ANTI-Disinformation: An Adversarial Attack and Defense Network Towards Improved Robustness for Disinformation Detection on Social Media.
2023 IEEE International Conference on Big Data (BigData)(2023)
摘要
The prevalence of disinformation, which includes malformation (e.g., cyberbullying) and misinformation (e.g., fake news) in online platforms has raised significant concerns, prompting the need for robust detection methods to mitigate its detrimental impact. While the field of text classification has witnessed notable advancements in recent years, existing approaches often overlook the evolving nature of disinformation, wherein perpetrators employ perturbations to toxic content to evade detection or censorship. To address this challenge, we present a novel framework, Adversarial Network Towards Improved robustness for Disinformation detection (ANTI-Disinformation), which leverages reinforcement learning techniques as adversarial attacks. Additionally, we propose a defense model to enhance model’s robustness against such attacks. To evaluate the effectiveness of our approach, we conduct extensive experiments on well-known disinformation datasets collected from multiple social media platforms. The results demonstrate our approach can effectively produce degradation in existing models’ performance the most, showcasing the effectiveness of our framework and the vulnerability of existing detection systems. The results also exhibit that the proposed defense methods can consistently outperform existing typical methods in constructing robust detection models.
更多查看译文
关键词
Disinformation Detection,Adversarial Attack,Adversarial Defense,Model Robustness,Reinforcement Learning
AI 理解论文
溯源树
样例
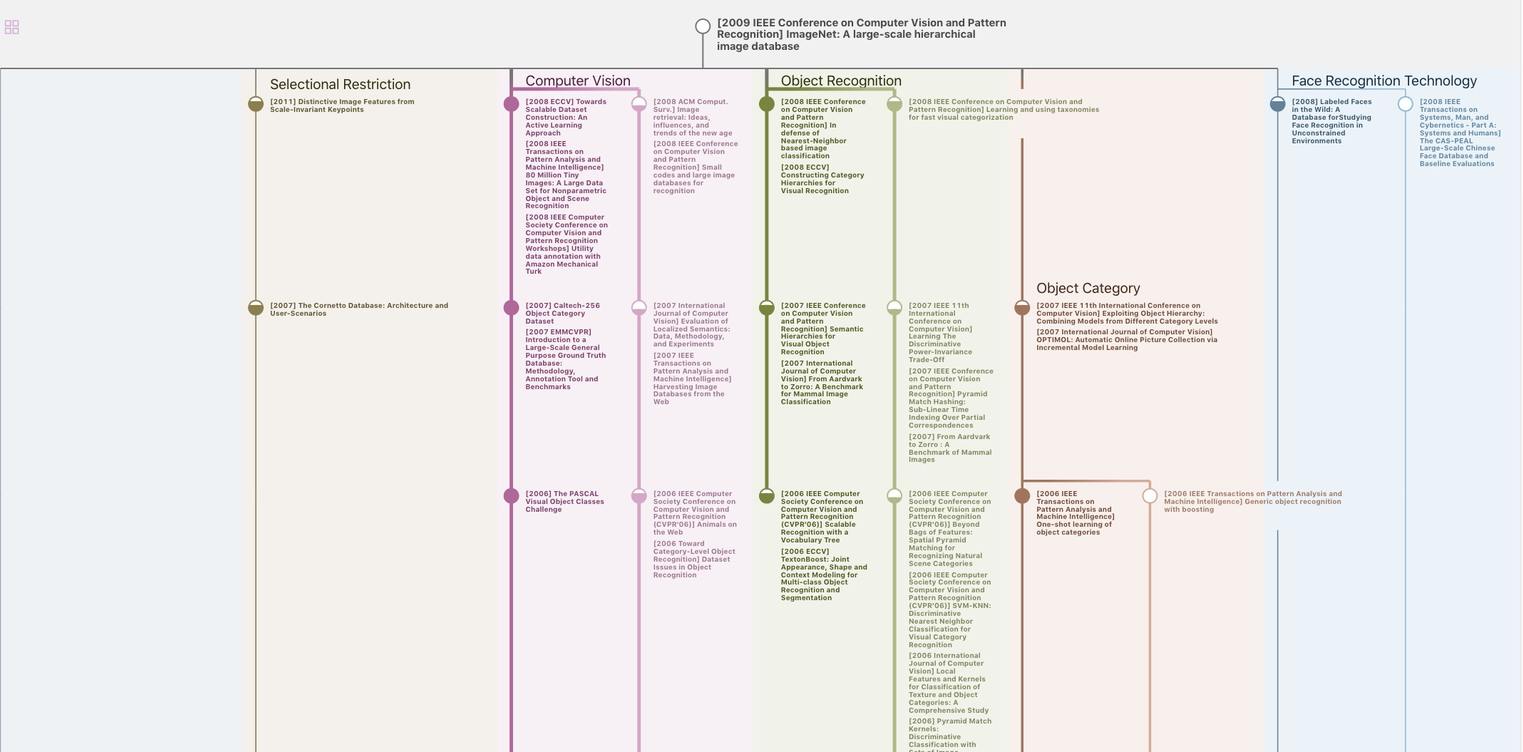
生成溯源树,研究论文发展脉络
Chat Paper
正在生成论文摘要