ReMap: Reorder Mapping for Multi-level Uneven Distribution on Sparse ReRAM Accelerator.
2023 IEEE 15th International Conference on ASIC (ASICON)(2023)
摘要
ReRAM-based accelerator has achieved excellent performance on DNN inference. However, due to its regular structure, it’s difficult to exploit its performance potential in face of data with sparsity property. Especially when compressing model through unstructured pruning, the introduced sparsity in uneven distribution is more difficult to be leveraged. Besides, in ReRAM-based sparse computation, there are also several kinds of uneven distribution in different hardware level, which result in computation parallelism reduction. In this study, we propose a novel approach to leverage weight sparsity and alleviate the multi-level uneven distribution. Through offline analysis of weight data, it’s possible to increase computation parallelism while ensuring that the upper bound of the calculation results remains within the range supported by the ADC. To further increase computation parallelism, we propose a dataflow optimization scheme by reordering the weight data in the column direction. The proposed dataflow maximizes computation parallelism with no hurt on accuracy. To apply the proposed calculation scheme, we propose a hardware architecture with little extra energy cost. Evaluation results show that the proposed optimization scheme can improve the performance by 5.2× while reducing total energy by 5.9× compared with traditional ReRAM accelerator design.
更多查看译文
关键词
ReRAM,DNN Accelerator,Weight Sparsity
AI 理解论文
溯源树
样例
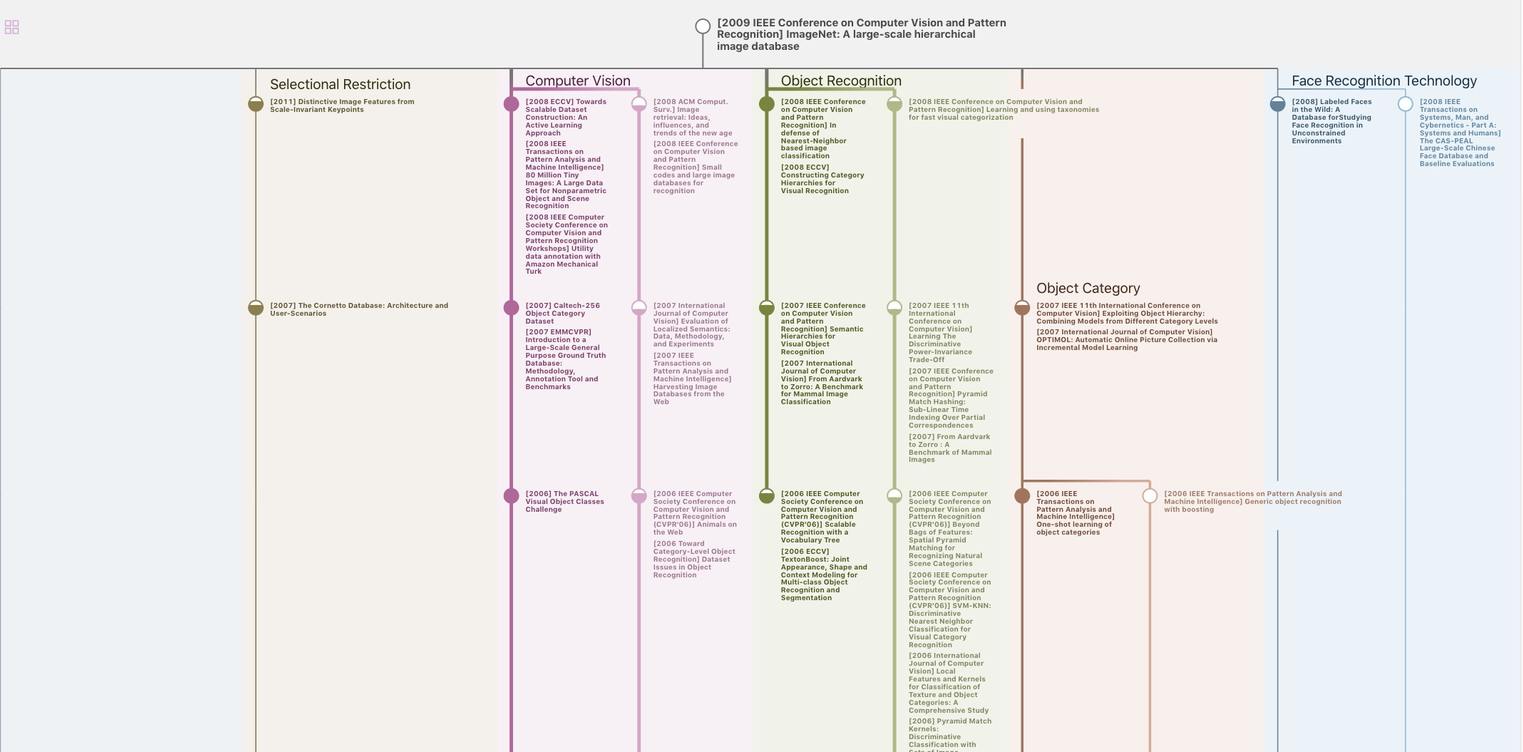
生成溯源树,研究论文发展脉络
Chat Paper
正在生成论文摘要