A Privacy-Preserving Semi-Decentralized Personalized Recommendation System.
2023 IEEE International Conference on Big Data (BigData)(2023)
摘要
In the present era of big data, recommendation systems play a crucial role in our daily lives by assisting us in making quicker and more informed decisions from a vast array of choices. The concept of personalized recommendations has gained widespread popularity, offering suggestions based on user profiles, preferences, and/or interests. Although many existing systems centralize data for making recommendations, the revelation of sensitive data poses a privacy concern, as research indicates the potential to de-identify anonymous users. For instance, sensitive information such as political views or sexual orientations can be inferred from seemingly non-sensitive data like product review and ratings. In this paper, we present a privacy-preserving personalized recommendation system named P2RecSys to address these privacy issues. Our system takes a semi-decentralized approach by treating each node in the network as an agent. Data are distributed to each agent within trusted networks, and the recommendation service provider only collects obfuscated data from agents using a differential-privacy mechanism. Consequently, data in P2RecSys are either safeguarded within local trusted networks or obfuscated outside of these networks. The final recommendation is then generated by combining local suggestions from the trusted network with obfuscated global suggestions from the service provider. The emphasis on local suggestions allows for highly personalized recommendations. Evaluation results demonstrate that P2RecSys achieves high accuracy in recommendations while effectively safeguarding user privacy.
更多查看译文
关键词
Big data,Recommendation system,Personalized recommendation,Collaborative filtering,Semi-centralized,Semi-decentralized,Privacy preservation
AI 理解论文
溯源树
样例
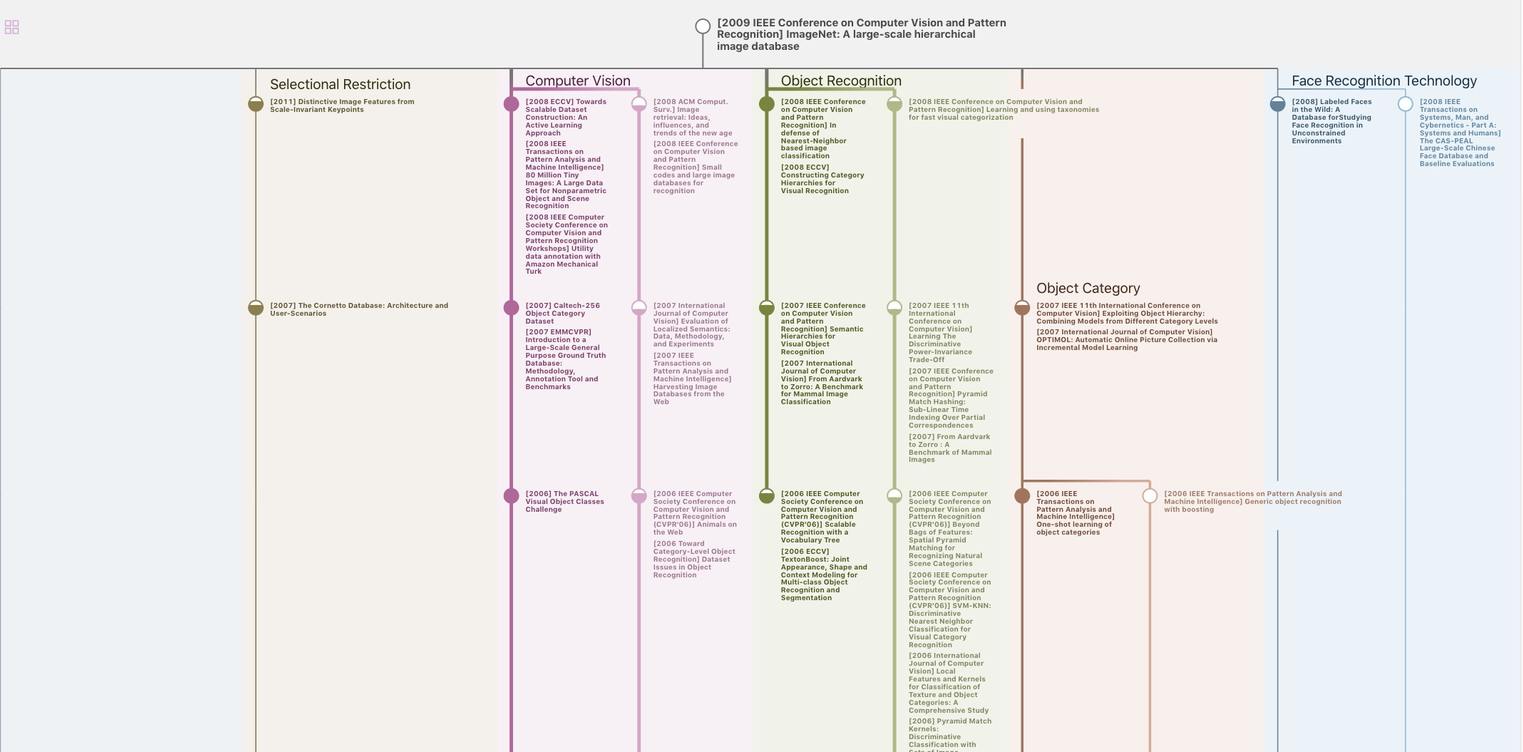
生成溯源树,研究论文发展脉络
Chat Paper
正在生成论文摘要