A Rewiring Contrastive Patch PerformerMixer Framework for Graph Representation Learning.
2023 IEEE International Conference on Big Data (BigData)(2023)
摘要
Integrating transformers with graph representation learning has emerged as a research focal point. However, recent studies showed that positional encoding in Transformers does not capture enough structural information between nodes. Additionally, existing graph neural network (GNN) models face the oversquashing issue, impeding information retention from distant nodes. To address, we transform graphs into regular structures, such as tokens, to enhance positional understanding and leverage transformer strengths. Inspired by the visual transformer (ViT) model, we propose partitioning graphs into patches and apply GNN models obtain fixed size vectors. Notably, our approach adopts contrastive learning for in-depth graph structure and incorporate more topological information via Ricci curvature to alleviate over-squashing problem by attenuating the effects of negatively curved edges while preserving the original graph structure. Unlike existing graph rewiring methods that directly modify graph structure by adding or removing edges, this approach is potentially more suitable for applications such as molecular learning where structural preservation is important. Our innovative pipeline subsequently introduces the PerformerMixer, a transformer variant with linear complexity, ensuring efficient computation. Evaluations on real-world benchmarks demonstrate our framework’s superior performance, like Peptides-func and achieve 3-WL expressiveness.
更多查看译文
关键词
Transformer,Graph representation learning,Contrastive learning
AI 理解论文
溯源树
样例
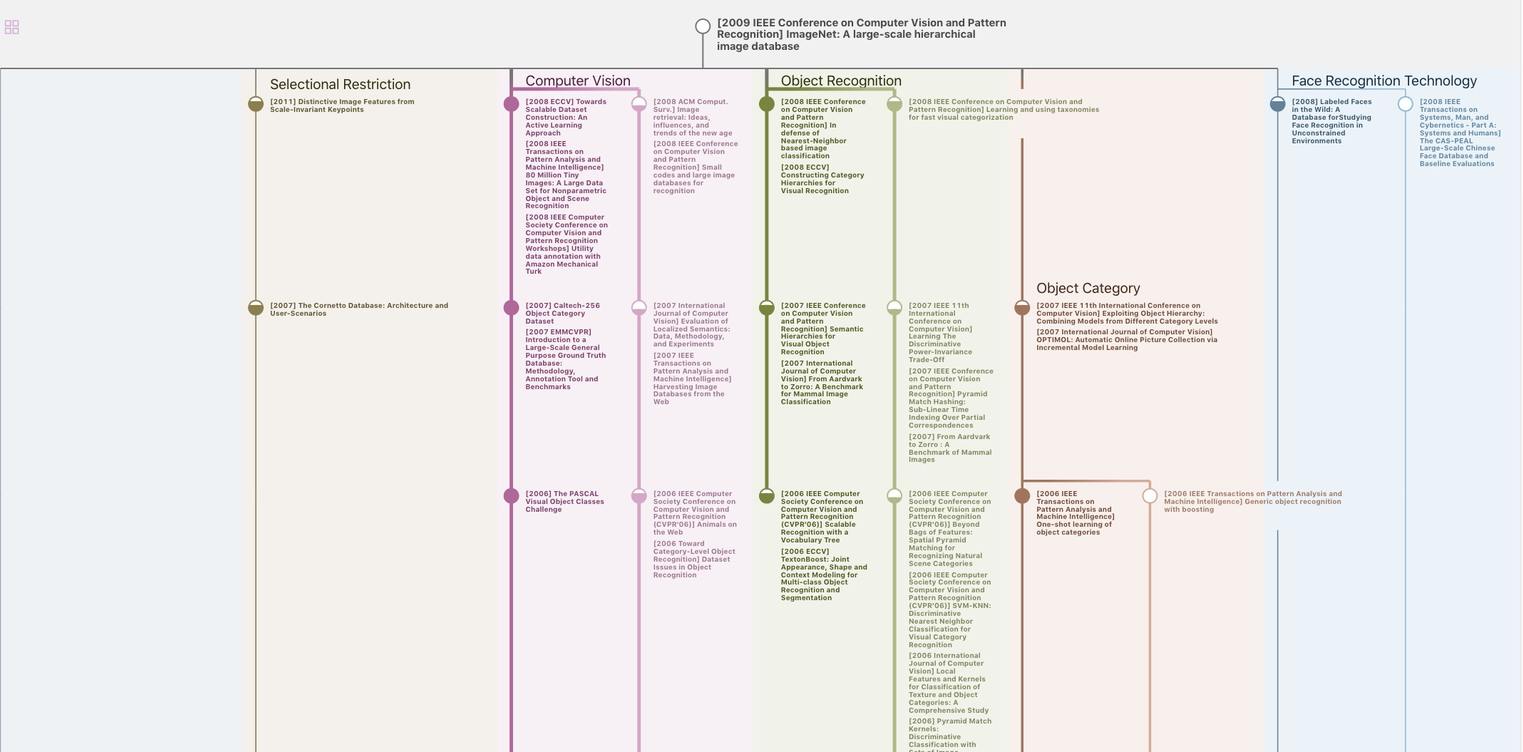
生成溯源树,研究论文发展脉络
Chat Paper
正在生成论文摘要