow supervision and semantic supervision to perform a semantic-aware shadow detection. Finally, we introduce a simple yet effective information buffer unit to overcome the gradient signal conflict from multi-task learning. Experimental results on three public benchmark datasets (i.e., ISTD, SBU, and UCF) show that our SaT can effectively detect ambiguous cases and achieve state-of-the-art results.
更多查看译文
关键词
Image processing,Shadow detection,Transformer,Semantic-aware,Multi-task learning
AI 理解论文
溯源树
样例
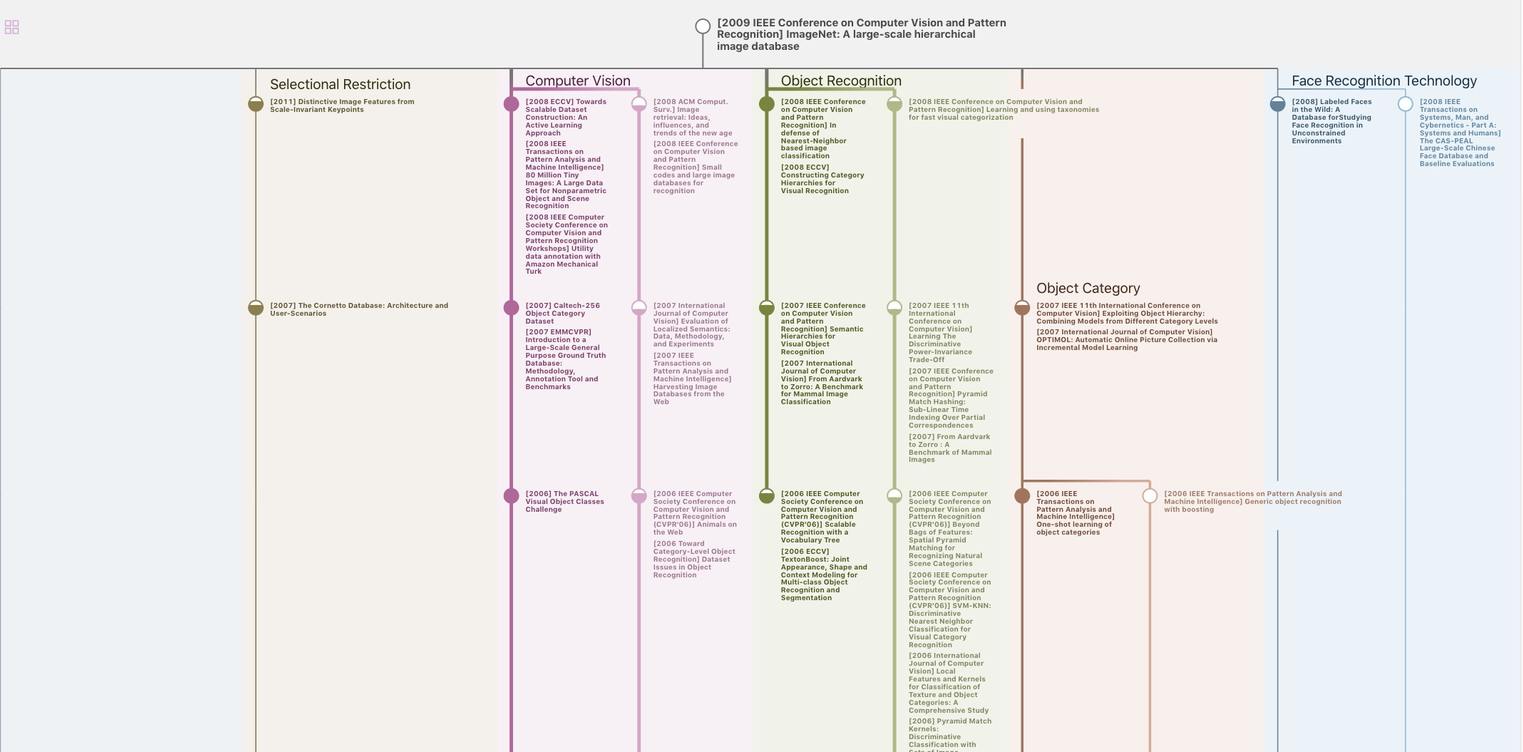
生成溯源树,研究论文发展脉络
Chat Paper
正在生成论文摘要