Stabilizing Adversarial Training for Generative Networks.
2023 IEEE International Conference on Big Data (BigData)(2023)
摘要
Generative modeling is a powerful technique that involves creating machine learning models capable of creating new data similar to the data it was trained on. Generative Adversarial Networks (GANs) are a leading approach for generative modeling. However, GAN training is known to be a notoriously difficult task. GAN convergence issues are largely caused by the supports of the real and generated distributions being disjoint. To tackle this open problem, we propose a novel GAN pre-training process that effectively aligns the supports of the generated and real data prior to applying traditional adversarial GAN training. The key component of our method, called AlignGAN, is learning a mapping between the input data distribution and a latent representation defined over a hypersphere, regularized by a One Class Classifier. This successfully encourages the generator to produce samples throughout the support of the real data, while not generating samples outside the support. We maintain support alignment through low-bandwidth noise convolutions and additional One Class regularization, leading to continued stable GAN training. We validate our approach against leading stabilization methods on three benchmark datasets, showing AlignGAN routinely produces the best results.
更多查看译文
关键词
GAN,distribution,support alignment
AI 理解论文
溯源树
样例
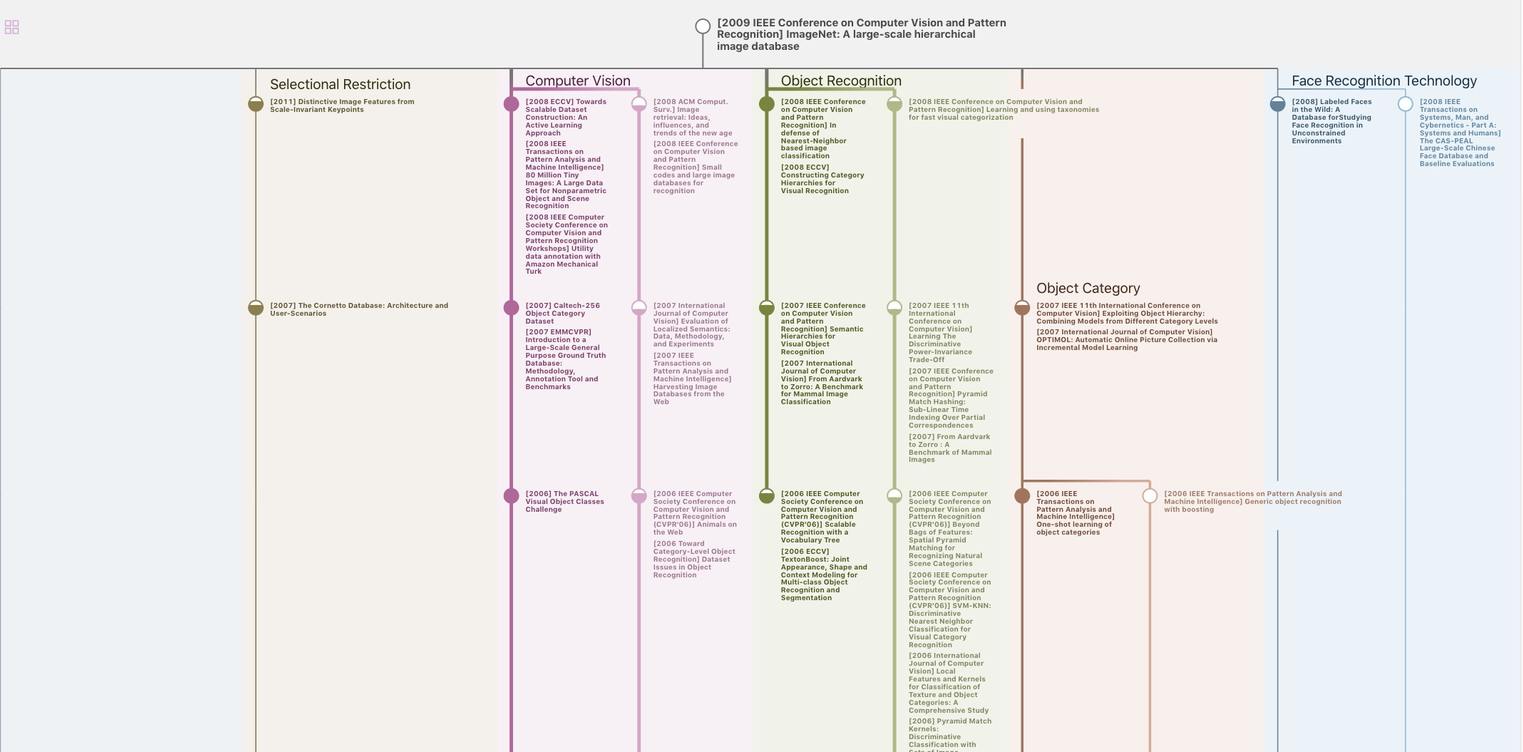
生成溯源树,研究论文发展脉络
Chat Paper
正在生成论文摘要